Are NLP Models really able to Solve Simple Math Word Problems?
NAACL-HLT(2021)
摘要
The problem of designing NLP solvers for math word problems (MWP) has seen sustained research activity and steady gains in the test accuracy. Since existing solvers achieve high performance on the benchmark datasets for elementary level MWPs containing one-unknown arithmetic word problems, such problems are often considered "solved" with the bulk of research attention moving to more complex MWPs. In this paper, we restrict our attention to English MWPs taught in grades four and lower. We provide strong evidence that the existing MWP solvers rely on shallow heuristics to achieve high performance on the benchmark datasets. To this end, we show that MWP solvers that do not have access to the question asked in the MWP can still solve a large fraction of MWPs. Similarly, models that treat MWPs as bag-of-words can also achieve surprisingly high accuracy. Further, we introduce a challenge dataset, SVAMP, created by applying carefully chosen variations over examples sampled from existing datasets. The best accuracy achieved by state-of-the-art models is substantially lower on SVAMP, thus showing that much remains to be done even for the simplest of the MWPs.
更多查看译文
关键词
nlp models,simple math word problems
AI 理解论文
溯源树
样例
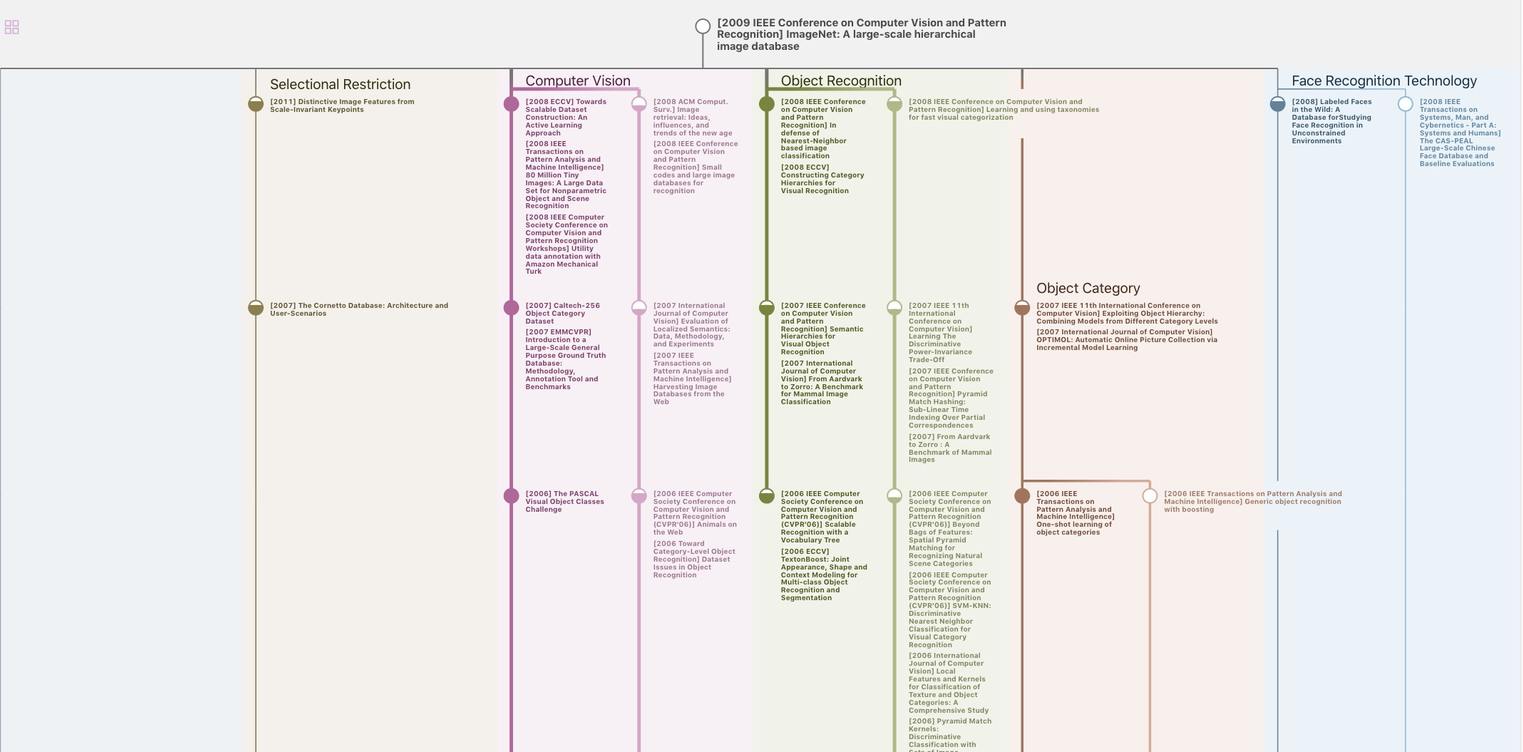
生成溯源树,研究论文发展脉络
Chat Paper
正在生成论文摘要