Coverage as a Principle for Discovering Transferable Behavior in Reinforcement Learning
CoRR(2021)
摘要
Designing agents that acquire knowledge autonomously and use it to solve new tasks efficiently is an important challenge in reinforcement learning, and unsupervised learning provides a useful paradigm for autonomous acquisition of task-agnostic knowledge. In supervised settings, representations discovered through unsupervised pre-training offer important benefits when transferred to downstream tasks. Given the nature of the reinforcement learning problem, we argue that representation alone is not enough for efficient transfer in challenging domains and explore how to transfer knowledge through behavior. The behavior of pre-trained policies may be used for solving the task at hand (exploitation), as well as for collecting useful data to solve the problem (exploration). We argue that policies pre-trained to maximize coverage will produce behavior that is useful for both strategies. When using these policies for both exploitation and exploration, our agents discover better solutions. The largest gains are generally observed in domains requiring structured exploration, including settings where the behavior of the pre-trained policies is misaligned with the downstream task.
更多查看译文
关键词
reinforcement,transferable behavior,learning,coverage
AI 理解论文
溯源树
样例
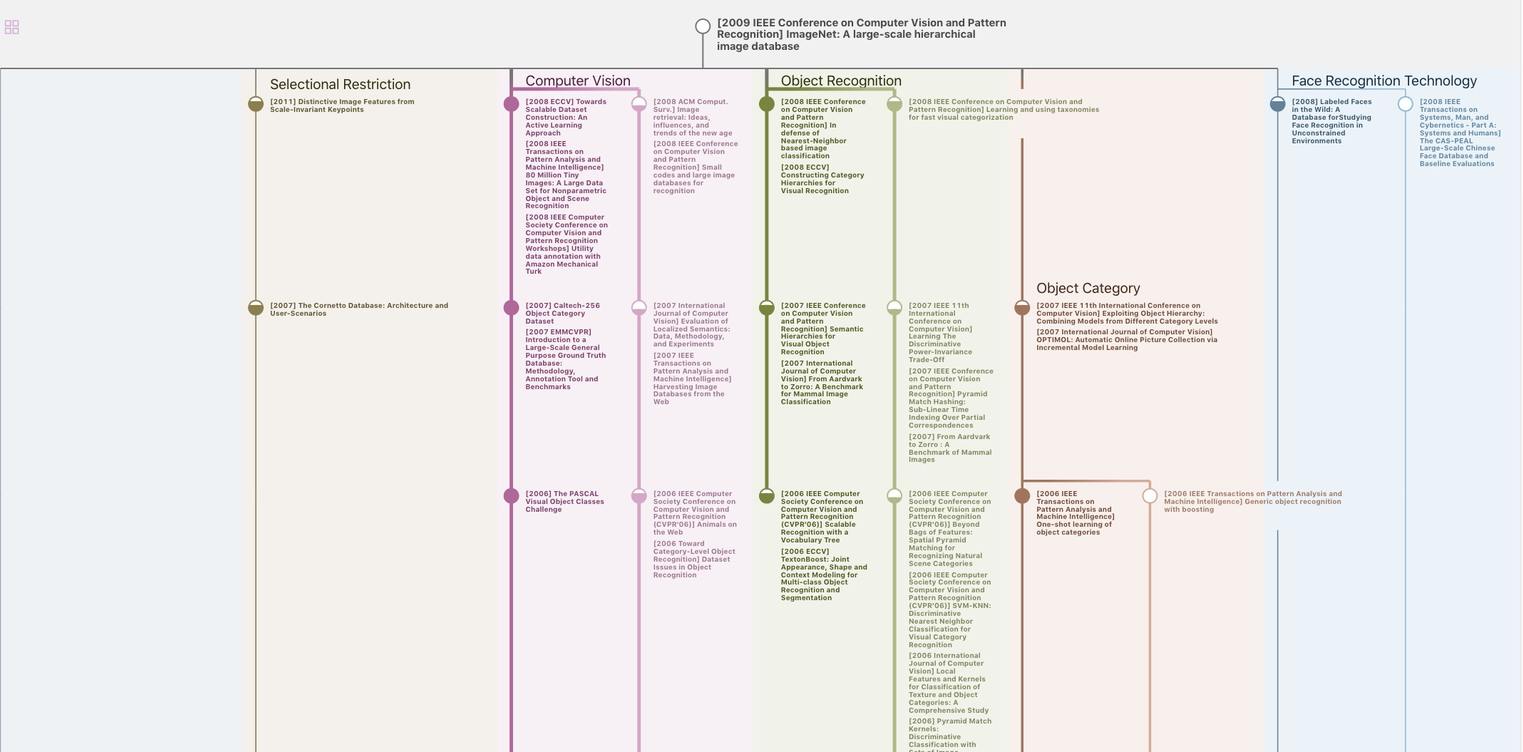
生成溯源树,研究论文发展脉络
Chat Paper
正在生成论文摘要