Learning Classifiers from Declarative Language
semanticscholar(2018)
摘要
Humans can efficiently learn new concepts using natural language communications. For example, a doctor can describe the concept of a malignant tumor through explanations such as ‘large tumors are usually malignant’. Here, the explanation does not only specify what attribute is important for learning the target concept (large size of a tumor), but the quantifier ‘usually’ also describes an expectation of its occurrence under the target model. In this work, we propose a framework through which such human advice can be converted to probabilistic constraints, which can drive the training of classification models without access to any labeled instances . We use semantic parsing to map sentences to probabilistic assertions that are grounded in observable attributes of the data, and employ a training framework that depends on the differential associative strength of linguistic quantifiers (e.g., ‘usually’ vs ‘always’). Our preliminary experiments show that this paradigm can reduce sample complexity of learning, and represents an encouraging direction for guiding machine learning using declarative knowledge.
更多查看译文
AI 理解论文
溯源树
样例
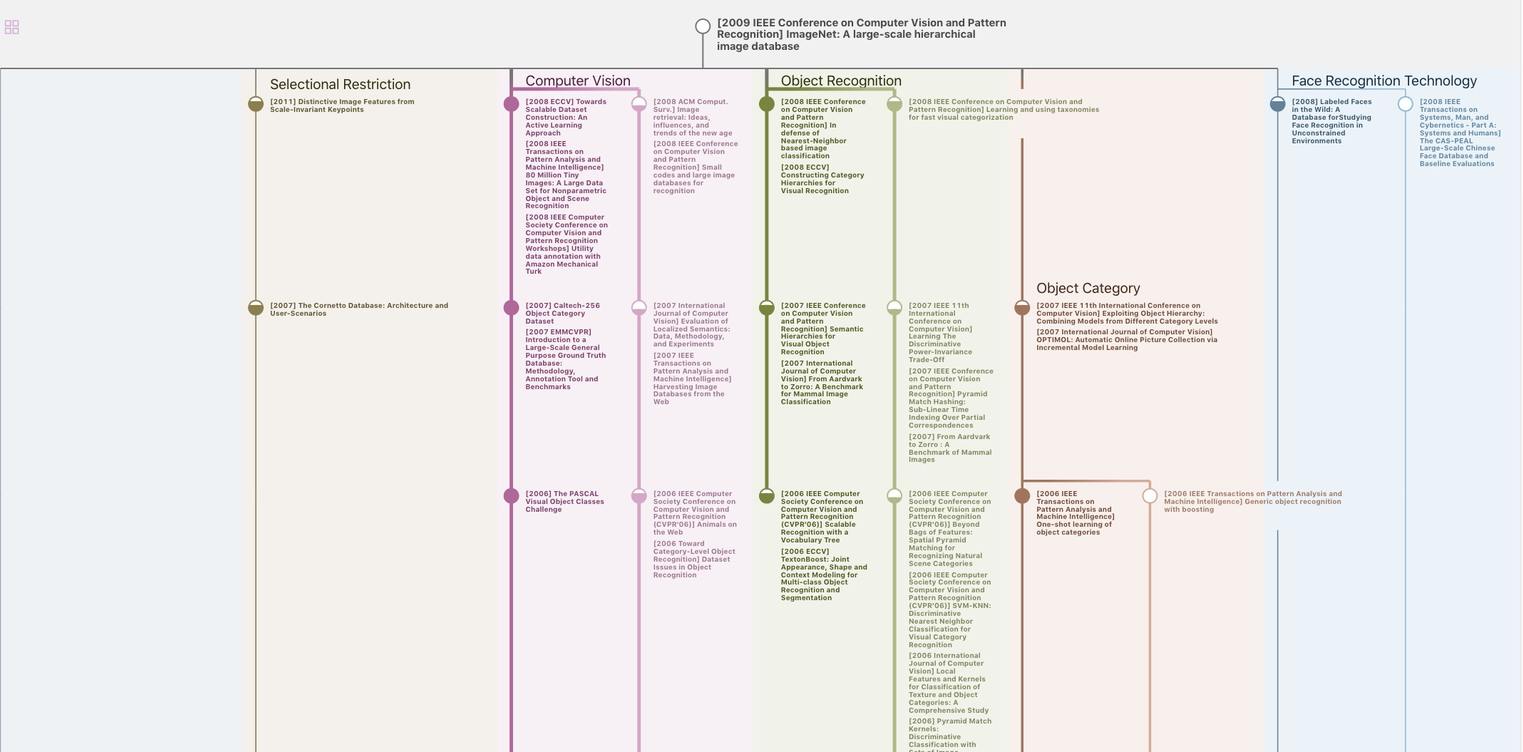
生成溯源树,研究论文发展脉络
Chat Paper
正在生成论文摘要