Scssnet: Learning Spatially-Conditioned Scene Segmentation On Lidar Point Clouds
2020 IEEE INTELLIGENT VEHICLES SYMPOSIUM (IV)(2020)
摘要
This work proposes a spatially-conditioned neural network to perform semantic segmentation and geometric scene completion in 3D on real-world LiDAR data. Spatially-conditioned scene segmentation (SCSSnet) is a representation suitable to encode properties of large 3D scenes at high resolution. A novel sampling strategy encodes free space information from LiDAR scans explicitly and is both simple and effective. We avoid the need for synthetically generated or volumetric ground truth data and are able to train and evaluate our method on semantically annotated LiDAR scans from the Semantic KITTI dataset. Ultimately, our method is able to predict scene geometry as well as a diverse set of semantic classes over a large spatial extent at arbitrary output resolution instead of a fixed discretization of space.Our experiments confirm that the learned scene representation is versatile and powerful and can be used for multiple downstream tasks. We perform point-wise semantic segmentation, point-of-view depth completion and ground plane segmentation. The semantic segmentation performance of our method surpasses the state of the art by a significant margin of 7% mIoU.
更多查看译文
关键词
lidar point clouds,scene segmentation,spatially-conditioned
AI 理解论文
溯源树
样例
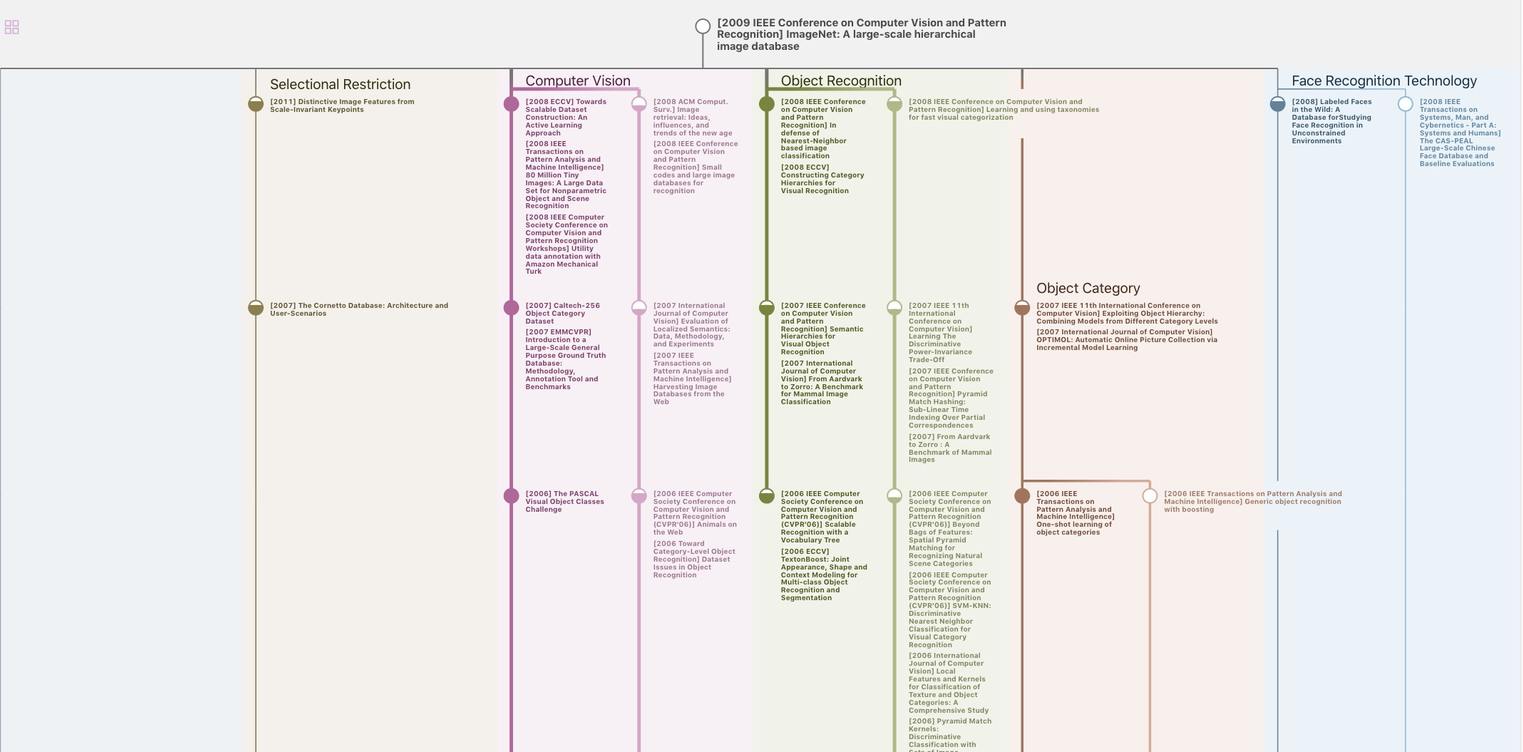
生成溯源树,研究论文发展脉络
Chat Paper
正在生成论文摘要