Skill discovery with well-defined objectives
semanticscholar(2019)
摘要
While many skill discovery methods have been proposed to accelerate learning and planning, most are based on heuristic methods without clear connections to how the skills impact the agent’s objective. As such, the conditions under which the algorithms are effective is often unclear. We claim that we should pursue skill discovery algorithms with explicit relationships to the objective of the agent to understand in what scenarios skill discovery methods are useful. We analyze two scenarios, planning and reinforcement learning and show that we are able to give bounds to the performance of the option discovery algorithms. For planning, we show that the problem of finding a set of options which minimizes the planning time is NP-hard, and give a polynomial-time algorithm that is approximately optimal under certain conditions. For reinforcement learning, we target goal-based tasks with sparse reward where the agent has to navigate through the state-space to reach the goal state without any reward signals other than the goal state. We show that the difficulty of discovering a distant rewarding state in an MDP is bounded by the expected cover time of a random walk over the graph induced by the MDP’s transition dynamics. We therefore propose an algorithm which finds an option which provably diminishes the expected cover time.
更多查看译文
AI 理解论文
溯源树
样例
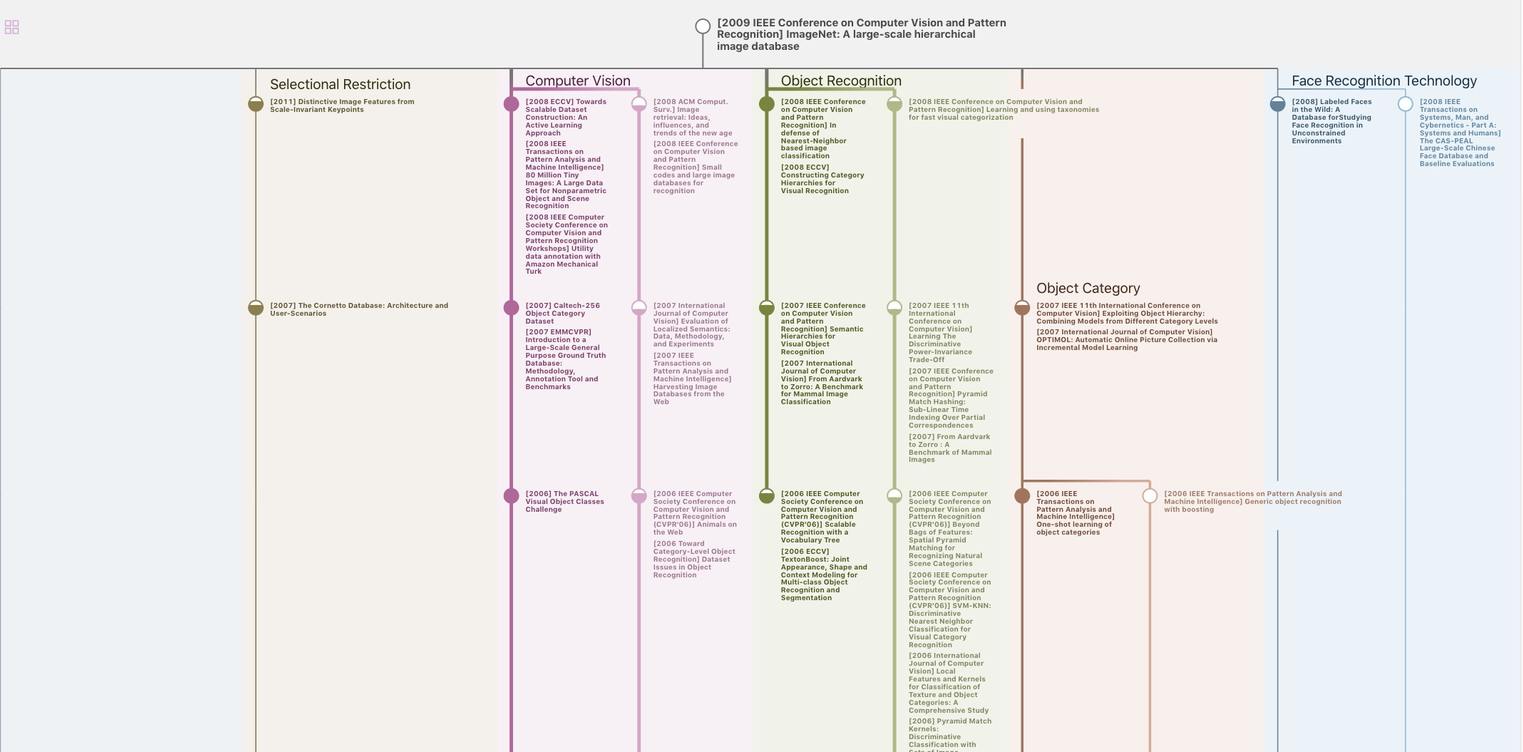
生成溯源树,研究论文发展脉络
Chat Paper
正在生成论文摘要