Default Project : QANet for SQuAD 2 . 0
semanticscholar(2019)
摘要
The Stanford Question Answering Dataset(SQuAD)[10] has spurred many novel architectures for extractive question answering, but there still remains the need for models which can handle unanswerable questions and that can train and predict efficiently. One such efficient architecture is QANet[13] which replaces RNNs with Transformer-like encoder blocks. We implement this architecture and improve its performance beyond a well tuned baseline. We do this by tweaking the architecture, incorporating some tag features as mentioned in DrQA[2], experiment with augmented loss functions, and by performing data augmentation through back translation. We also experimented with variants of local attention. We achieve a test F1 of 63.8 and a dev F1 of 68.0 with a single model in the non-PCE category.
更多查看译文
AI 理解论文
溯源树
样例
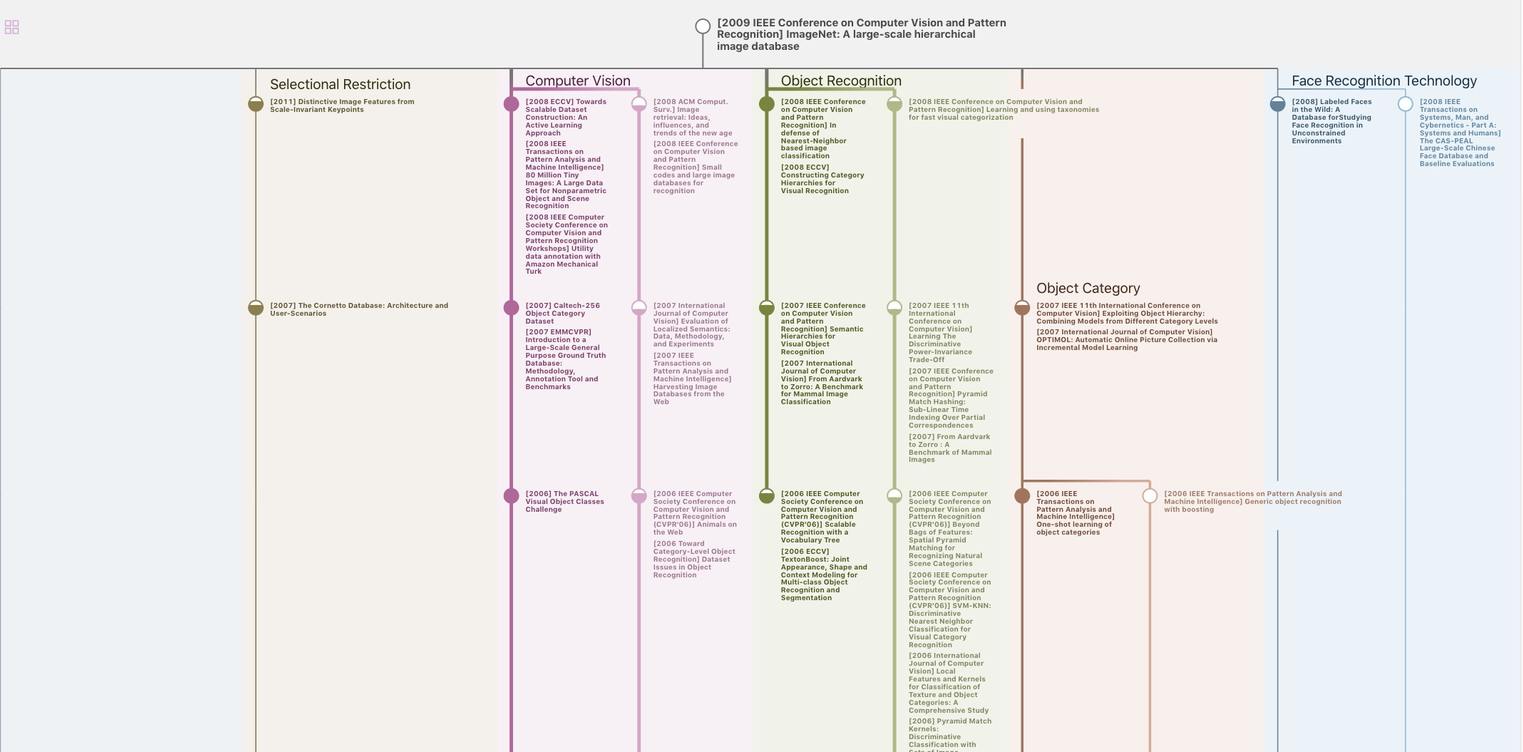
生成溯源树,研究论文发展脉络
Chat Paper
正在生成论文摘要