SpArNet: Sparse Asynchronous Neural Network execution for energy efficient inference
2020 2nd IEEE International Conference on Artificial Intelligence Circuits and Systems (AICAS)(2020)
Abstract
Biological neurons are known to have sparse and asynchronous communications using spikes. Despite our incomplete understanding of processing strategies of the brain, its low energy consumption in fulfilling delicate tasks suggests the existence of energy efficient mechanisms. Inspired by these key factors, we introduce SpArNet, a bio-inspired quantization scheme to convert a pre-trained convolutional neural network to a spiking neural network, with the aim of minimizing the computational load for execution on neuromorphic processors. The proposed scheme has significant advantages over the reference CNN in a reduced number of synaptic operations, and can be used for frequent executions of inference tasks. The computational load of SpArNet is adjusted to the spatio-temporal dynamics of the the input data. We have tested the converted network on two applications (autonomous steering and hand gesture recognition), demonstrating a significant reduction on the number of required synaptic operations.
MoreTranslated text
Key words
SpArNet,bio-inspired quantization scheme,pre-trained convolutional neural network,spiking neural network,computational load,converted network,sparse asynchronous neural network execution,energy efficient inference,biological neurons,sparse communications,asynchronous communications,low energy consumption,autonomous steering,hand gesture recognition
AI Read Science
Must-Reading Tree
Example
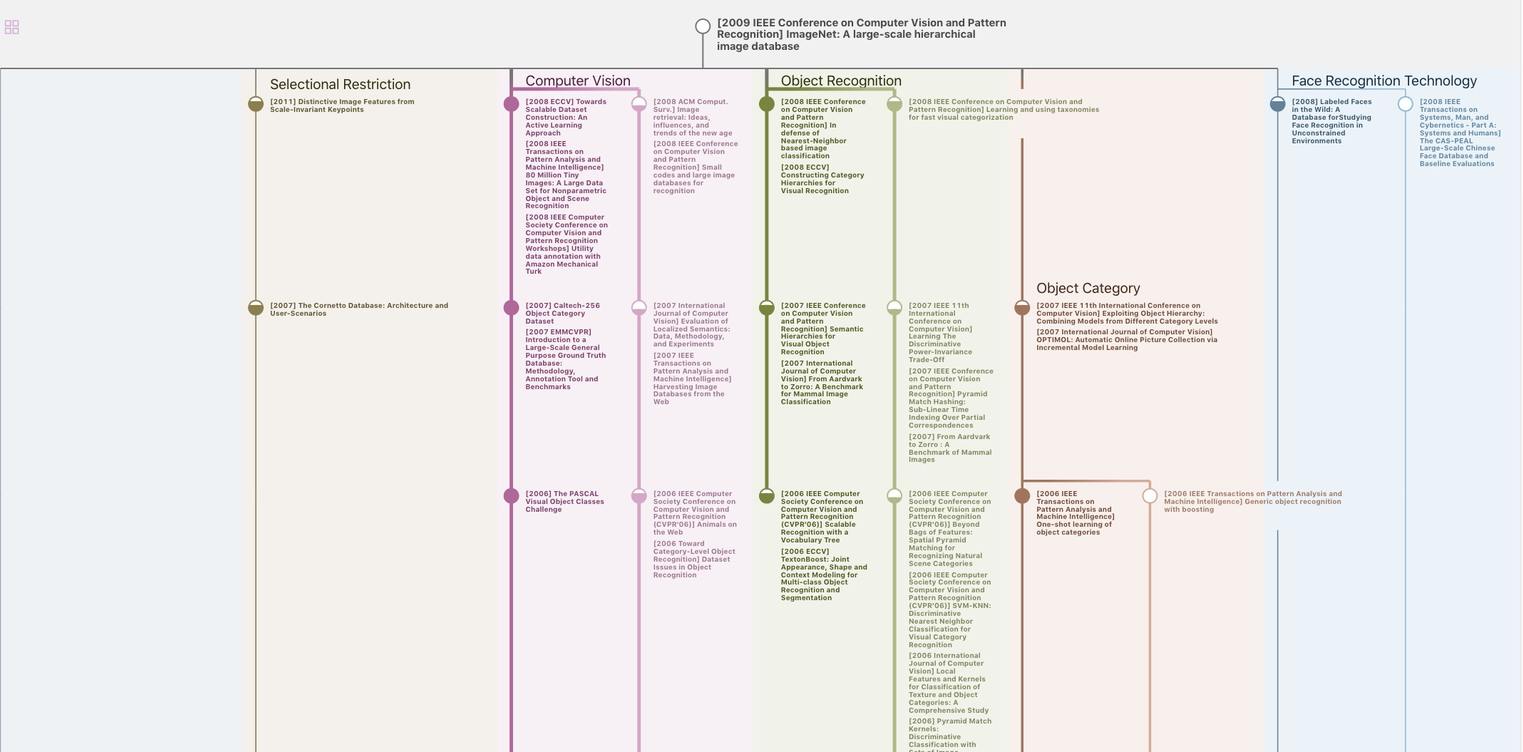
Generate MRT to find the research sequence of this paper
Chat Paper
Summary is being generated by the instructions you defined