A probabilistic model of redundancy in information extraction
IJCAI(2005)
摘要
Unsupervised Information Extraction (UIE) is the task of extracting knowledge from text without using hand-tagged training examples. A fundamental problem for both UIE and supervised IE is assessing the probability that extracted information is correct. In massive corpora such as the Web, the same extraction is found repeatedly in different documents. How does this redundancy impact the probability of correctness? This paper introduces a combinatorial "balls-andurns" model that computes the impact of sample size, redundancy, and corroboration from multiple distinct extraction rules on the probability that an extraction is correct. We describe methods for estimating the model's parameters in practice and demonstrate experimentally that for UIE the model's log likelihoods are 15 times better, on average, than those obtained by Pointwise Mutual Information (PMI) and the noisy-or model used in previous work. For supervised IE, the model's performance is comparable to that of Support Vector Machines, and Logistic Regression.
更多查看译文
关键词
support vector machines,redundancy impact,probabilistic model,noisy-or model,logistic regression,supervised ie,fundamental problem,unsupervised information extraction,pointwise mutual information,multiple distinct extraction rule,different document,information extraction,redundancy,sample size,rule based systems,information retrieval,support vector machine,internet,regression analysis
AI 理解论文
溯源树
样例
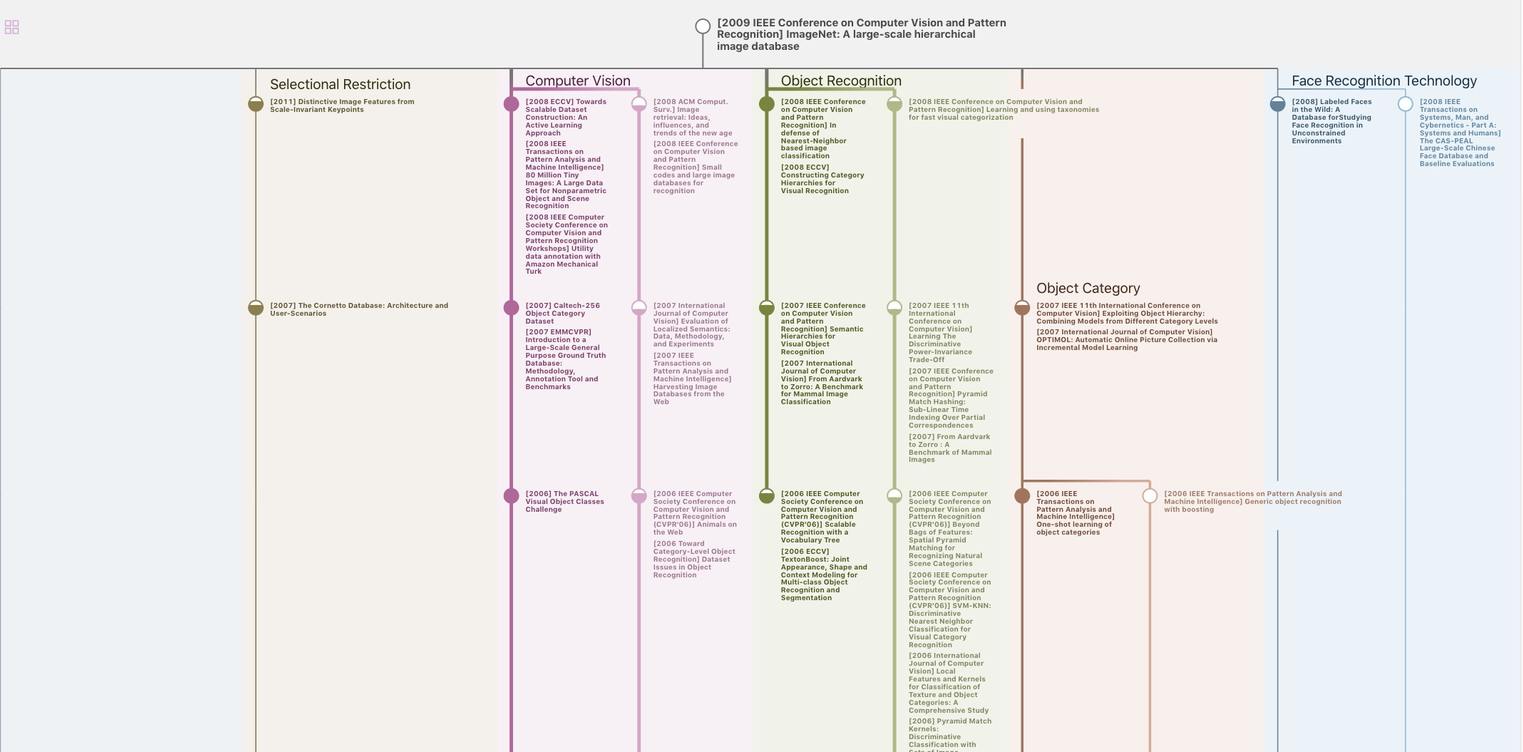
生成溯源树,研究论文发展脉络
Chat Paper
正在生成论文摘要