A Novel Object Re-Track Framework for 3D Point Clouds
MM '20: The 28th ACM International Conference on Multimedia Seattle WA USA October, 2020(2020)
摘要
3D point cloud data is an important data source for autonomous vehicles to perceive the surroundings. Achieving accurate object tracking of 3D point clouds has become a challenging task. In this paper, we propose a 3D object two-stage re-track framework directly utilizing point clouds as the input, without using the ground truth as the reference box. The framework consists of a coarse stage and a fine stage. By tracking back the previous T frames and expanding the search space for each frame, we add the fine stage to re-track the lost objects of the coarse stage. Moreover, we design a dense AutoEncoder to enhance the discrimination in the latent space and improve shape completion performance, thus improving tracking performance. A Sample Update Strategy is also proposed to aggregate similar model shape samples in different frames, which improves the quality of the model shape. In terms of motion models for the proposed re-track framework, we further compare Kalman Filter with PointLSTM and do an extensive analysis. Finally, we test the re-track framework on the KITTI tracking dataset and outperform the public benchmark by 17.1%/15.5% in Success and Precision, respectively. Our code and model are available at https://github.com/FengZicai/Re-Track.
更多查看译文
关键词
Point Clouds, Object Tracking, Motion Forecasting, 3D Vision
AI 理解论文
溯源树
样例
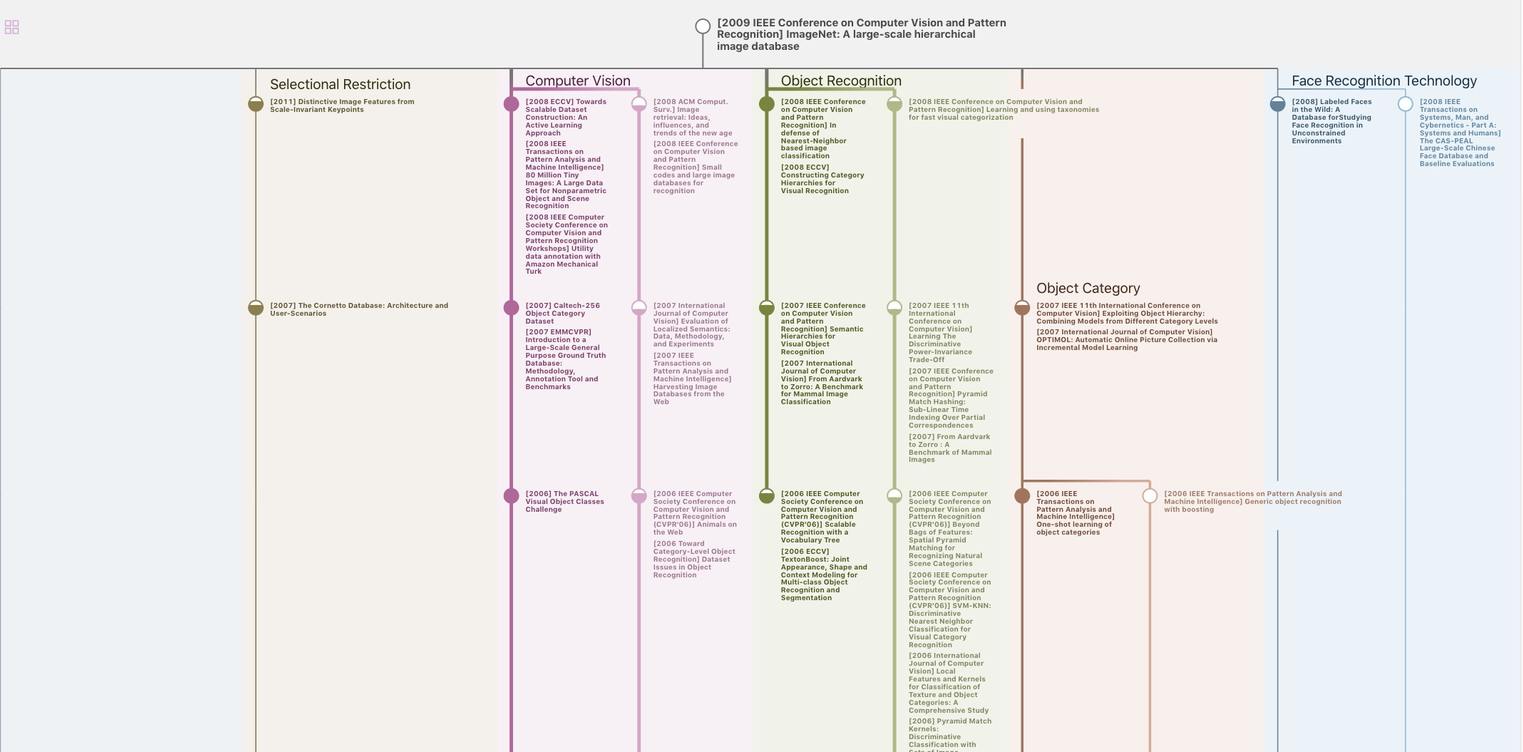
生成溯源树,研究论文发展脉络
Chat Paper
正在生成论文摘要