An Exploration Strategy Facing Non-Stationary Agents.
AAMAS(2017)
摘要
The success or failure of any learning algorithm is partially due to the exploration strategy it exerts. However, most exploration strategies assume that the environment is stationary and non-strategic. This work investigates how to design exploration strategies in non-stationary and adversarial environments. Our experimental setting uses a two agents strategic interaction scenario, where the opponent switches between different behavioral patterns. The agent's objective is to learn a model of the opponent's strategy to act optimally, despite non-determinism and stochasticity. Our contribution is twofold. First, we present drift exploration as a strategy for switch detection. Second, we propose a new algorithm called R-MAX# that reasons and acts in terms of two objectives: 1) to maximize utilities in the short term while learning and 2) eventually explore implicitly looking for opponent behavioral changes. We provide theoretical results showing that R-MAX# is guaranteed to detect the opponent's switch and learn a new model in terms of finite sample complexity.
更多查看译文
关键词
Exploration,non-stationary environments,repeated games
AI 理解论文
溯源树
样例
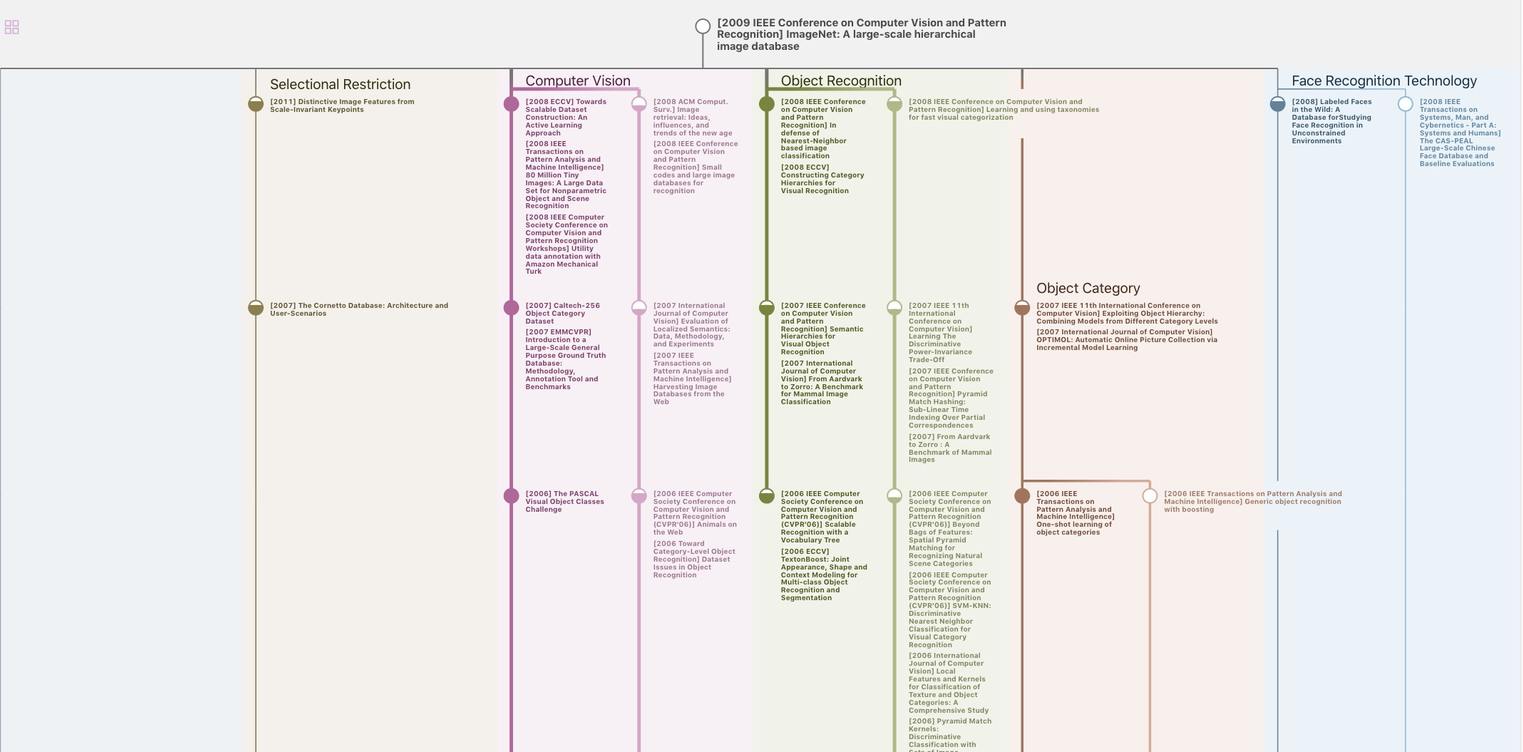
生成溯源树,研究论文发展脉络
Chat Paper
正在生成论文摘要