Inverse Regression for Ridge Recovery: A Data-Driven Approach for Parameter Reduction in Computer Experiments
Statistics and computing(2019)
摘要
Parameter reduction can enable otherwise infeasible design and uncertainty studies with modern computational science models that contain several input parameters. In statistical regression, techniques for sufficient dimension reduction (SDR) use data to reduce the predictor dimension of a regression problem. A computational scientist hoping to use SDR for parameter reduction encounters a problem: a computer prediction is best represented by a deterministic function of the inputs, so data comprised of computer simulation queries fail to satisfy the SDR assumptions. To address this problem, we interpret SDR methods sliced inverse regression (SIR) and sliced average variance estimation (SAVE) as estimating the directions of a ridge function, which is a composition of a low-dimensional linear transformation with a nonlinear function. Within this interpretation, SIR and SAVE estimate matrices of integrals whose column spaces are contained in the ridge directions' span; we analyze and numerically verify convergence of these column spaces as the number of computer model queries increases. Moreover, we show example functions that are not ridge functions but whose inverse conditional moment matrices are low-rank. Consequently, the computational scientist should beware when using SIR and SAVE for parameter reduction, since SIR and SAVE may mistakenly suggest that truly important directions are unimportant.
更多查看译文
关键词
Sufficient dimension reduction,Ridge functions,Ridge recovery
AI 理解论文
溯源树
样例
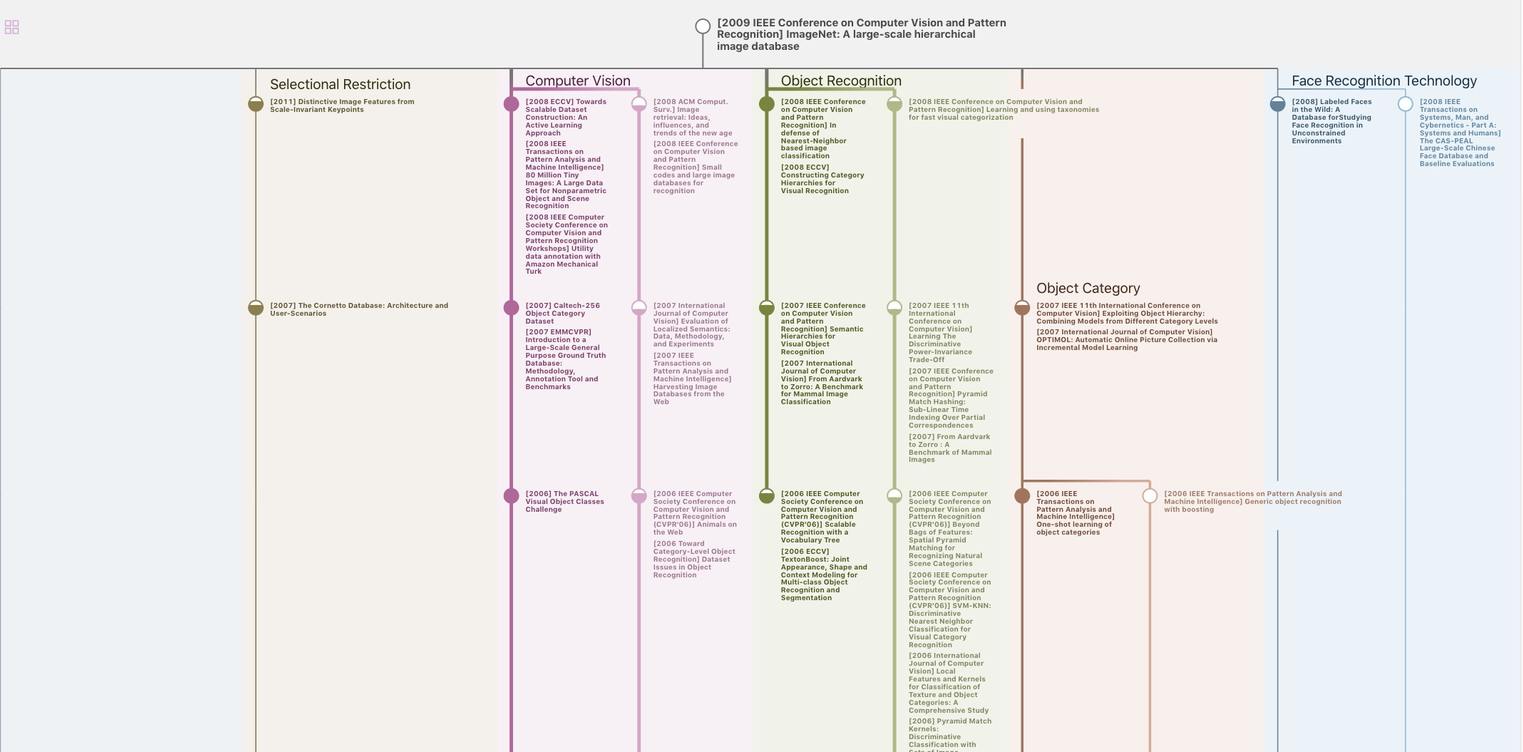
生成溯源树,研究论文发展脉络
Chat Paper
正在生成论文摘要