A Comparison of LSTMs and Attention Mechanisms for Forecasting Financial Time Series.
arXiv: Learning(2018)
摘要
While LSTMs show increasingly promising results for forecasting Financial Time Series (FTS), this paper seeks to assess if attention mechanisms can further improve performance. The hypothesis is that attention can help prevent long-term dependencies experienced by LSTM models. To test this hypothesis, the main contribution of this paper is the implementation of an LSTM with attention. Both the benchmark LSTM and the LSTM with attention were compared and both achieved reasonable performances of up to 60% on five stocks from Kaggleu0027s Two Sigma dataset. This comparative analysis demonstrates that an LSTM with attention can indeed outperform standalone LSTMs but further investigation is required as issues do arise with such model architectures.
更多查看译文
AI 理解论文
溯源树
样例
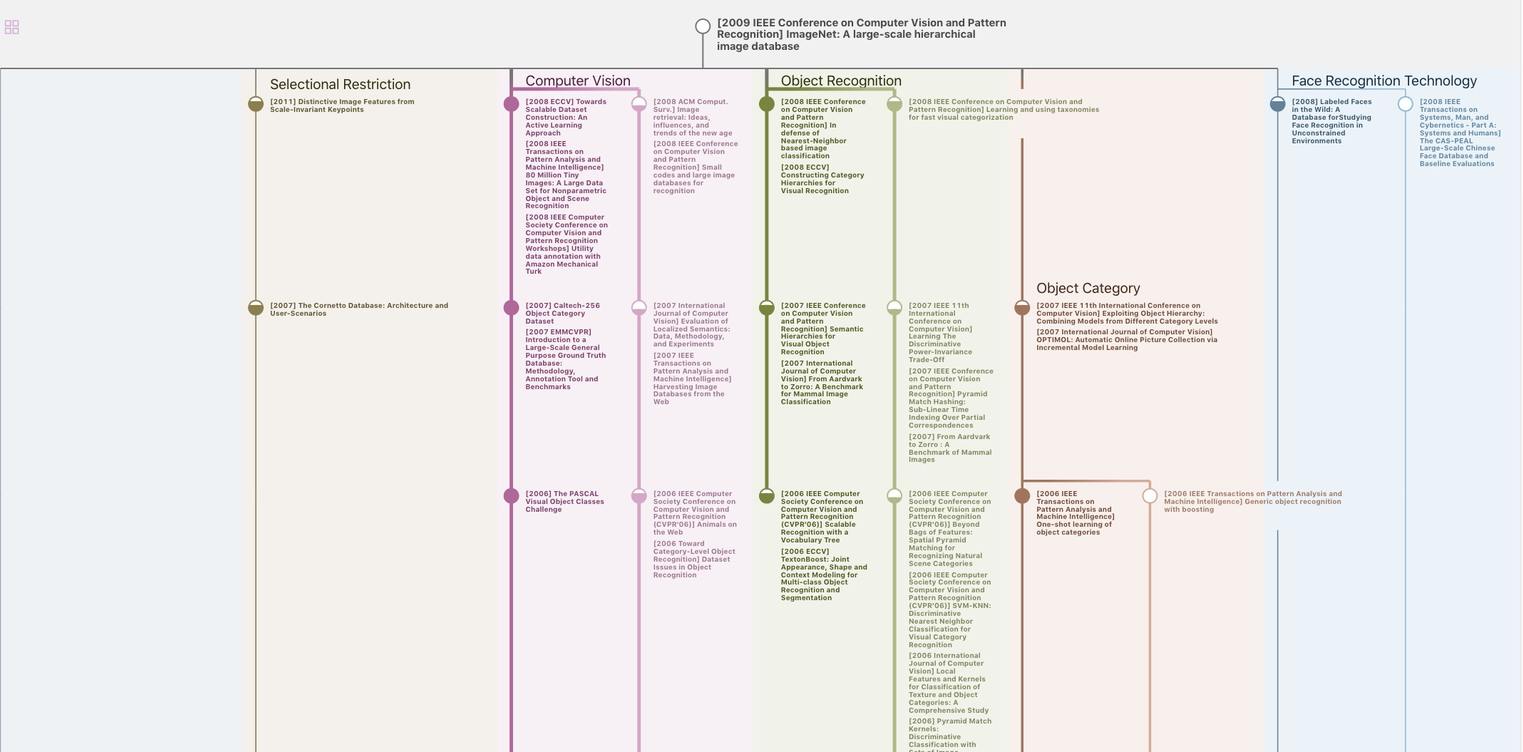
生成溯源树,研究论文发展脉络
Chat Paper
正在生成论文摘要