Biological data annotation via a human-augmenting AI-based labeling interface
user-5f8cf7e04c775ec6fa691c92(2021)
摘要
Abstract Biology has become a prime area for the deployment of deep learning and artificial intelligence (AI), enabled largely by the massive datasets that the field can generate. Key to most AI tasks is the availability of a sufficiently large, labeled dataset with which to train AI models. In the context of microscopy, it is easy to generate image datasets containing millions of cells and structures. However, it is challenging to obtain large-scale high-quality annotations for AI models. Here we present HALI (Human-Augmenting Labeling Interface), a human-in-the-loop AI-based data labeling tool which begins un-initialized and learns annotations from a human, in real-time. Using a multi-part AI composed of three deep learning models, HALI learns from just a few examples and immediately increases both the efficiency of the annotator, and the quality of the annotations. Using a highly repetitive use-case --- annotating cell types --- and running experiments with seven pathologists --- experts at microscopic analysis of biological specimens --- we demonstrate an average human-efficiency improvement of 5.15x and an average data-quality boost of 4.34%, measured across four use-cases and two tissue stain types.
更多查看译文
AI 理解论文
溯源树
样例
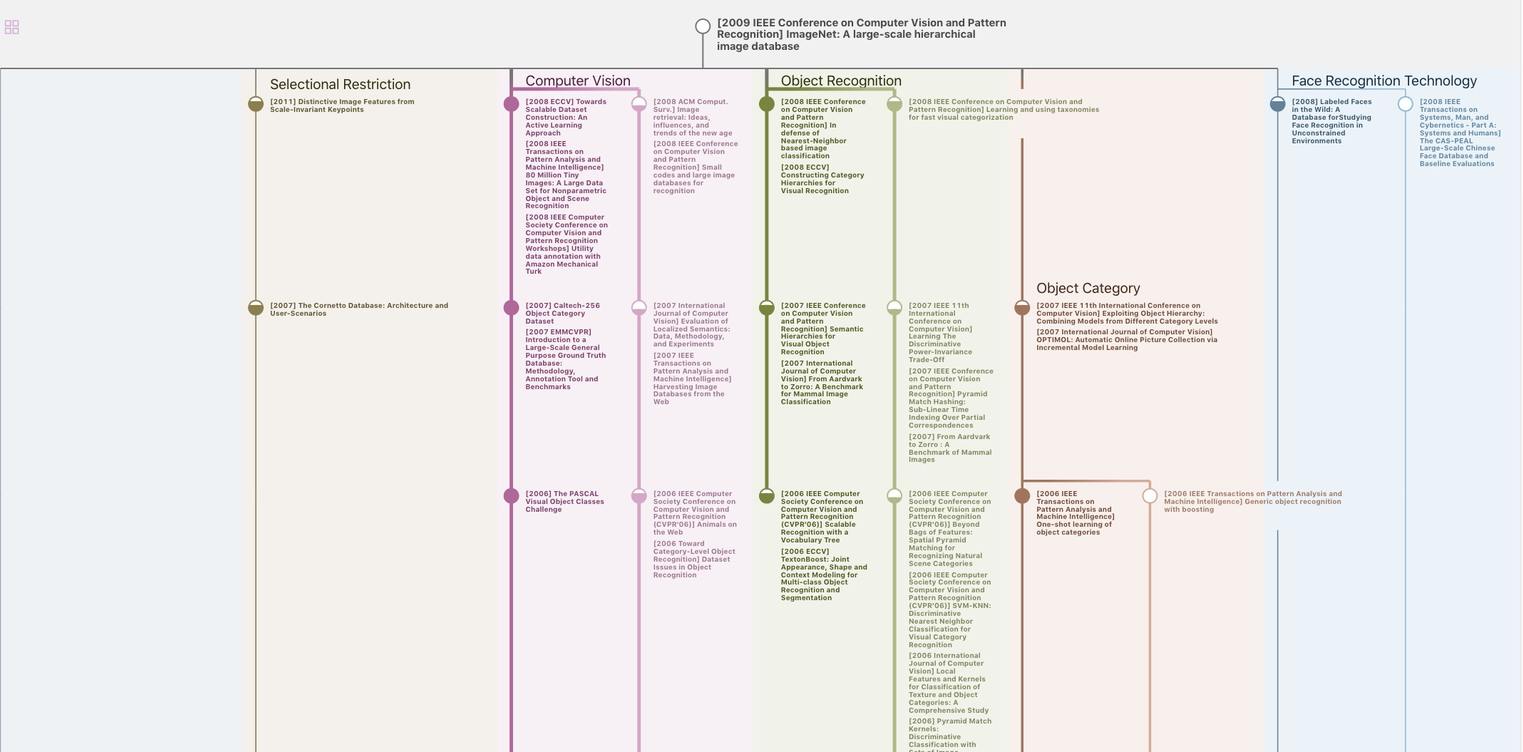
生成溯源树,研究论文发展脉络
Chat Paper
正在生成论文摘要