An Automatic Sparse Model Estimation Method Guided by Constraints That Encode System Properties
2019 18TH EUROPEAN CONTROL CONFERENCE (ECC)(2019)
摘要
Finding efficient methods to estimate model parameters or build models from time-series data is a central quest in Systems and Synthetic Biology. To aid this search, existing parameter estimation methods were adapted from other fields, or new ones were developed. In this paper, we use the Sparse Bayesian Learning (SBL) framework, which was first developed in the field of signal processing, and implement it as an iterative convex optimization problem. We extend the existing SBL framework to accommodate constraints that enforce certain system properties, such as nonnegative state variables or bounded state trajectories. These properties are vital parts of a dynamical model in biology and chemistry but are often overlooked in the parameter estimation literature. As a result of this work, the extended framework can automatically build “proper” dynamical models from time-series data. Finally, the examples show that such framework complemented with appropriate constraints can aid the model building process.
更多查看译文
关键词
iterative convex optimization problem,nonnegative state variables,bounded state trajectories,dynamical model,time-series data,model building process,parameter estimation methods,sparse Bayesian learning framework,SBL framework,dynamical models,model parameter estimation,Systems and Synthetic Biology,system properties encoding,automatic sparse model estimation method
AI 理解论文
溯源树
样例
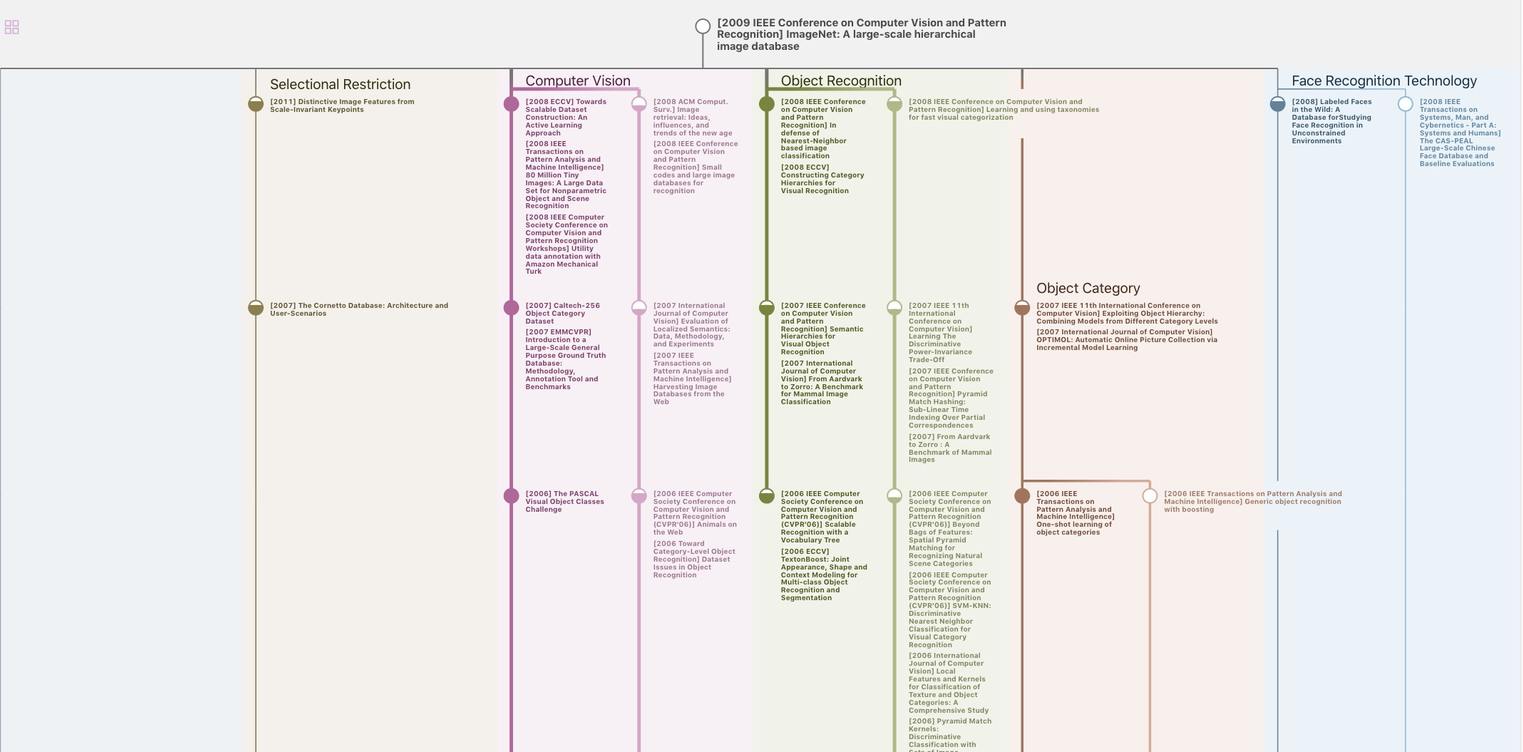
生成溯源树,研究论文发展脉络
Chat Paper
正在生成论文摘要