Efficient Training On Edge Devices Using Online Quantization
PROCEEDINGS OF THE 2020 DESIGN, AUTOMATION & TEST IN EUROPE CONFERENCE & EXHIBITION (DATE 2020)(2020)
摘要
Sensor-specific calibration functions offer superior performance over global models and single-step calibration procedures but require prohibitive levels of sampling in the input feature space. Sensor self-calibration by gathering training data through collaborative calibration or self-analyzing predictive results allows these sensors to gather sufficient information. Resource-constrained edge devices are then stuck between high communication costs for transmitting training data to a centralized server and high memory requirements for storing data lo y. We propose online da.t quantization that maximizes the diversity of input features, maintaining a representative set of data from a larger stream of training data points. We test the effectiveness of online da ".t quantization on two real -world datasets: air quality 'bration and power prediction modeling. Online Dataset Quantization outperforms reservoir sampling and performs equally to offline methods.
更多查看译文
关键词
sensor self-calibration,resource-constrained edge devices,online dataset quantization,air quality calibration,power prediction modeling,sensor-specific calibration functions,Internet of Things
AI 理解论文
溯源树
样例
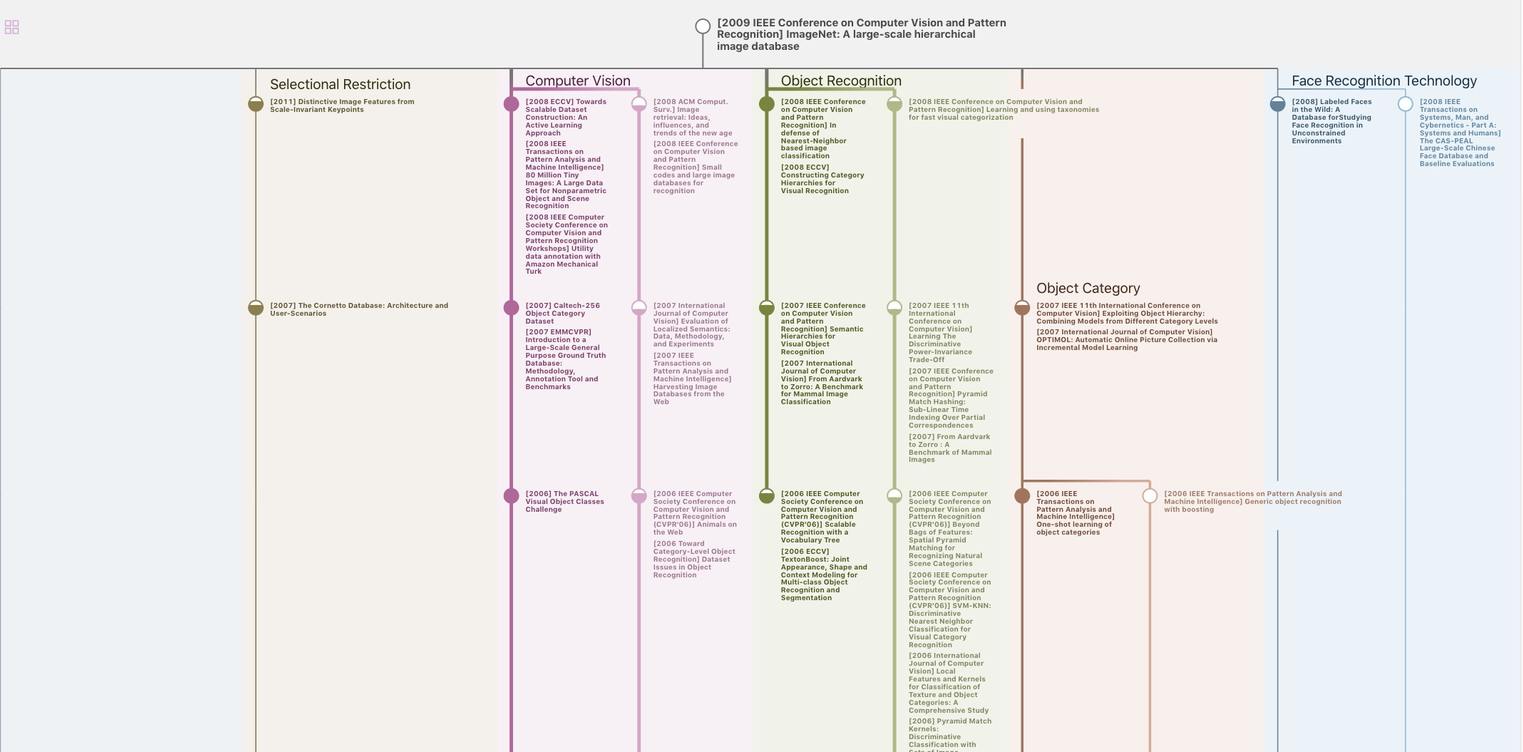
生成溯源树,研究论文发展脉络
Chat Paper
正在生成论文摘要