A Novel Tax Evasion Detection Framework via Fused Transaction Network Representation
2020 IEEE 44th Annual Computers, Software, and Applications Conference (COMPSAC)(2020)
摘要
Tax evasion usually refers to the false declaration of taxpayers to reduce their tax obligations; this type of behavior leads to the loss of taxes and damage to the fair principle of taxation. Tax evasion detection plays a crucial role in reducing tax revenue loss. Currently, efficient auditing methods mainly include traditional data-mining-oriented methods, which cannot be well adapted to the increasingly complicated transaction relationships between taxpayers. Driven by this requirement, recent studies have been conducted by establishing a transaction network and applying the graphical pattern matching algorithm for tax evasion identification. However, such methods rely on expert experience to extract the tax evasion chart pattern, which is time-consuming and labor-intensive. More importantly, taxpayers' basic attributes are not considered and the dual identity of the taxpayer in the transaction network is not well retained. To address this issue, we have proposed a novel tax evasion detection framework via fused transaction network representation (TED-TNR), to detecting tax evasion based on fused transaction network representation, which jointly embeds transaction network topological information and basic taxpayer attributes into low-dimensional vector space, and considers the dual identity of the taxpayer in the transaction network. Finally, we conducted experimental tests on real-world tax data, revealing the superiority of our method, compared with state-of-the-art models.
更多查看译文
关键词
Transaction network, Network representation learning, Tax evasion detection
AI 理解论文
溯源树
样例
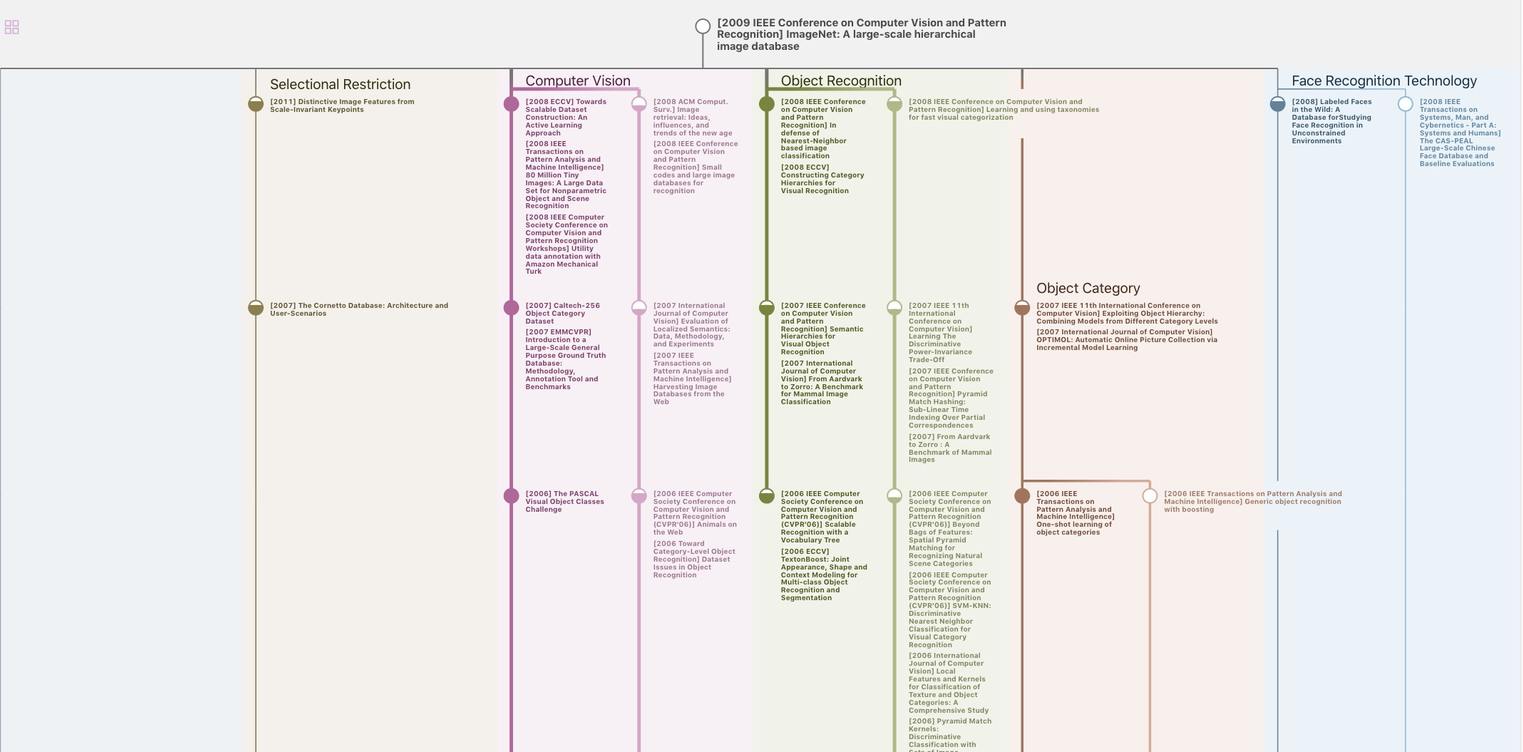
生成溯源树,研究论文发展脉络
Chat Paper
正在生成论文摘要