Masked Autoencoder for Distribution Estimation on Small Structured Data Sets
IEEE transactions on neural networks and learning systems(2021)
摘要
Autoregressive models are among the most successful neural network methods for estimating a distribution from a set of samples. However, these models, such as other neural methods, need large data sets to provide good estimations. We believe that knowing structural information about the data can improve their performance on small data sets. Masked autoencoder for distribution estimation (MADE) is a well-structured density estimator, which alters a simple autoencoder by setting a set of masks on its connections to satisfy the autoregressive condition. Nevertheless, this model does not benefit from extra information that we might know about the structure of the data. This information can especially be advantageous in case of training on small data sets. In this article, we propose two autoencoders for estimating the density of a small set of observations, where the data have a known Markov random field (MRF) structure. These methods modify the masking process of MADE, according to conditional dependencies inferred from the MRF structure, to reduce either the model complexity or the problem complexity. We compare the proposed methods with some related binary, discrete, and continuous density estimators on MNIST, binarized MNIST, OCR-letters, and two synthetic data sets.
更多查看译文
关键词
Estimation,Computational modeling,Data models,Neural networks,Complexity theory,Training,Markov processes,Deep learning,density estimation,Markov random field (MRF),masked autoencoder
AI 理解论文
溯源树
样例
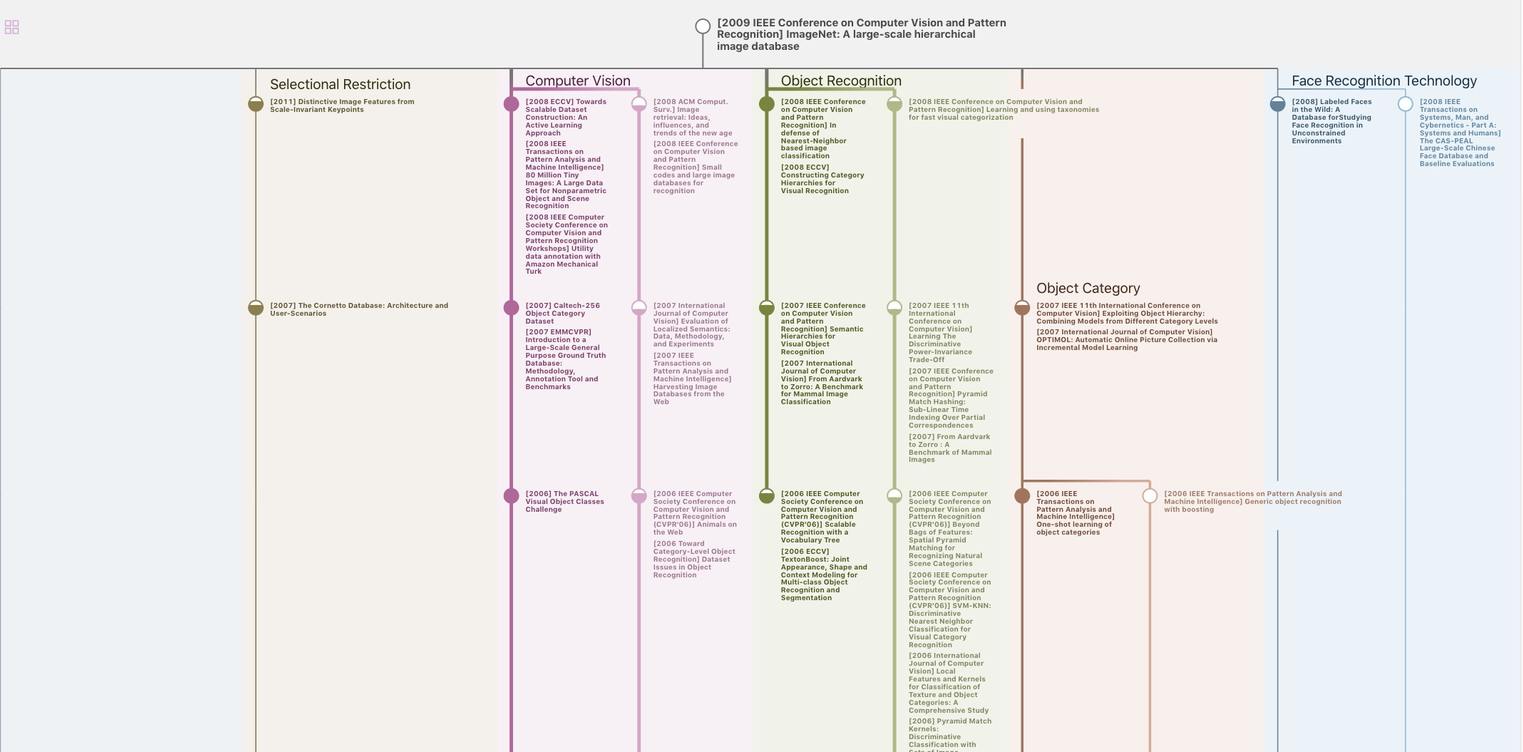
生成溯源树,研究论文发展脉络
Chat Paper
正在生成论文摘要