Beyond Gradient Descent For Regularized Segmentation Losses
2019 IEEE/CVF CONFERENCE ON COMPUTER VISION AND PATTERN RECOGNITION (CVPR 2019)(2019)
Abstract
The simplicity of gradient descent (GD) made it the default method for training ever-deeper and complex neural networks. Both loss functions and architectures are often explicitly tuned to be amenable to this basic local optimization. In the context of weakly-supervised CNN segmentation, we demonstrate a well-motivated loss function where an alternative optimizer (ADM)(1) achieves the state-of-the-art while GD performs poorly. Interestingly, GD obtains its best result for a "smoother" tuning of the loss function. The results are consistent across different network architectures. Our loss is motivated by well-understood MRF/CRF regularization models in "shallow" segmentation and their known global solvers. Our work suggests that network design/training should pay more attention to optimization methods.
MoreTranslated text
Key words
Optimization Methods,Deep Learning,Scene Analysis and Understanding,Segmentation,Grouping and Shape
AI Read Science
Must-Reading Tree
Example
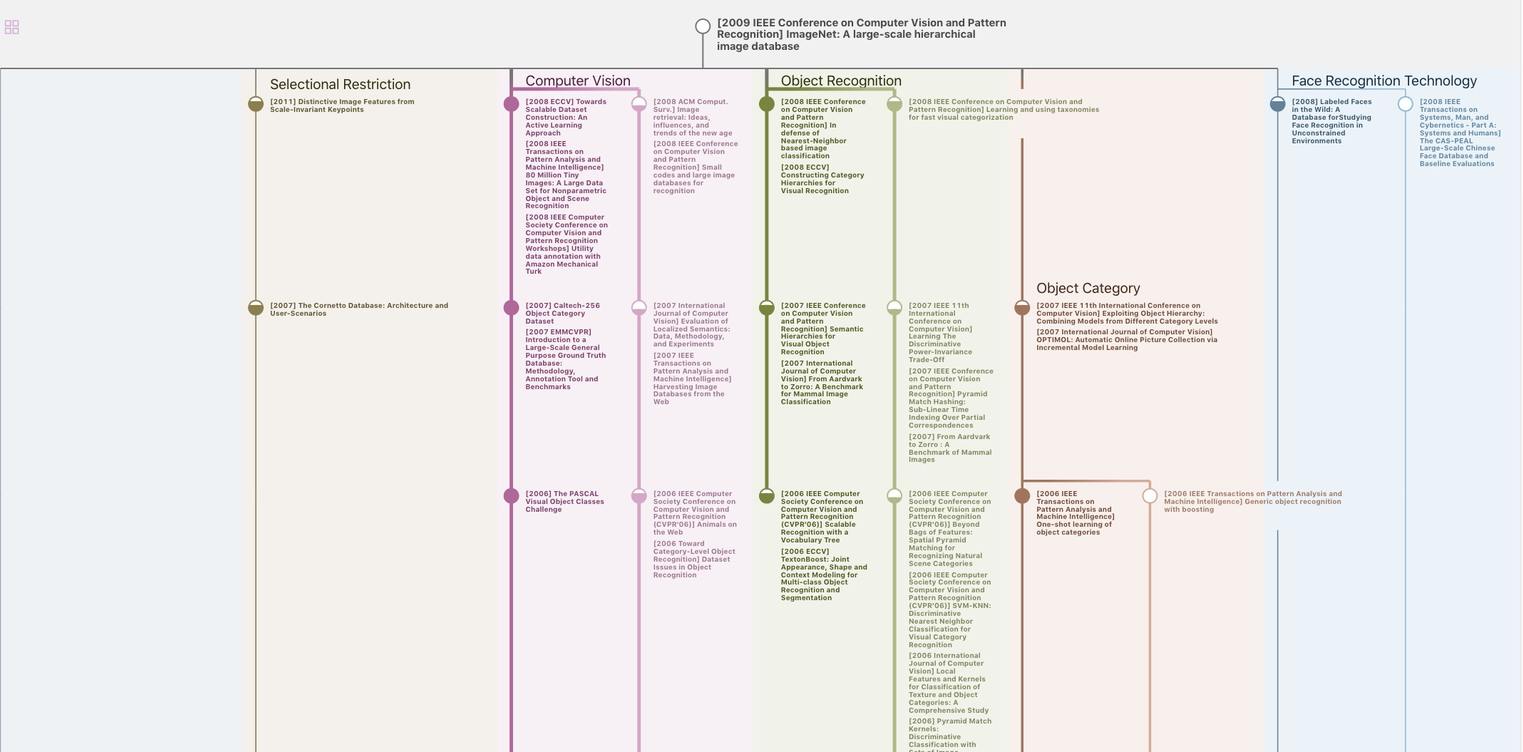
Generate MRT to find the research sequence of this paper
Chat Paper
Summary is being generated by the instructions you defined