KiloNeRF - Speeding up Neural Radiance Fields with Thousands of Tiny MLPs.
ICCV(2021)
摘要
NeRF synthesizes novel views of a scene with unprecedented quality by fitting a neural radiance field to RGB images. However, NeRF requires querying a deep Multi-Layer Perceptron (MLP) millions of times, leading to slow rendering times, even on modern GPUs. In this paper, we demonstrate that significant speed-ups are possible by utilizing thousands of tiny MLPs instead of one single large MLP. In our setting, each individual MLP only needs to represent parts of the scene, thus smaller and faster-to-evaluate MLPs can be used. By combining this divide-and-conquer strategy with further optimizations, rendering is accelerated by two orders of magnitude compared to the original NeRF model without incurring high storage costs. Further, using teacher-student distillation for training, we show that this speed-up can be achieved without sacrificing visual quality.
更多查看译文
关键词
Image and video synthesis,Efficient training and inference methods
AI 理解论文
溯源树
样例
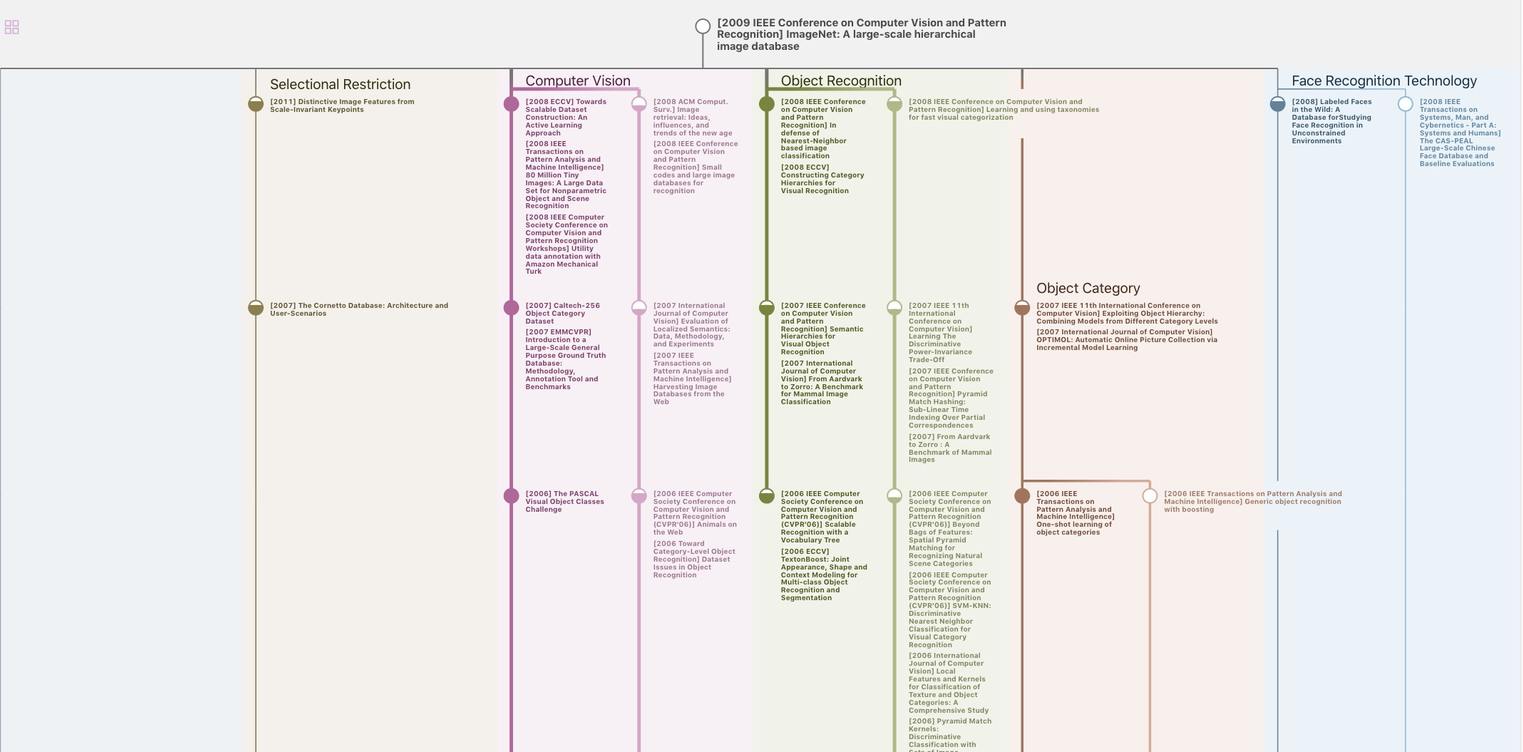
生成溯源树,研究论文发展脉络
Chat Paper
正在生成论文摘要