Detecting and Diagnosing Adversarial Images with Class-Conditional Capsule Reconstructions
ICLR(2020)
摘要
Adversarial examples raise questions about whether neural network models are sensitive to the same visual features as humans. In this paper, we first detect adversarial examples or otherwise corrupted images based on a class-conditional reconstruction of the input. To specifically attack our detection mechanism, we propose the Reconstructive Attack which seeks both to cause a misclassification and a low reconstruction error. This reconstructive attack produces undetected adversarial examples but with much smaller success rate. Among all these attacks, we find that CapsNets always perform better than convolutional networks. Then, we diagnose the adversarial examples for CapsNets and find that the success of the reconstructive attack is highly related to the visual similarity between the source and target class. Additionally, the resulting perturbations can cause the input image to appear visually more like the target class and hence become non-adversarial. This suggests that CapsNets use features that are more aligned with human perception and have the potential to address the central issue raised by adversarial examples.
更多查看译文
关键词
Adversarial Examples, Detection of adversarial attacks
AI 理解论文
溯源树
样例
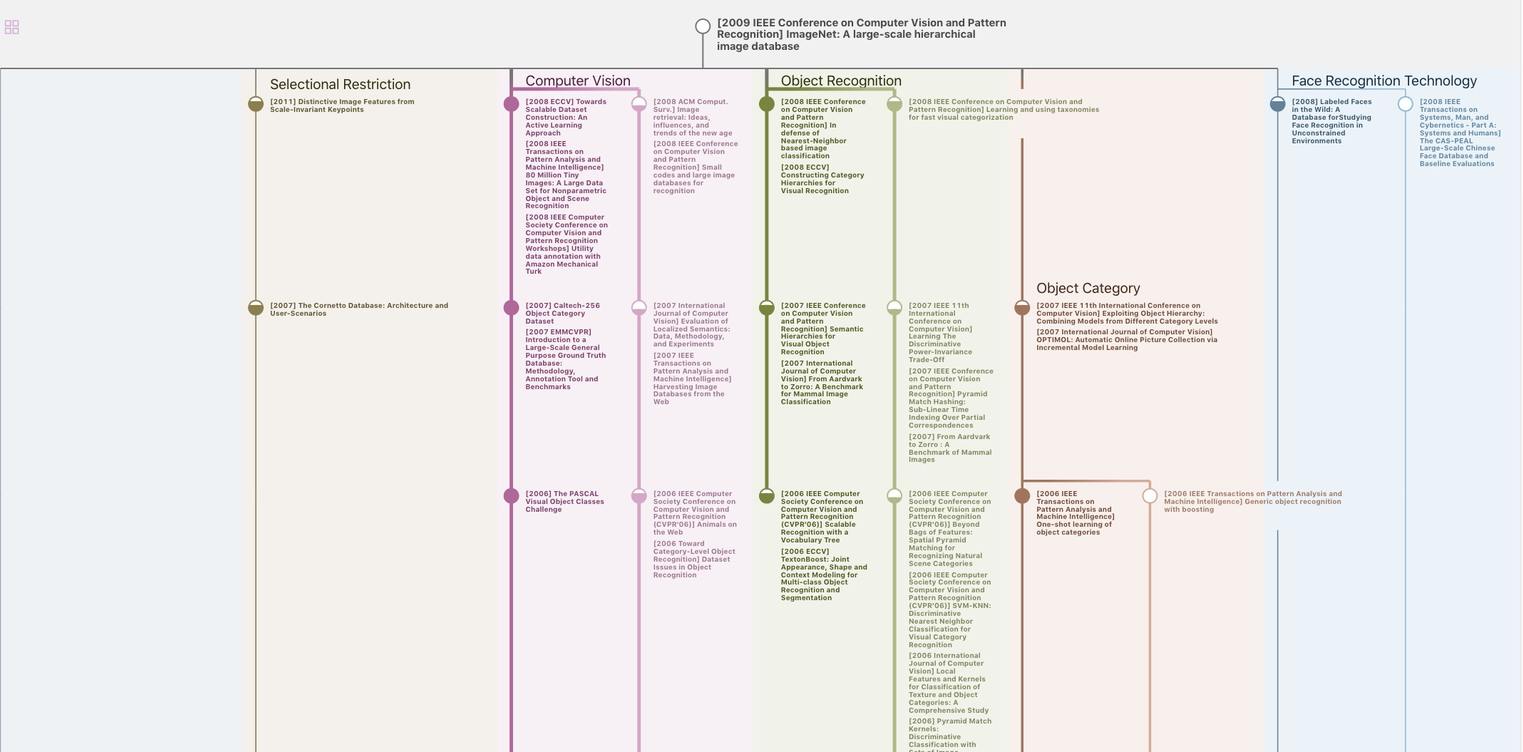
生成溯源树,研究论文发展脉络
Chat Paper
正在生成论文摘要