Recognizing Global Reservoirs from Landsat 8 Images: A Deep Learning Approach
IEEE journal of selected topics in applied earth observations and remote sensing(2019)
摘要
Man-made reservoirs are key components of terrestrial hydrological systems. Identifying the location and number of reservoirs is the premise for studying the impact of human activities on water resources and environmental changes. While complete bottom-up censuses can provide a comprehensive view of the reservoir landscape, they are time-consuming and laborious and are thus infeasible on a global scale. Moreover, it is challenging to distinguish man-made reservoirs from natural lakes in remote sensing images. This study proposes a convolutional neural network (CNN)-based framework to recognize global reservoirs from Landsat 8 imageries. On the basis of the HydroLAKES dataset, a Landsat 8 cloud-free mosaic of 2017 was clipped for each feature (reservoir or lake) and was resized into 224 x 224 patches, which were collected as training and testing samples. Compared to other deep learning methods (Alexnet and VGG) and state-of-the-art traditional machine learning methods (support vector machine, random forest, gradient boosting, and bag-of-visual-words), we found that fine-tuning the pretrainedCNNmodel, ResNet-50, could reach the highest accuracy (91.45%). Application cases in Kansas (USA, North America), Mpumalanga (South Africa, Africa), and Kostanay (Kazakhstan, Asia) resulted in classification accuracies of better than 99%, which showed the applicability of the proposed ResNet-50model to the extraction of reservoirs froma vast amount of moderate resolution images. The framework that was developed in this paper is the first attempt to combine remote sensing big data and the deep learning technique to the recognition of reservoirs at a global scale.
更多查看译文
关键词
Convolutional neural network (CNN),deep learning,Landsat,object recognition,reservoir
AI 理解论文
溯源树
样例
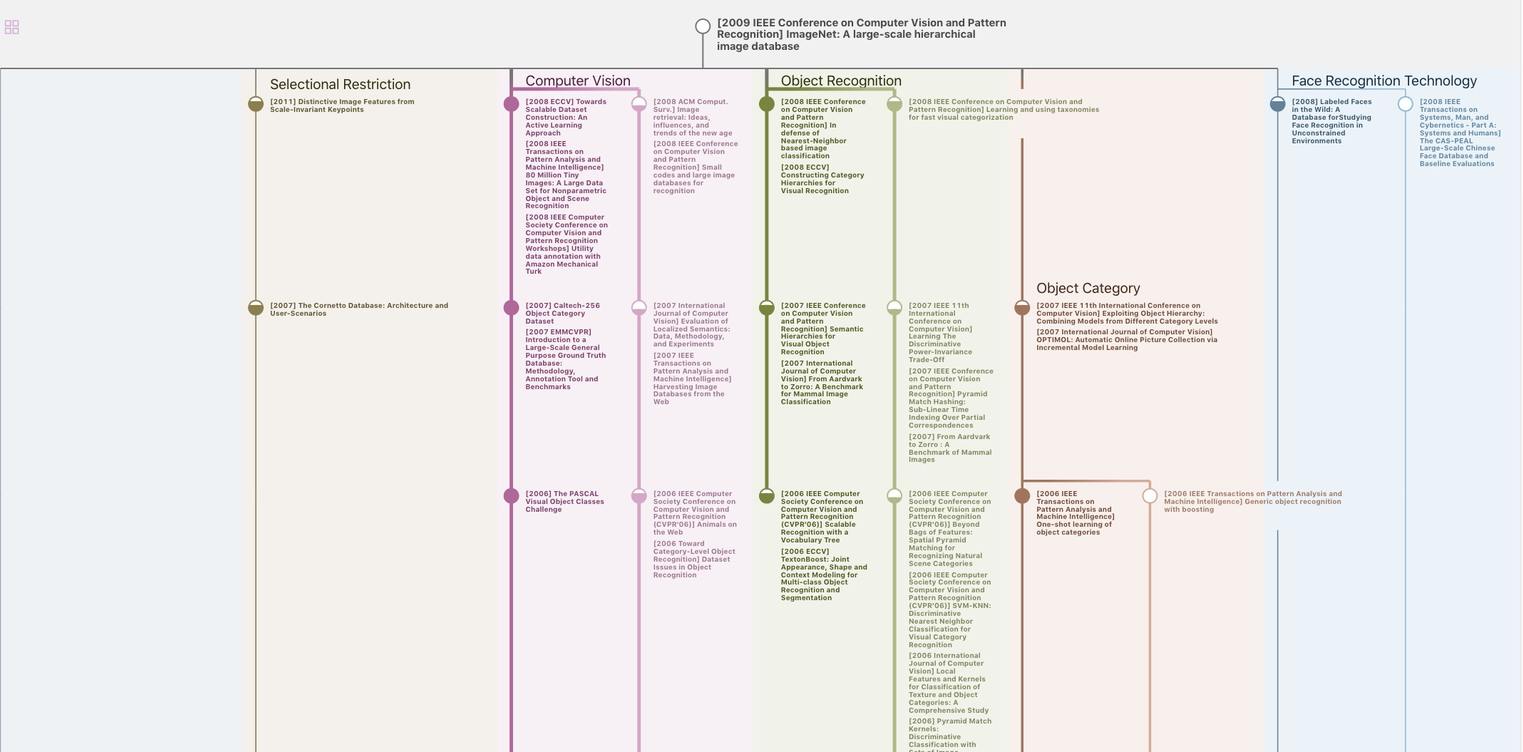
生成溯源树,研究论文发展脉络
Chat Paper
正在生成论文摘要