Approximate Relative Value Learning for Average-reward Continuous State MDPs
UAI(2020)
摘要
In this paper, we propose an approximate relative value learning (ARVL) algorithm for nonparametric MDPs with continuous state space and finite actions and average reward criterion. It is a sampling based algorithm combined with kernel density estimation and function approximation via nearest neighbors. The theoretical analysis is done via a random contraction operator framework and stochastic dominance argument. This is the first such algorithm for continuous state space MDPs with average reward criteria with these provable properties which does not require any discretization of state space as far as we know. We then evaluate the proposed algorithm on a benchmark problem numerically.
更多查看译文
关键词
approximate relative value learning,average-reward
AI 理解论文
溯源树
样例
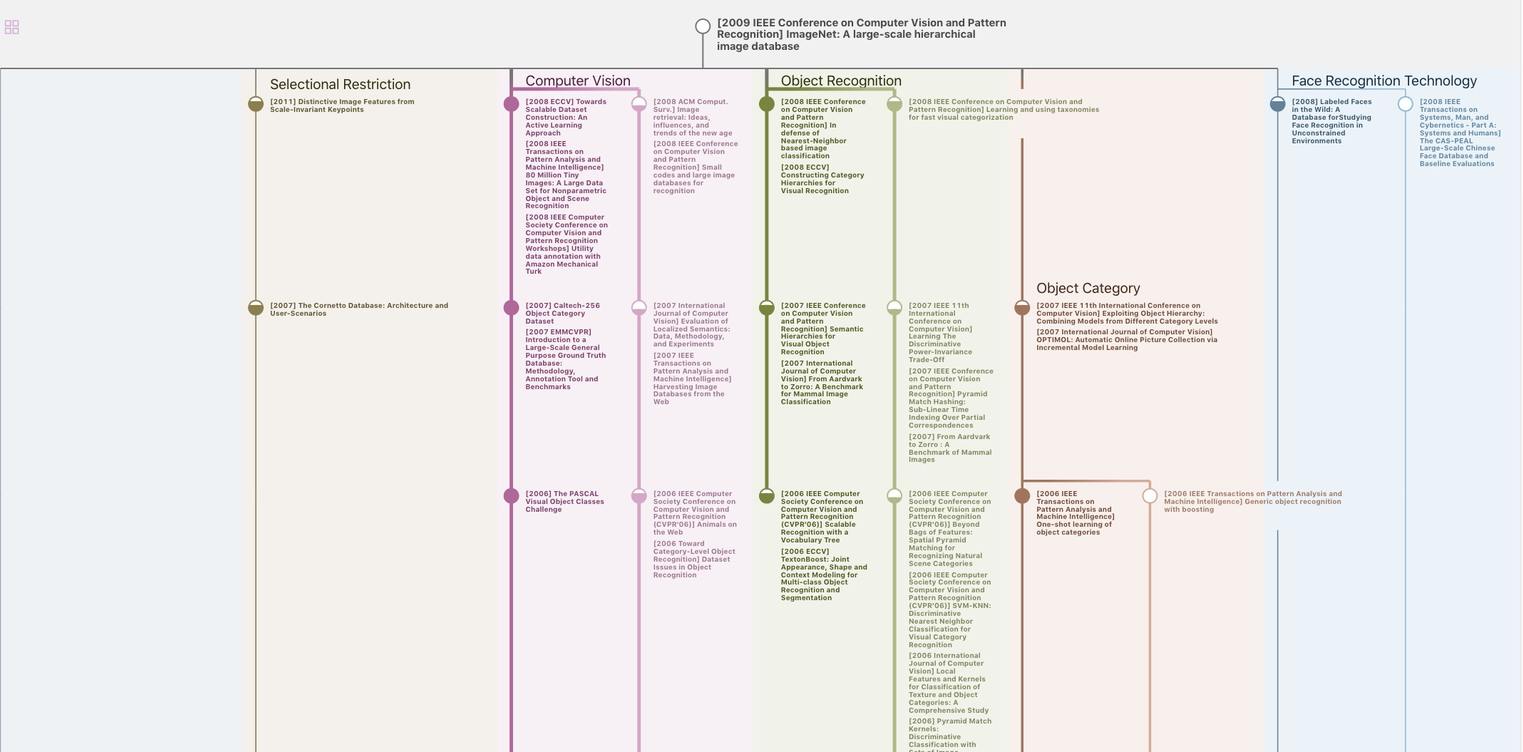
生成溯源树,研究论文发展脉络
Chat Paper
正在生成论文摘要