A Dense Pointnet Plus Plus Architecture For 3d Point Cloud Semantic Segmentation
2019 IEEE INTERNATIONAL GEOSCIENCE AND REMOTE SENSING SYMPOSIUM (IGARSS 2019)(2019)
摘要
3D point cloud data has been widely used in remote sensing mapping because it is not affected by lighting, shadows and other factors. How to improve the performance of semantic segmentation of 3D point cloud data has attracted more and more attention. Previous works connected shallow features in encoders directly with deep features in decoders, which will lead to semantic gap. In this paper, we propose a Dense PointNet++ architecture, called DPNet, for semantic segmentation of 3D point cloud data. In order to weaken the semantic gap, multiple nested up-sampling layers and a series of cumulative, short and long skip link concatenation are introduced in the network to obtain more abundant point cloud features. Grid map and model fusion are used to further correct the results of network segmentation. The experimental results on US3D data set show that DPNet is superior to existing advanced architectures, especially for the categories with small samples. Moreover, DPNet with grid map and model fusion ranks the first place in 2019 IEEE GRSS Data fusion contest 3D point cloud classification challenge.
更多查看译文
关键词
3D point cloud semantic segmentation, DPNet, short and long skip link concatenation, grid map
AI 理解论文
溯源树
样例
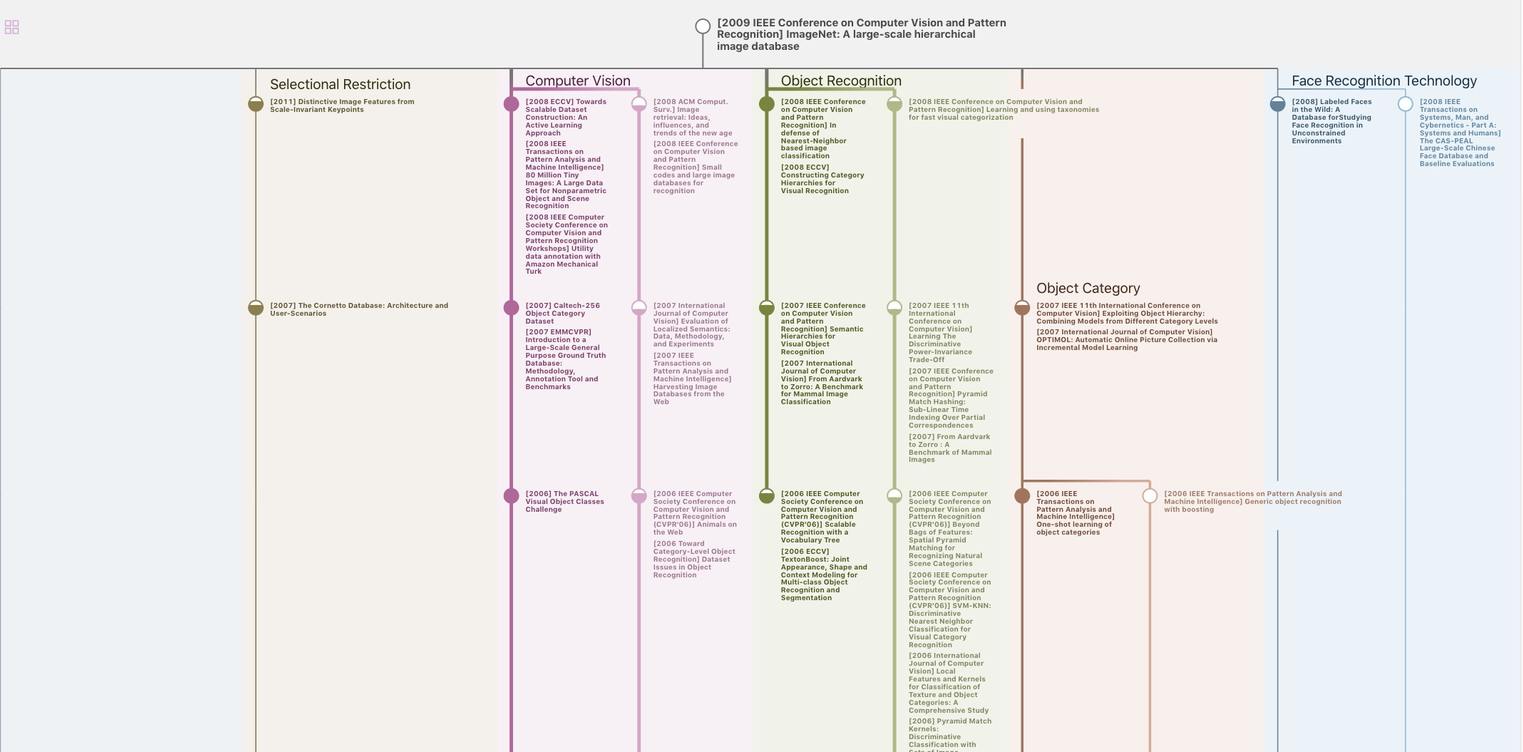
生成溯源树,研究论文发展脉络
Chat Paper
正在生成论文摘要