Modeling and Forecasting Armed Conflict: AutoML with Human-Guided Machine Learning
2019 IEEE International Conference on Big Data (Big Data)(2019)
摘要
Machine learning has made slow inroads into quantitative social science due to both a mismatch of machine learning's strengths to the causal and inferential tasks domain researchers pursue [1] and also a lack of algorithmic training among many domain experts [2]. However, conflict research the empirical examination of political unrest, violence and civil war has seen a growing emphasis on prediction and forecasting models. We describe automated machine learning (AutoML) to identify models, and human-guided machine learning (HGML), and show how these can incorporate domain knowledge and research requirements into model selection and assessment, and provide high quality machine learning pipelines to domain experts comparable to state-of-the-literature solutions. We examine three peer-reviewed papers with predictive models of conflict [3, 4, 5] and run their data through our HGML system using multiple AutoML engines and find this system produces slightly elevated performance on each paper's model, without any ML expertise required of the user. Our research has three takeaways for computational social science. First, predictive models of conflict would benefit from even minimal applications of AutoML; Secondly, human-guided machine learning offers the attractive option of constraining AutoML systems to address the kinds of questions conflict researchers assess with predictive models; Finally, current existing AutoML implementations produce divergent solutions and so can be productively harnessed in parallel.
更多查看译文
关键词
Human-guided machine learning,Automated machine learning (AutoML),conflict forecasting
AI 理解论文
溯源树
样例
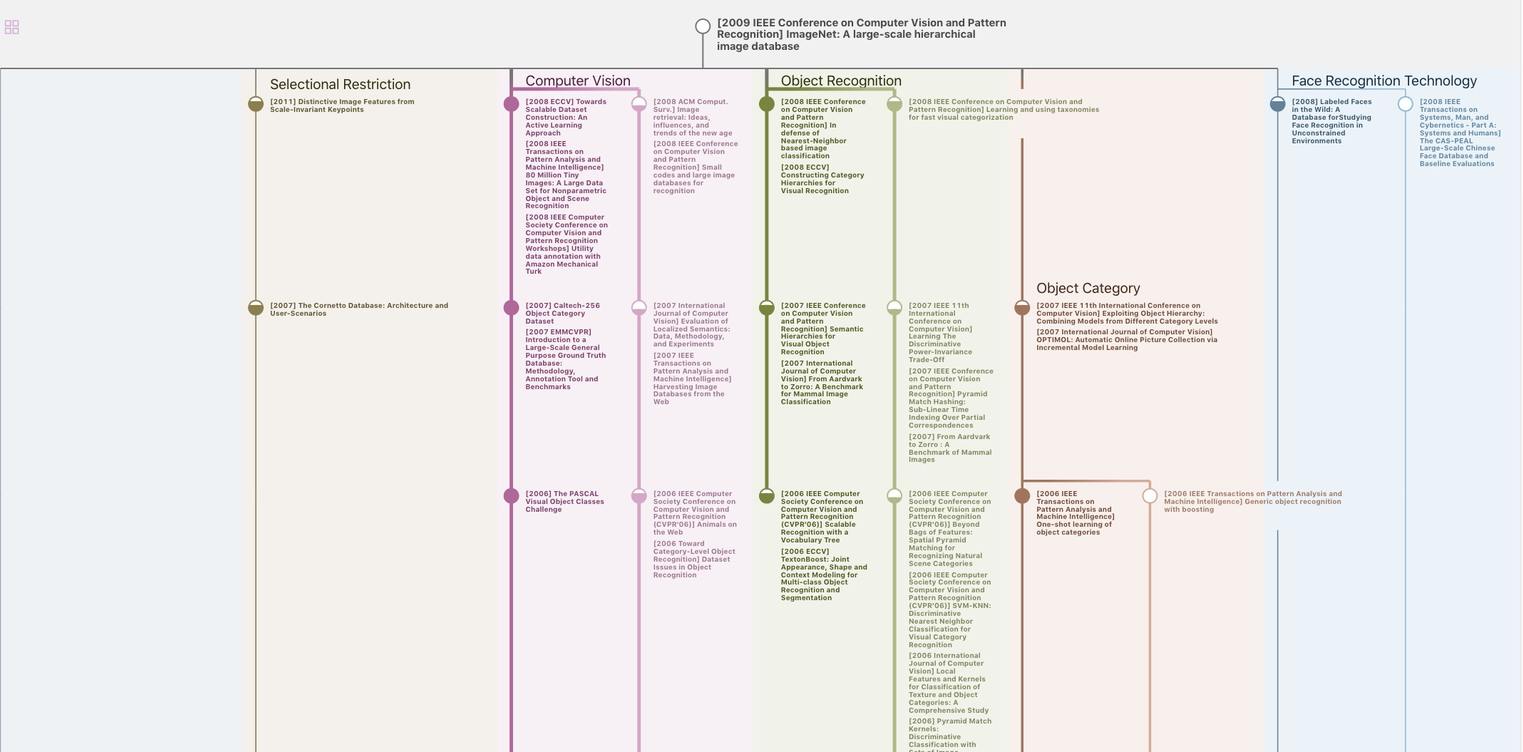
生成溯源树,研究论文发展脉络
Chat Paper
正在生成论文摘要