Improving Translation Robustness with Visual Cues and Error Correction
arxiv(2021)
摘要
Neural Machine Translation models are brittle to input noise. Current robustness techniques mostly adapt models to existing noisy texts, but these models generally fail when faced with unseen noise and their performance degrades on clean texts. In this paper, we introduce the idea of visual context to improve translation robustness against noisy texts. In addition, we propose a novel error correction training regime by treating error correction as an auxiliary task to further improve robustness. Experiments on English-French and English-German translation show that both multimodality and error correction training are beneficial for model robustness to known and new types of errors, while keeping the quality on clean texts.
更多查看译文
关键词
translation robustness,error correction,visual cues
AI 理解论文
溯源树
样例
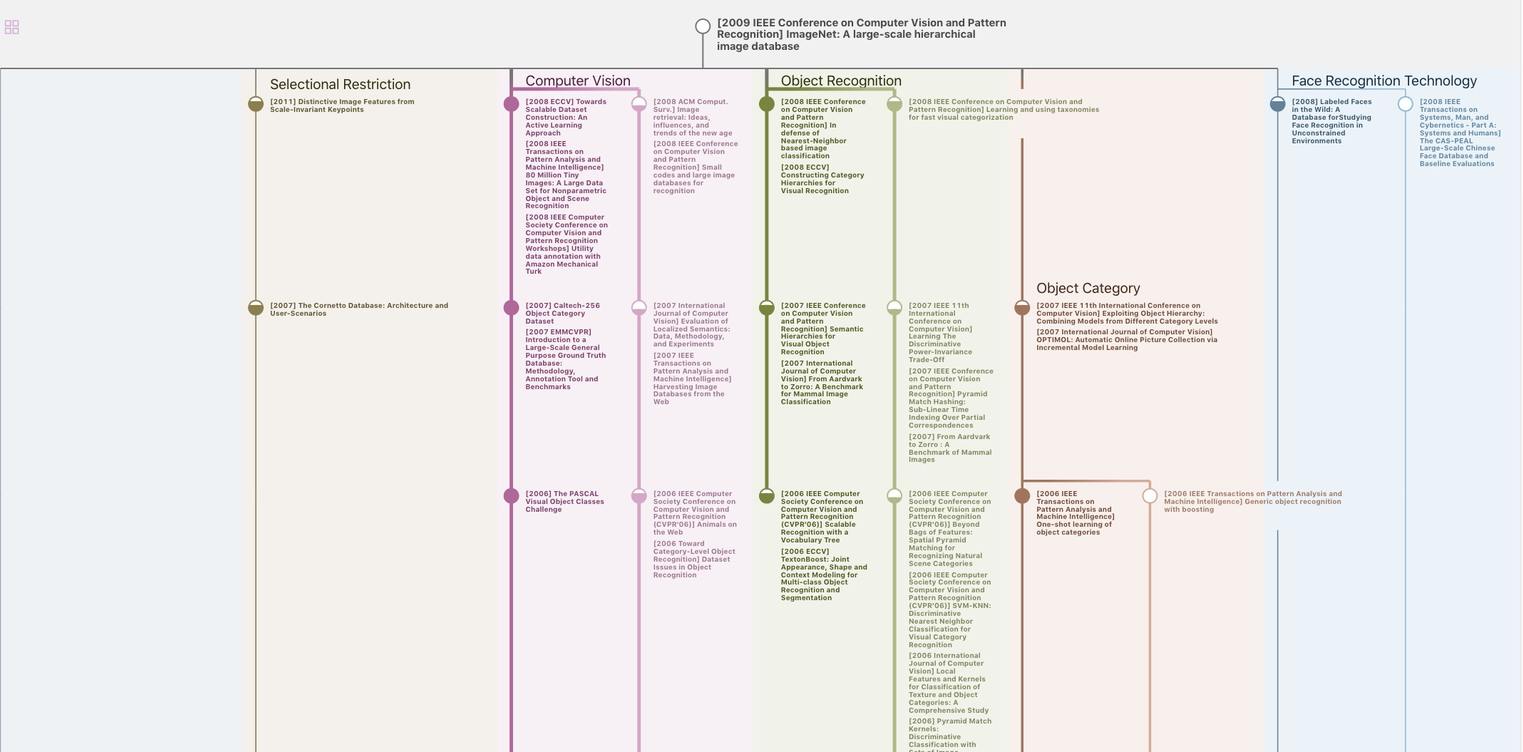
生成溯源树,研究论文发展脉络
Chat Paper
正在生成论文摘要