Designing Synthetic Overhead Imagery to Match a Target Geographic Region: Preliminary Results Training Deep Learning Models
IGARSS 2020 - 2020 IEEE INTERNATIONAL GEOSCIENCE AND REMOTE SENSING SYMPOSIUM(2020)
摘要
Convolutional Neural Networks (CNNs) have dominated performance on benchmark problems for object recognition in remote sensing imagery. However, recent work has shown that they may perform poorly when tested on imagery collected over a geographic location that was not present in its training imagery. In this work we explore the potential of designing synthetic overhead imagery to match a target geographic region (e.g., city or county), after which the synthetic imagery could be used to train deep learning models to recognize the unique visual features of objects/background in the target geographic location. We term this approach geographic domain matching. Towards this goal, we utilize a publicly-available dataset of synthetic overhead imagery, Synthinel-1. We systematically alter individual visual features of Synthinel-1 in an effort to match one real-world testing city: Vienna, Austria. We then evaluate whether these individual alterations improve the performance benefits of Synthinel-1 on Vienna and other cities. The results suggest that our proposed methodologies for altering the synthetic imagery to match Vienna were effective, thereby taking first step towards developing methods for designing synthetic overhead imagery for domain matching.
更多查看译文
关键词
overhead imagery,segmentation,domain adaptation,building segmentation
AI 理解论文
溯源树
样例
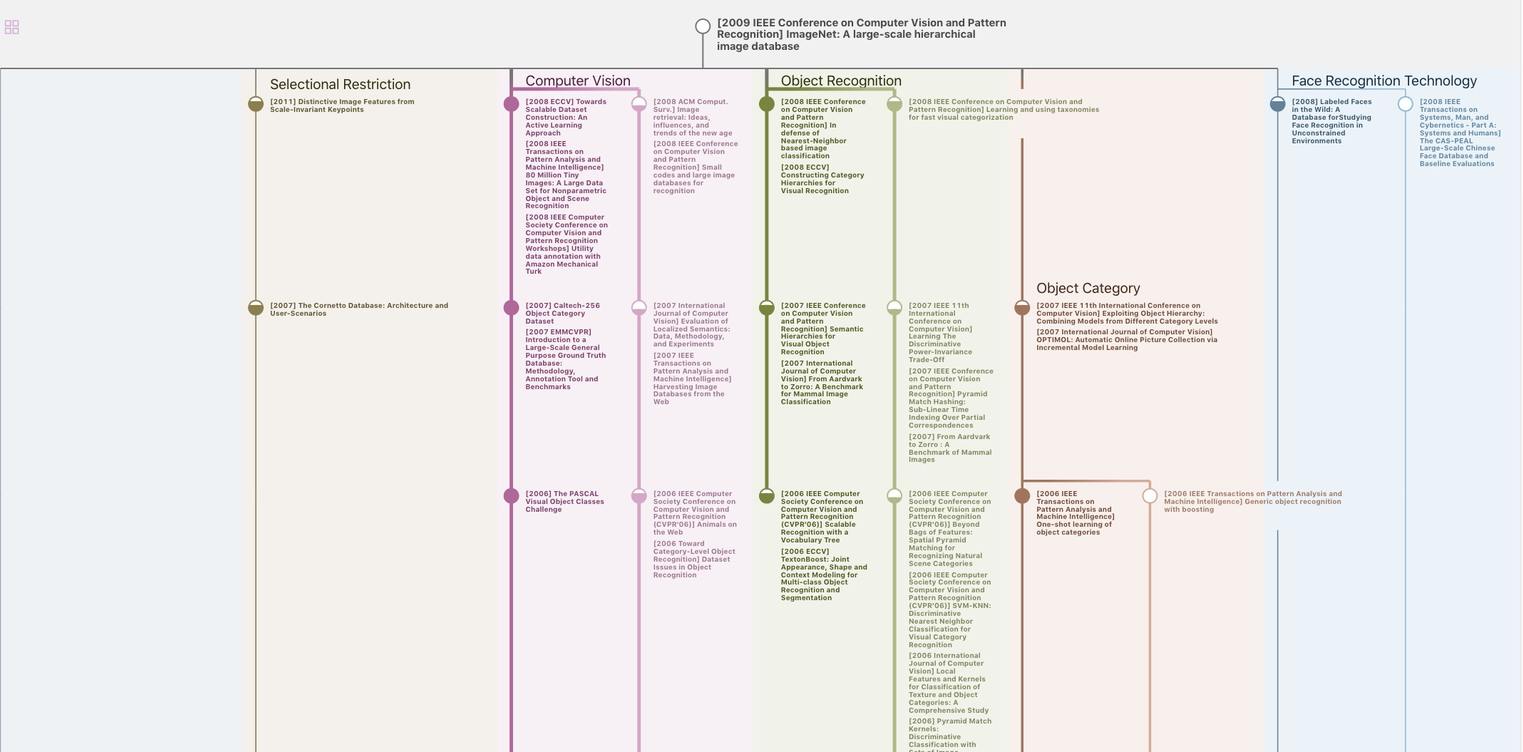
生成溯源树,研究论文发展脉络
Chat Paper
正在生成论文摘要