An Optimal Reduction Of Tv-Denoising To Adaptive Online Learning
24TH INTERNATIONAL CONFERENCE ON ARTIFICIAL INTELLIGENCE AND STATISTICS (AISTATS)(2021)
摘要
We consider the problem of estimating a function from n noisy samples whose discrete Total Variation (TV) is bounded by C-n. We reveal a deep connection to the seemingly disparate problem of Strongly Adaptive online learning (Daniely et al., 2015) and provide an O(n log n) time algorithm that attains the near minimax optimal rate of (O) over tilde (n(1/3)C(n)(2/3)) under squared error loss. The resulting algorithm runs online and optimally adapts to the unknown smoothness parameter C-n. This leads to a new and more versatile alternative to wavelets-based methods for (1) adaptively estimating TV bounded functions; (2) online forecasting of TV bounded trends in time series.
更多查看译文
关键词
online learning,optimal reduction,tv-denoising
AI 理解论文
溯源树
样例
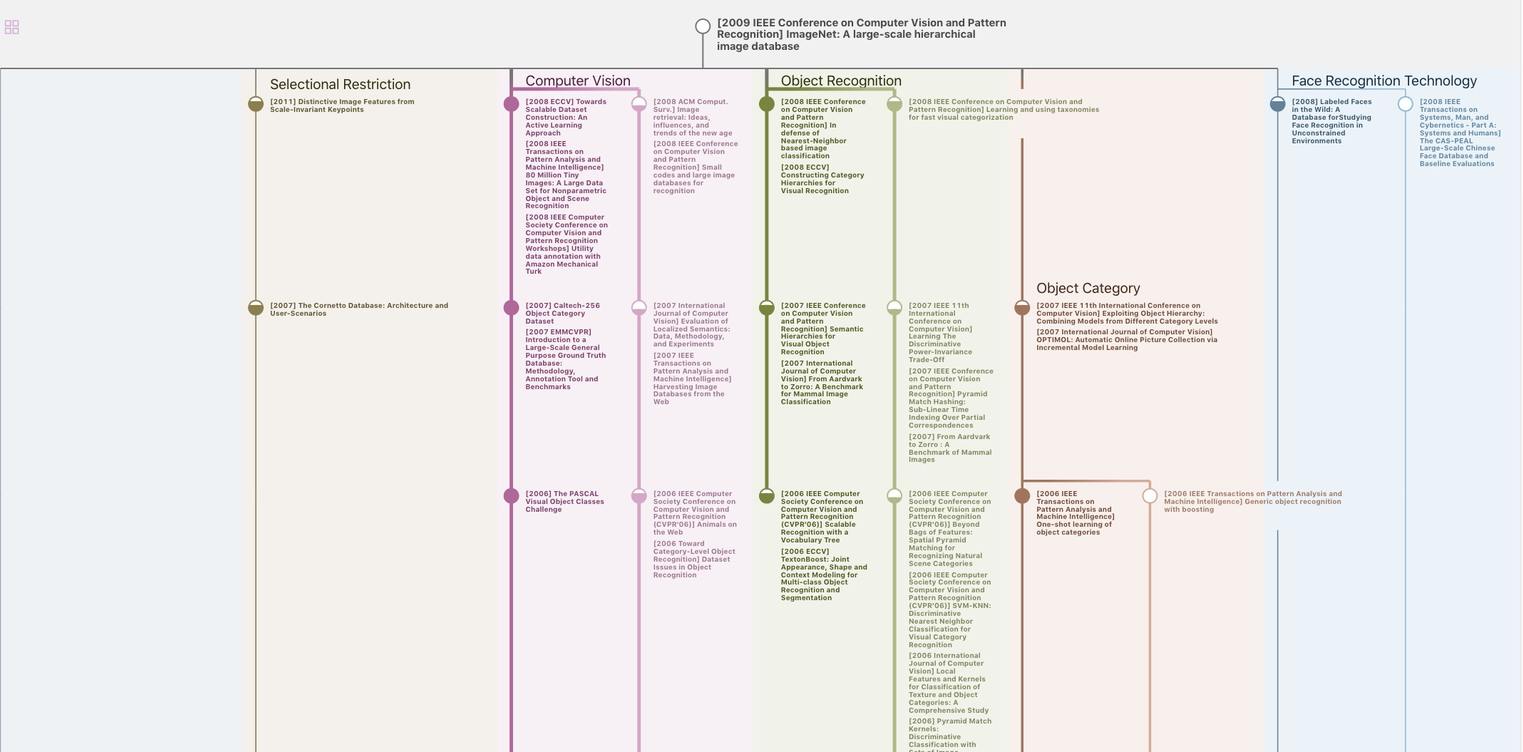
生成溯源树,研究论文发展脉络
Chat Paper
正在生成论文摘要