Navigation in Complex Networks Using Random Walk Theory and Principal Component Analysis
2019 16th International Computer Conference on Wavelet Active Media Technology and Information Processing(2019)
摘要
With quickly increasing size of complex network, the traditional shortest path routing strongly restricts the ability and scalability of navigation due to the requirement of global network topology. To overcome the drawbacks, we assume a hidden metric space for embedding complex network via random walk theory and principal component analysis. Then, based on the resulting hidden metric space, the distances between pairs of nodes are computed to navigate messages from source to target nodes according to the greedy routing. Because the greedy routing only demands the local network topology, the scalability of navigation is reserved. Furthermore, based on the evaluation metrics, i.e., successful routing rate, hop length, and stretch, we show the greed routing based on network embedding enables the better ability of navigation in both synthetic and real networks.
更多查看译文
关键词
Navigation,Hidden metric space,Random walk,Principal component analysis,Complex network
AI 理解论文
溯源树
样例
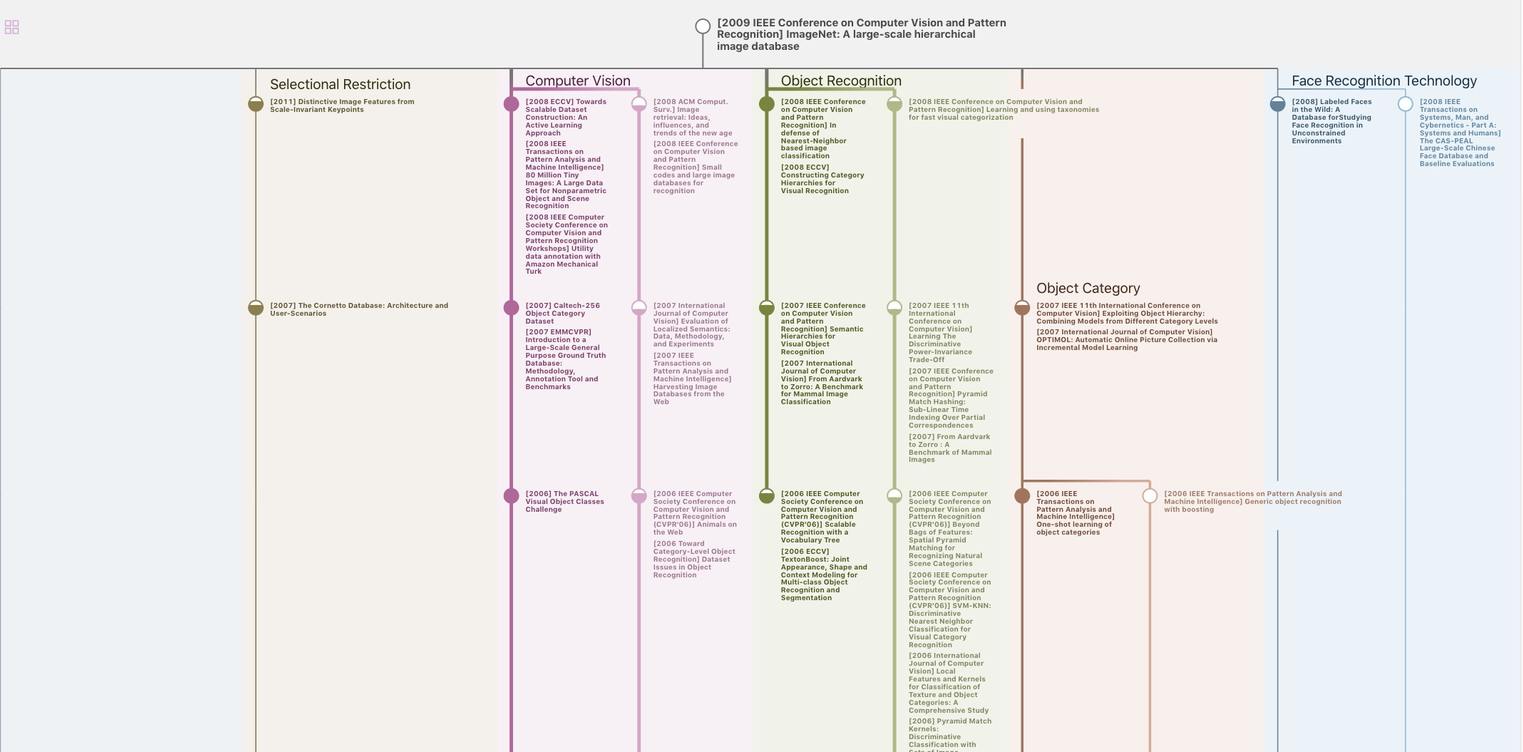
生成溯源树,研究论文发展脉络
Chat Paper
正在生成论文摘要