Hype: A Benchmark For Human Eye Perceptual Evaluation Of Generative Models
ADVANCES IN NEURAL INFORMATION PROCESSING SYSTEMS 32 (NIPS 2019)(2019)
摘要
Generative models often use human evaluations to measure the perceived quality of their outputs. Automated metrics are noisy indirect proxies, because they rely on heuristics or pretrained embeddings. However, up until now, direct human evaluation strategies have been ad-hoc, neither standardized nor validated. Our work establishes a gold standard human benchmark for generative realism. We construct HUMAN EYE PERCEPTUAL EVALUATION (HYPE), a human benchmark that is (1) grounded in psychophysics research in perception, (2) reliable across different sets of randomly sampled outputs from a model, (3) able to produce separable model performances, and (4) efficient in cost and time. We introduce two variants: one that measures visual perception under adaptive time constraints to determine the threshold at which a model's outputs appear real (e.g. 250ms), and the other a less expensive variant that measures human error rate on fake and real images sans time constraints. We test HYPE across six state-of-the-art generative adversarial networks and two sampling techniques on conditional and unconditional image generation using four datasets: CelebA, FFHQ, CIFAR-10, and ImageNet. We find that HYPE can track the relative improvements between models, and we confirm via bootstrap sampling that these measurements are consistent and replicable.[GRAPHICS].
更多查看译文
关键词
perceived quality,bootstrap sampling,visual perception
AI 理解论文
溯源树
样例
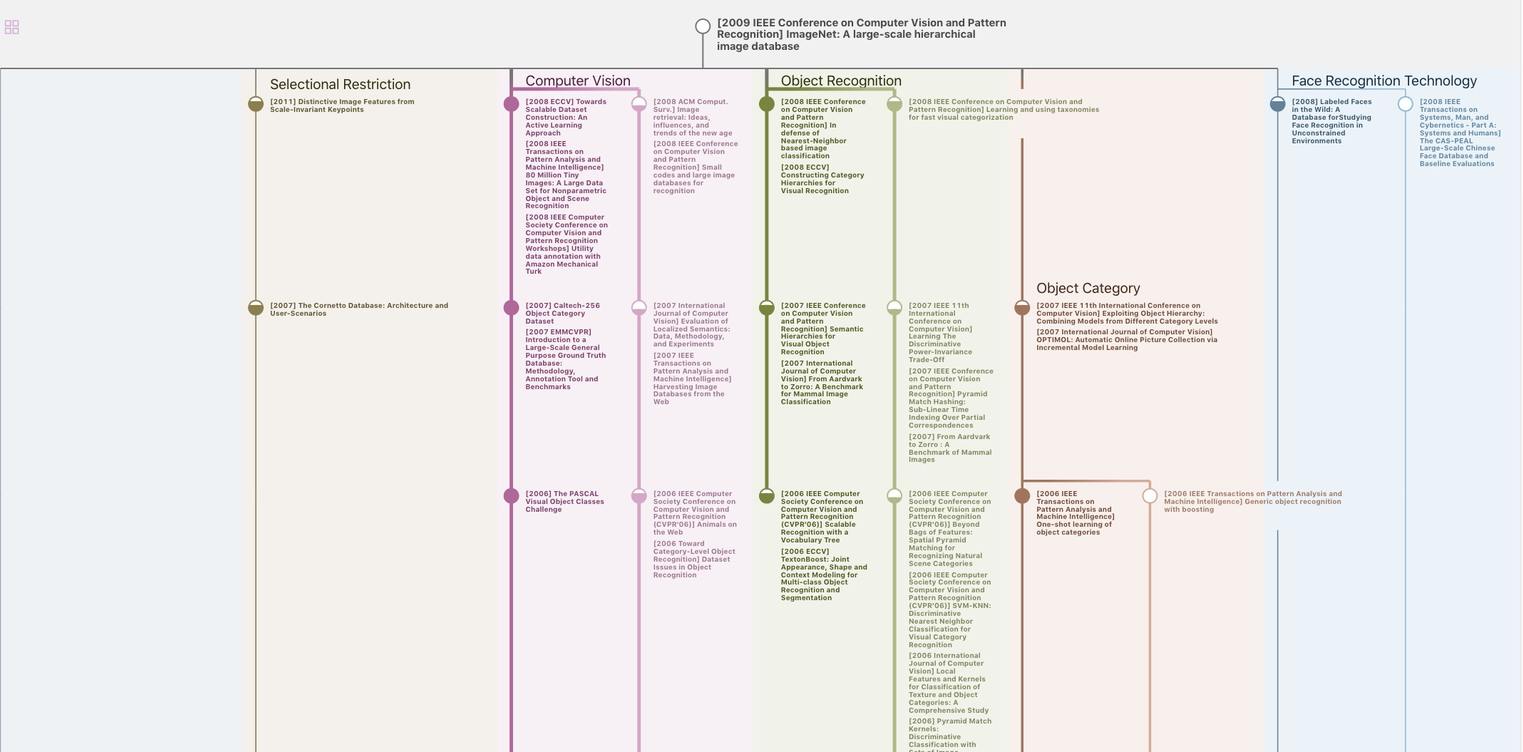
生成溯源树,研究论文发展脉络
Chat Paper
正在生成论文摘要