A composite method to infer drug resistance with mixed genomic data
biorxiv(2020)
摘要
Background: The increasing incidence of drug resistance in tuberculosis and other infectious diseases poses an escalating cause for concern, emphasizing the urgent need to devise robust computational and molecular methods identify drug resistant strains. Although machine learning-based approaches using whole-genome sequence data can facilitate the inference of drug resistance, current implementations do not optimally take advantage of information in public databases and are not robust for small sample sizes and mixed attribute types. Results: In this paper we introduce the Composite MetaDistance method, an approach for feature selection and classification of high-dimensional, unbalanced datasets with mixed attribute features from various data sources. We introduce a mixed-attribute, multi-view distance function to calculate distances between samples, with optimal handling of nominal features and different feature views. We also introduce a novel feature set for drug resistance prediction in Mycobacterium tuberculosis, using data from diverse sources. We compare the performance of Composite MetaDistance to multiple machine learning algorithms for Mycobacterium tuberculosis drug resistance prediction for three drugs. Composite MetaDistance consistently outperforms existing algorithms for small sample training sets, and performs as well as other algorithms for training sets with larger sample sizes. Conclusion: The feature set formulation introduced in this paper is utilizes mutational and publicly available information for each gene, and is much richer than ever devised previously. The prediction algorithm, Composite MetaDistance, is sample size agnostic and robust especially given small sample sizes. Proper handling of nominal features improves performance even with a very small number of nominal features. We expect Composite MetaDistance to be even more robust for datasets with a higher percentage of nominal features. The algorithm is application independent and can be used for any mixed attribute dataset.
更多查看译文
关键词
Machine learning,Mixed attributes,Multi-view,Feature selection,Unbalanced data,Small samples,Drug resistance prediction,<italic>Mycobacterium tuberculosis</italic>
AI 理解论文
溯源树
样例
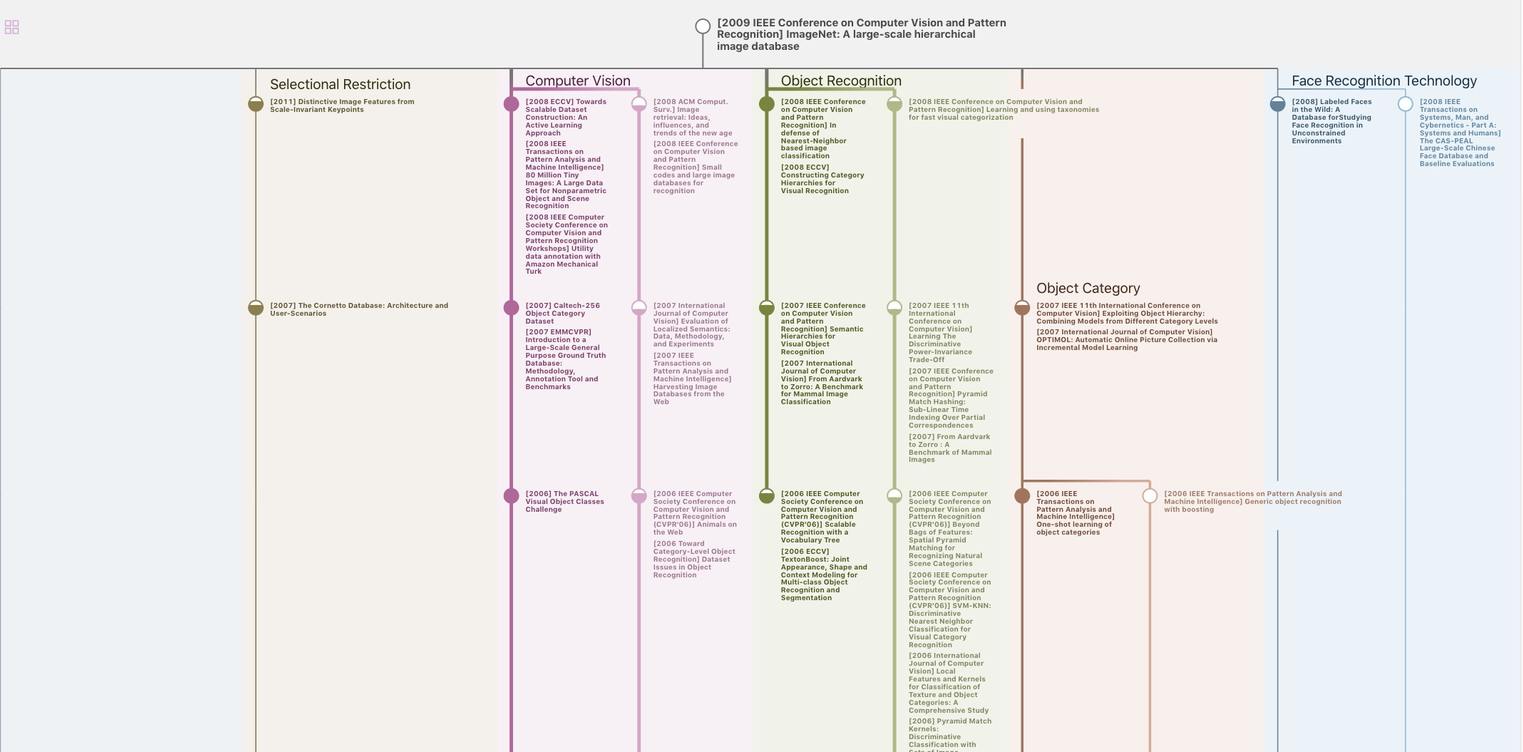
生成溯源树,研究论文发展脉络
Chat Paper
正在生成论文摘要