Visual Relationships As Functions: Enabling Few-Shot Scene Graph Prediction
2019 IEEE/CVF INTERNATIONAL CONFERENCE ON COMPUTER VISION WORKSHOPS (ICCVW)(2019)
摘要
Scene graph prediction - classifying of objects and predicates in a visual scene - requires substantial training data. The long-tailed distribution of relationships can he an obstacle for such approaches, however; as they can only be trained on the small set of predicates that carry sufficient labels. We introduce the first scene graph prediction model that supports few-shot learning of predicates, enabling scene graph approaches to generalize to a set of new predicates. First, we introduce a new model of predicates as that operate on object features or image locations. Next, we define a scene graph model where these functions are trained as message passing protocols within a new graph convolution framework. We train the framework with a frequently occurring set of predicates and show that our approach outperforms those that use the same amount of supervision by 1.78 at recall@50 and performs on par with other scene graph models. Next, we extract object representations generated by the trained predicate functions to train few-shot predicate classifiers on rare predicates with as fern as 1 labeled example. When compared to strong baselines like transfer learning from existing state-of-the-art representations, we show improved 5-shot performance by 4.16 recall@1. Finally, we show that our predicate functions generate interpretable visual, enabling, the first interpretable scene graph model.
更多查看译文
关键词
computer vision,scene graphs,few shot,graph convolution networks,interpretability,image understanding
AI 理解论文
溯源树
样例
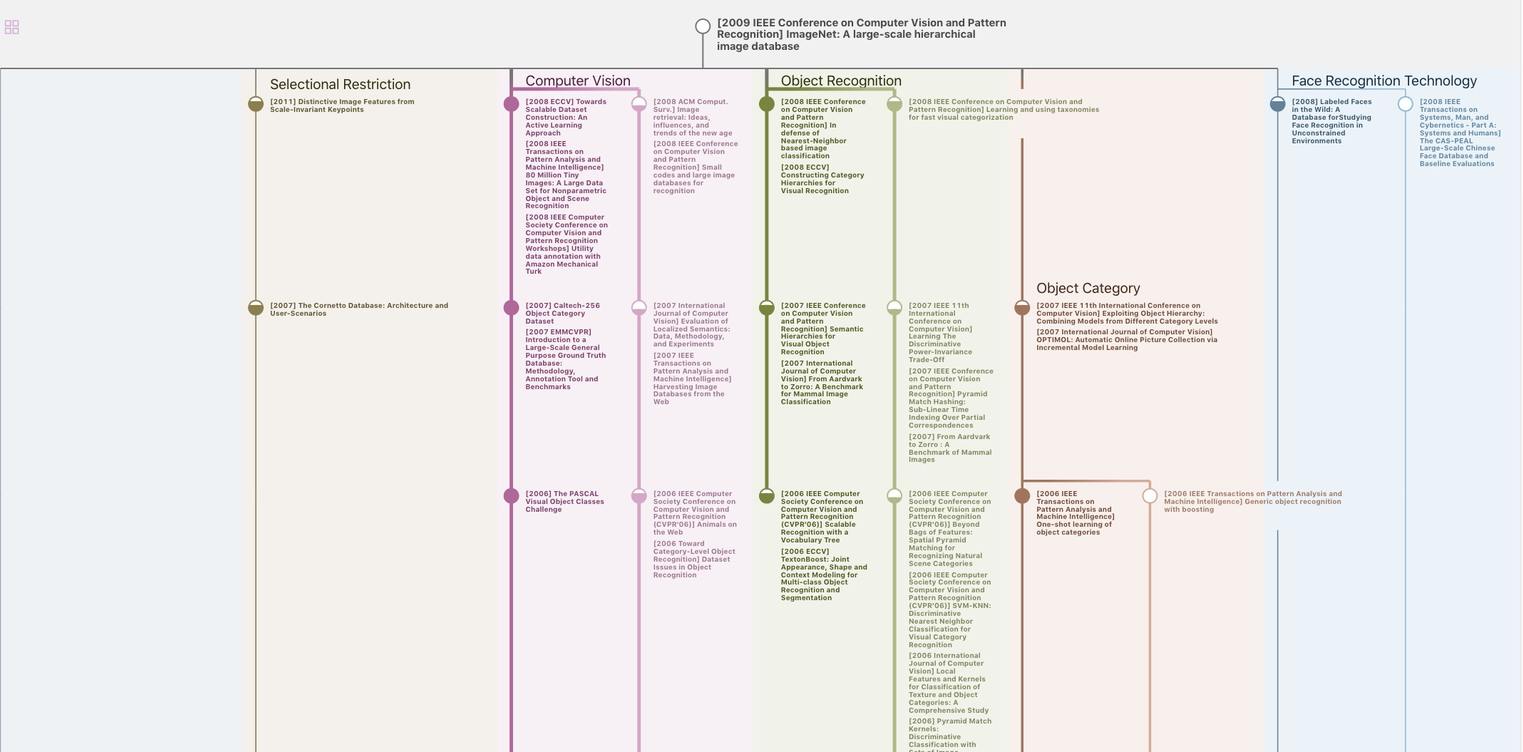
生成溯源树,研究论文发展脉络
Chat Paper
正在生成论文摘要