Gaussian Quadrature and Polynomial Approximation for One-Dimensional Ridge Functions.
SIAM JOURNAL ON SCIENTIFIC COMPUTING(2019)
摘要
Many of the input-parameter-to-output-quantity-of-interest maps that arise in computational science admit a surprising low-dimensional structure, where the outputs vary primarily along a handful of directions in the high-dimensional input space. This type of structure is well modeled by a ridge function, which is a composition of a low-dimensional linear transformation with a nonlinear function. If the goal is to compute statistics of the output-e.g., as in uncertainty quantification or robust design-then one should exploit this low-dimensional structure, when present, to accelerate computations. We develop Gaussian quadrature and the associated polynomial approximation for one-dimensional ridge functions. The key elements of our method are (i) approximating the univariate density of the given linear combination of inputs by repeated convolutions and (ii) a Lanczos Stieltjes method for constructing orthogonal polynomials and Gaussian quadrature.
更多查看译文
关键词
Gaussian quadrature,orthogonal polynomials,ridge functions
AI 理解论文
溯源树
样例
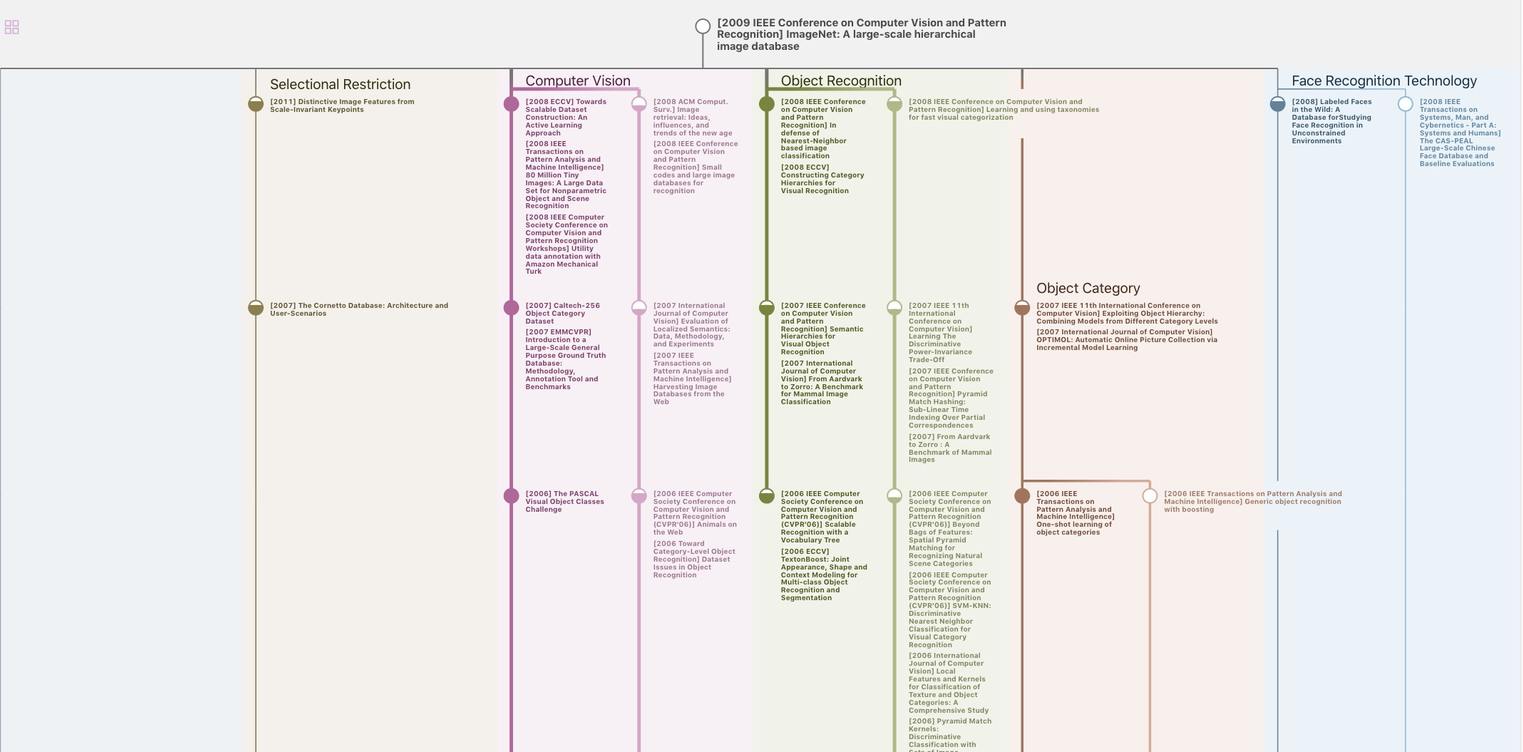
生成溯源树,研究论文发展脉络
Chat Paper
正在生成论文摘要