Chiral cliffs: Investigating the influence of chirality on binding affinity.
CHEMMEDCHEM(2018)
摘要
Chirality is understood by many as a binary concept: a molecule is either chiral or it is not. In terms of the action of a structure on polarized light, this is indeed true. When examined through the prism of molecular recognition, the answer becomes more nuanced. In this work, we investigated chiral behavior on protein-ligand binding: when does chirality make a difference in binding activity? Chirality is a property of the 3D structure, so recognition also requires an appreciation of the conformation. In many situations, the bioactive conformation is undefined. We set out to address this by defining and using several novel 2D descriptors to capture general characteristic features of the chiral center. Using machine-learning methods, we built different predictive models to estimate if a chiral pair (a set of two enantiomers) might exhibit a chiral cliff in a binding assay. A set of about 3800 chiral pairs extracted from the ChEMBL23 database was used to train and test our models. By achieving an accuracy of up to 75%, our models provide good performance in discriminating chiral cliffs from non-cliffs. More importantly, we were able to derive some simple guidelines for when one can reasonably use a racemate and when an enantiopure compound is needed in an assay. We critically discuss our results and show detailed examples of using our guidelines. Along with this publication we provide our dataset, our novel descriptors, and the Python code to rebuild the predictive models.
更多查看译文
关键词
2D descriptors,activity cliffs,chirality,feature selection,machine learning
AI 理解论文
溯源树
样例
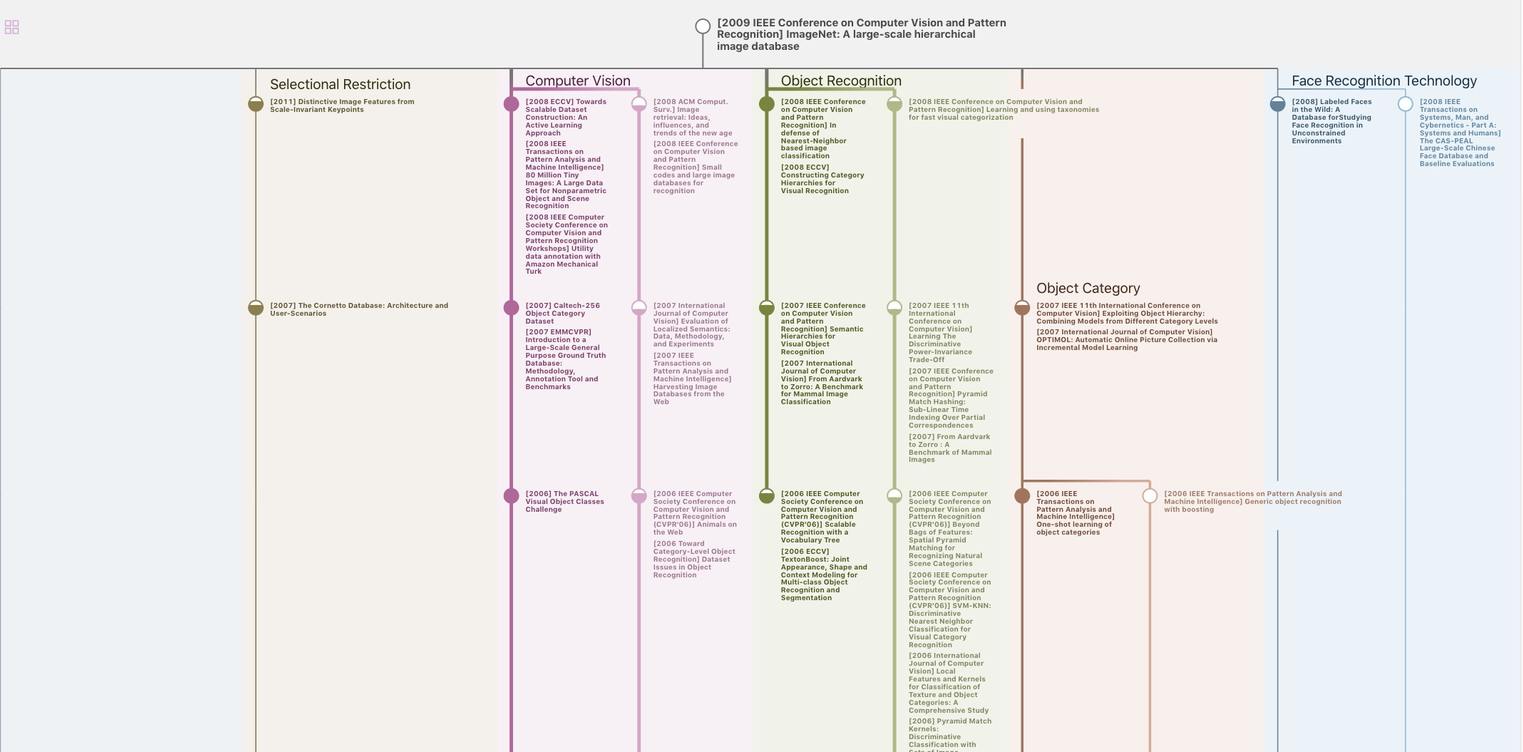
生成溯源树,研究论文发展脉络
Chat Paper
正在生成论文摘要