A multi-encoder neural conversation model.
Neurocomputing(2019)
摘要
With the development of deep neural networks, Sequence-to-sequence (Seq2Seq) models become a popular technique of conversation models. Current Seq2Seq models with single encoder-decoder structures tend to generate responses which contain high frequency patterns on datasets. However, these patterns are always generic and meaningless. Generic and meaningless responses will lead the conversation between computer and human to an end quickly. According to our observations, human conversations are always topic related. If the conversation data can be divided into different clusters according to their topics, high frequency patterns will be topic related rather than generic. We consider that a model trained in different clusters can generate more topic related and meaningful responses. Inspired by this idea, we propose a Multi-Encoder Neural Conversation (MENC) model. MENC can make use of topic information by its multi-encoder structure. To the best of our knowledge, it is the first work which applies multi-encoder structures into conversation models. We conduct our experiments on two daily conversation datasets. Our experiments show that MENC gets a better performance than other mainstream models on both subject and object evaluation metrics.
更多查看译文
关键词
Multi-encoder,Conversation,Sequence-to-sequence models
AI 理解论文
溯源树
样例
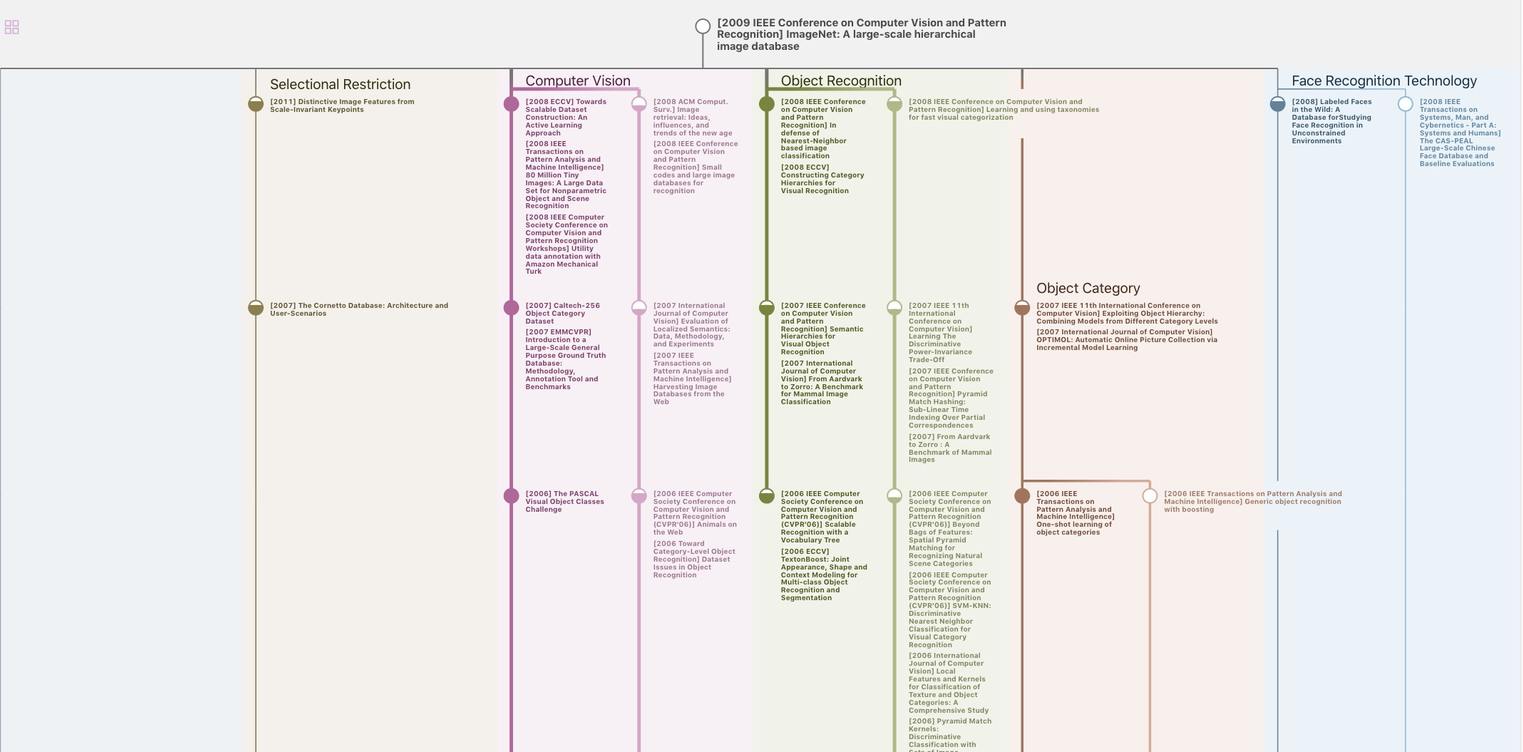
生成溯源树,研究论文发展脉络
Chat Paper
正在生成论文摘要