Poster session booklet
semanticscholar(2019)
摘要
ToF depth data is affected by a high level of noise and by Multi-Path Interference corruption. Machine learning has seldom been applied for ToF denoising due to the limited availability of labeled real data. Here, a CNN is trained on synthetic data in a supervised way and an adversarial learning strategy is used to perform unsupervised domain adaptation to real world data, avoiding the domain shift issue. Experimental results demonstrate that our method outperforms state-of-the-art techniques. Contact: gianluca.agresti@phd.unipd.it Presentation Type: Poster Date: Monday 8 July 2019 Time: 21:30 Poster Session: 1
更多查看译文
AI 理解论文
溯源树
样例
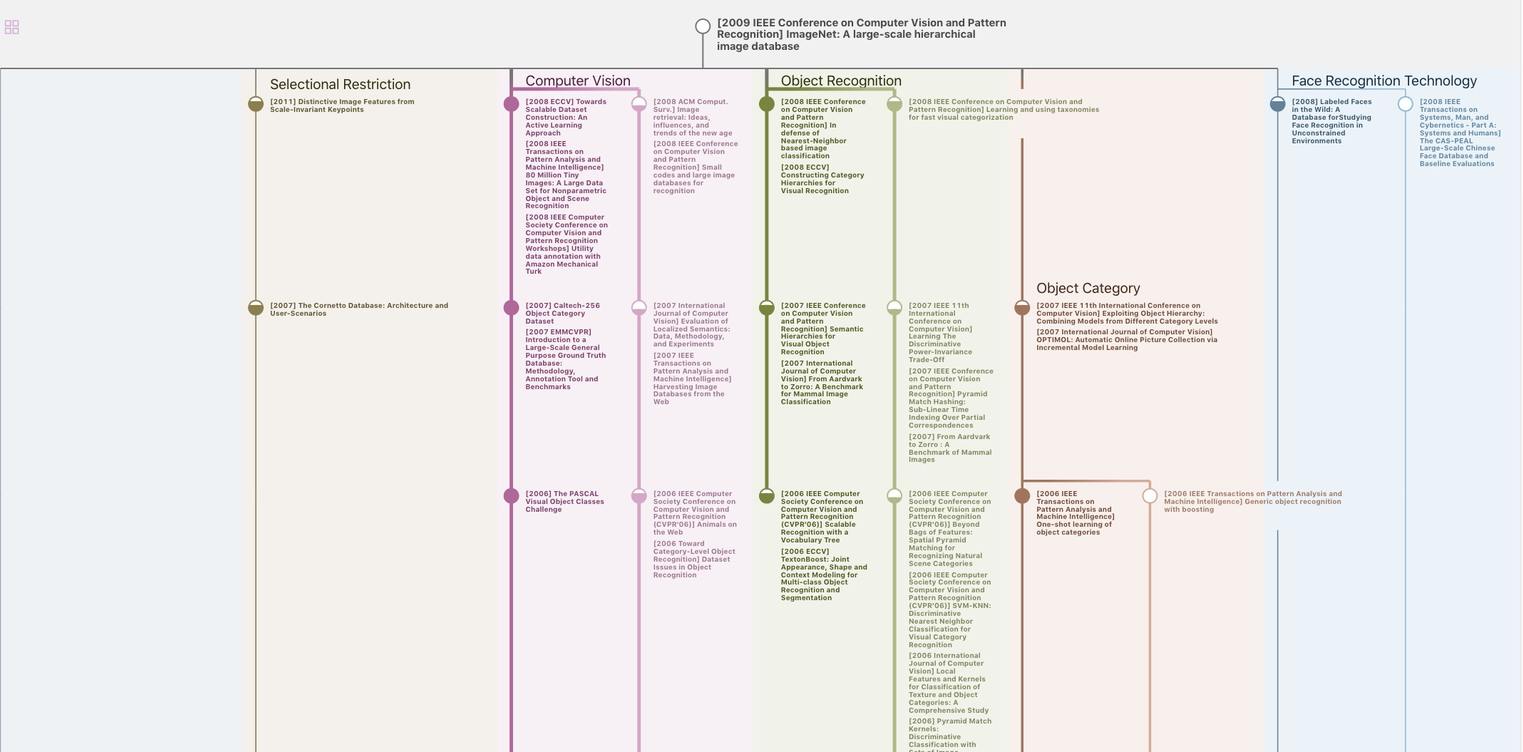
生成溯源树,研究论文发展脉络
Chat Paper
正在生成论文摘要