FF-CNN: An efficient deep neural network for mitosis detection in breast cancer histological images
Communications in Computer and Information Science(2017)
摘要
We utilized deep fused fully convolutional neural network (FF-CNN) based on the knowledge transferred from pre-trained AlexNet model to detect mitoses in hematoxylin and eosin stained histology image of breast cancer. Currently, existing mitosis counting methods are based on either the handcrafted features including morphology, color and texture or the abstract features learning from deep neural network. The handcrafted features are pertinent, corresponding to what learned from lower layers of deep convolutional neural network (CNN), on the other hand, features extracted from higher layers are comprehensive. Both handcrafted and extracted features are significant to mitosis detection. More importantly, this detection suffers from class-imbalance and the inconsistent staining color of H&E images. This paper proposed a modified fully convolutional neural network (FCN) structure combining the rich features from different level layers together and a multi-step fine-tuning strategy to reduce over-fitting. In order to treat class-imbalance, we utilized a cascaded approach to select the most confusing non-mitosis samples which made the training efficiently, and weighted the loss function by the corresponding class frequency. For the inconsistent staining appearance issue, we applied the method of stain-normalization to the augment training samples to improve generalization ability of model and to pre-process testing images to obtain more accurate results. As preliminarily validated on the public 2014 ICPR MITOSIS data, our method achieves a better performance in term of detection accuracy than ever recorded for this dataset with an acceptable detection speed. © Springer International Publishing AG 2017.
更多查看译文
关键词
Breast cancer,Class-imbalance,CNN,Mitosis detection,Stain-normalization,Training samples
AI 理解论文
溯源树
样例
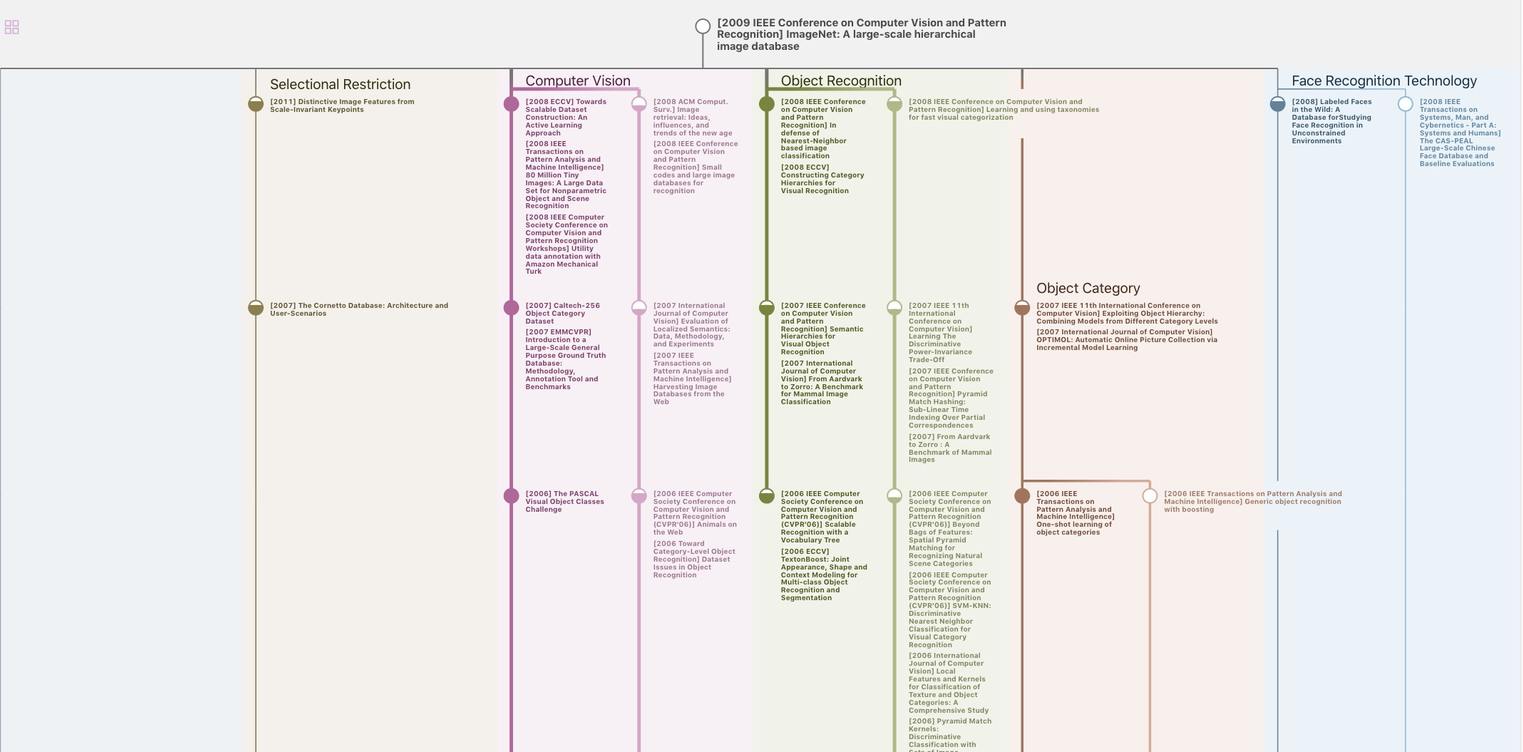
生成溯源树,研究论文发展脉络
Chat Paper
正在生成论文摘要