Visual Task Progress Estimation with Appearance Invariant Embeddings for Robot Control and Planning
IROS(2020)
摘要
To fulfill the vision of full autonomy, robots must be capable of reasoning about the state of the world. In vision-based tasks, this means that a robot must understand the dissimilarities between its current perception of the environment with that of another state. To be of practical use, this dissimilarity must be quantifiable and computed over scenes with different viewpoints, nature (simulated vs. real), and appearances (shape, color, luminosity, etc.). Motivated by this problem, we propose an approach that uses the consistency of the progress among different examples and viewpoints of a task to train a deep neural network to map images into measurable features. Our method builds upon Time-Contrastive Networks (TCNs), originally proposed as a representation for continuous visuomotor skill learning, to train the network using only discrete snapshots taken at different stages of a task such that the network becomes sensitive to differences in task phases. We associate these embeddings to a sequence of images representing gradual task accomplishment, allowing a robot to iteratively query its motion planner with the current visual state to solve long-horizon tasks. We quantify the granularity achieved by the network in recognizing the number of objects in a scene and in measuring the volume of liquid in a cup. Our experiments leverage this granularity to make a mobile robot move a desired number of objects into a storage area and to control the amount of pouring in a cup.
更多查看译文
关键词
visual task progress estimation,appearance invariant embeddings,robot control,visual understanding,deep neural network,time-contrastive networks,mobile robot,TCN,robot planning,object detection
AI 理解论文
溯源树
样例
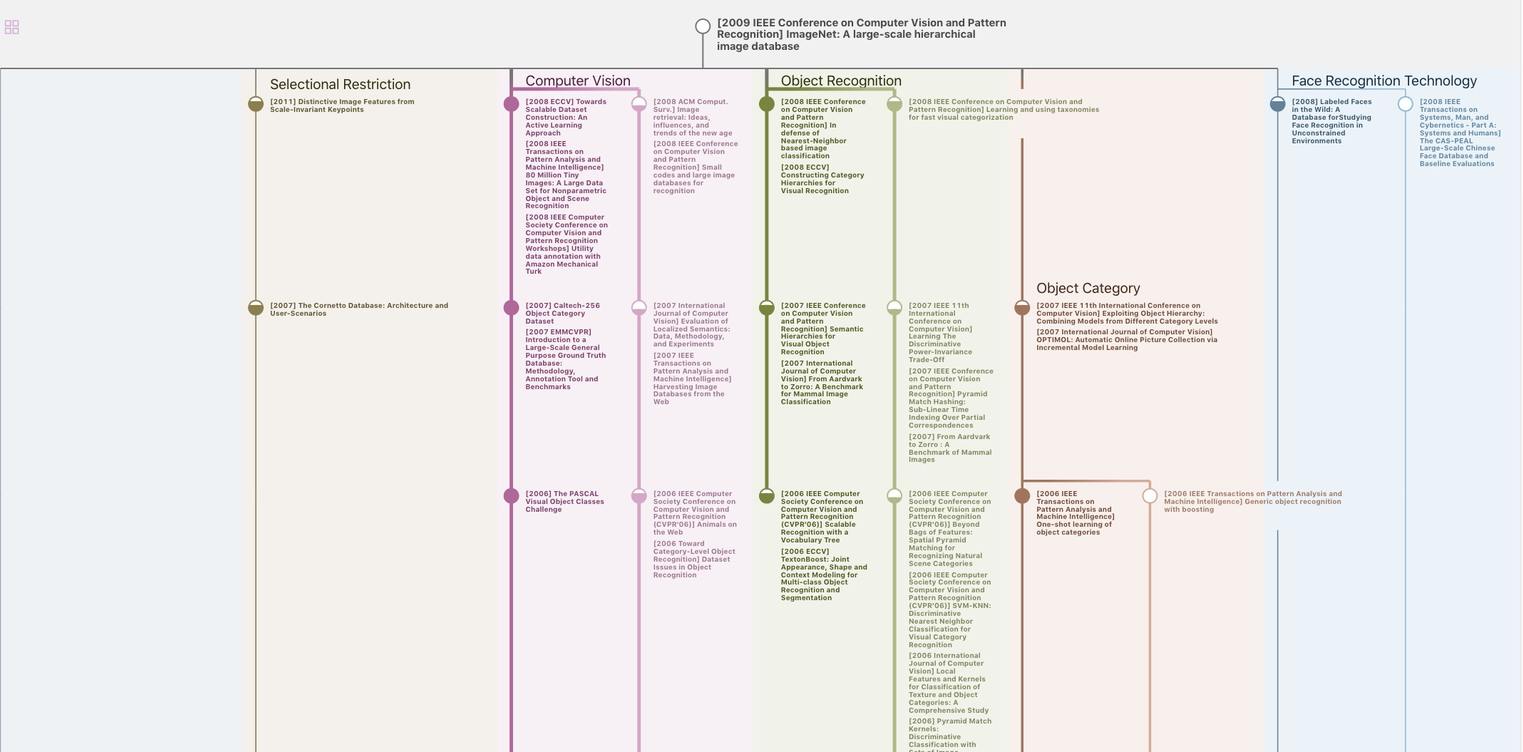
生成溯源树,研究论文发展脉络
Chat Paper
正在生成论文摘要