Learning Temporal Dynamics from Cycles in Narrated Video.
ICCV(2021)
摘要
Learning to model how the world changes as time elapses has proven a challenging problem for the computer vision community. We propose a self-supervised solution to this problem using temporal cycle consistency jointly in vision and language, training on narrated video. Our model learns modality-agnostic functions to predict forward and backward in time, which must undo each other when composed. This constraint leads to the discovery of high-level transitions between moments in time, since such transitions are easily inverted and shared across modalities. We justify the design of our model with an ablation study on different configurations of the cycle consistency problem. We then show qualitatively and quantitatively that our approach yields a meaningful, high-level model of the future and past. We apply the learned dynamics model without further training to various tasks, such as predicting future action and temporally ordering sets of images.
更多查看译文
关键词
Vision + language,Video analysis and understanding
AI 理解论文
溯源树
样例
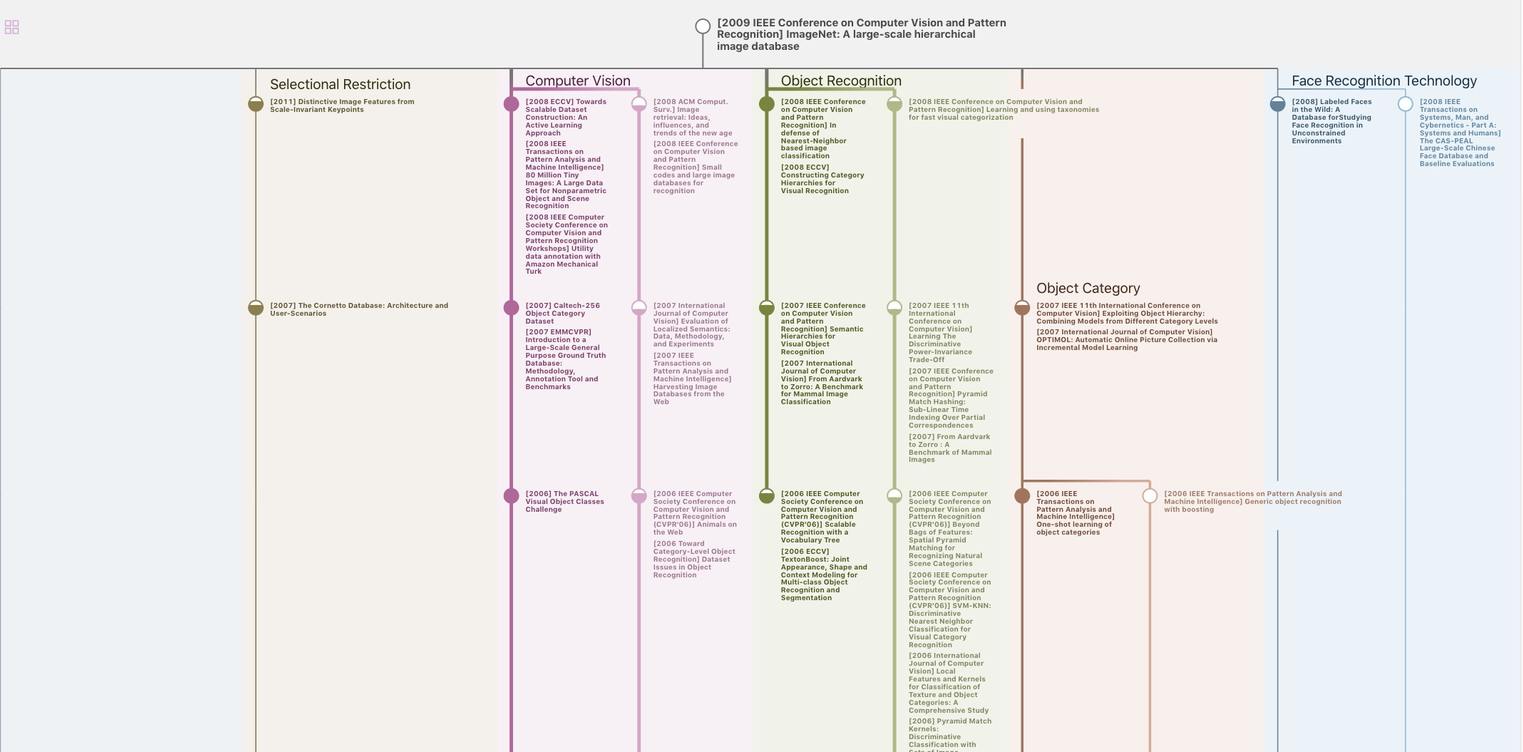
生成溯源树,研究论文发展脉络
Chat Paper
正在生成论文摘要