Identifying Facemask-wearing Condition Using Image Super-Resolution with Classification Network to Prevent COVID-19
SENSORS(2020)
摘要
The rapid worldwide spread of Coronavirus Disease 2019 (COVID-19) has resulted in a global pandemic. Correct facemask wearing is valuable for infectious disease control, but the effectiveness of facemasks has been diminished, mostly due to improper wearing. However, there have not been any published reports on the automatic identification of facemask-wearing conditions. In this study, we develop a new facemask-wearing condition identification method by combining image super-resolution and classification networks (SRCNet), which quantifies a three-category classification problem based on unconstrained 2D facial images. The proposed algorithm contains four main steps: Image pre-processing, facial detection and cropping, image super-resolution, and facemask-wearing condition identification. Our method was trained and evaluated on the public dataset Medical Masks Dataset containing 3835 images with 671 images of no facemask-wearing, 134 images of incorrect facemask-wearing, and 3030 images of correct facemask-wearing. Finally, the proposed SRCNet achieved 98.70% accuracy and outperformed traditional end-to-end image classification methods using deep learning without image super-resolution by over 1.5% in kappa. Our findings indicate that the proposed SRCNet can achieve high-accuracy identification of facemask-wearing conditions, thus having potential applications in epidemic prevention involving COVID-19.
更多查看译文
关键词
facial recognition,convolutional neural network,image super-resolution,facemask-wearing condition,deep learning,SRCNet,COVID-19
AI 理解论文
溯源树
样例
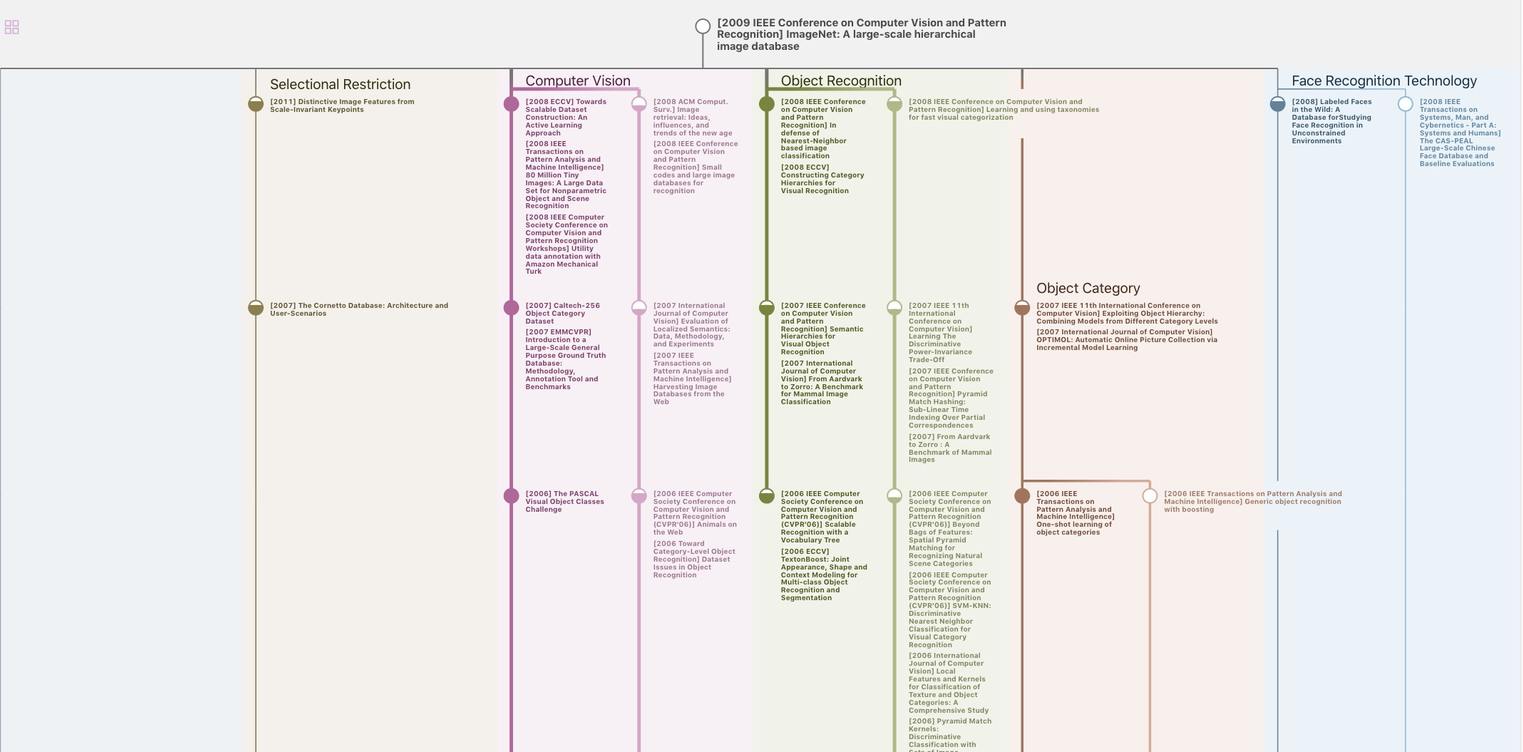
生成溯源树,研究论文发展脉络
Chat Paper
正在生成论文摘要