Multi-Task Learning For Parsing The Alexa Meaning Representation Language.
THIRTY-SECOND AAAI CONFERENCE ON ARTIFICIAL INTELLIGENCE / THIRTIETH INNOVATIVE APPLICATIONS OF ARTIFICIAL INTELLIGENCE CONFERENCE / EIGHTH AAAI SYMPOSIUM ON EDUCATIONAL ADVANCES IN ARTIFICIAL INTELLIGENCE(2018)
摘要
The Alexa Meaning Representation Language (AMRL) is a compositional graph-based semantic representation that includes-fine-grained types, properties, actions, and roles and can represent a wide variety of spoken language. AMRL increases the ability of virtual assistants to represent more complex requests, including logical and conditional statements as well as ones with nested clauses. Due to this representational capacity, the acquisition of large scale data resources is challenging, which limits the accuracy of resulting models. This paper has two primary contributions. The first contribution is a linearization of the AMRL parses that aligns it to a related task of spoken language understanding (SLU) and a deep neural network architecture that uses multi-task learning to predict AMRL fine-grained types, properties and intents. The second contribution is a deep neural network architecture that leverages embeddings from the large-scale data resources that are available for SLID. When combined, these contributions enable the training of accurate models of AMRL parsing, even in the presence of data sparsity. The proposed models, which use the linearized AMRL parse, multi-task learning, residual connections and embeddings from SLU, decrease the error rates in the prediction of the full AMRL parse by 3.56% absolute.
更多查看译文
AI 理解论文
溯源树
样例
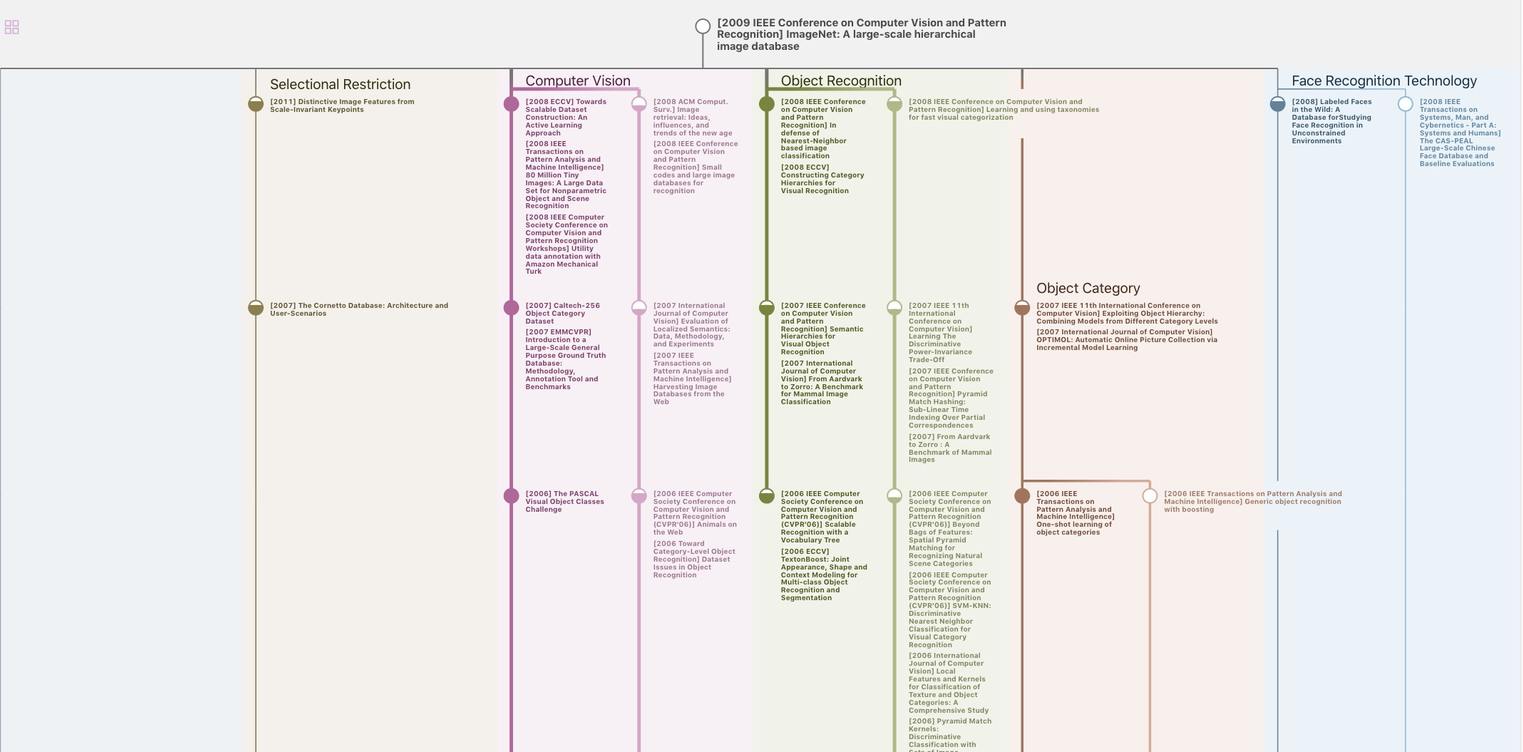
生成溯源树,研究论文发展脉络
Chat Paper
正在生成论文摘要