cGAIL: C onditional G enerative A dversarial I mitation L earning—An Application in Taxi Drivers’ Strategy Learning
IEEE Transactions on Big Data(2022)
摘要
Smart passenger-seeking strategies employed by taxi drivers contribute not only to drivers’ incomes, but also higher quality of service passengers received. Therefore, understanding taxi drivers’ behaviors and learning the good passenger-seeking strategies are crucial to boost taxi drivers’ well-being and public transportation quality of service. However, we observe that drivers’ preferences of choosing which area to find the next passenger are diverse and dynamic across locations and drivers. It is hard to learn the location-dependent preferences given the partial data (i.e., an individual driver’s trajectory may not cover all locations). In this article, we make the first attempt to develop conditional generative adversarial imitation learning (cGAIL) model, as a unifying collective inverse reinforcement learning framework that learns the driver’s decision-making preferences and policies by transferring knowledge across taxi driver agents and across locations. Our evaluation results on three months of taxi GPS trajectory data in Shenzhen, China, demonstrate that the driver’s preferences and policies learned from cGAIL are on average 36.2 percent more accurate than those learned from other state-of-the-art baseline approaches.
更多查看译文
关键词
Urban computing,inverse reinforcement learning,generative adversarial imitation learning
AI 理解论文
溯源树
样例
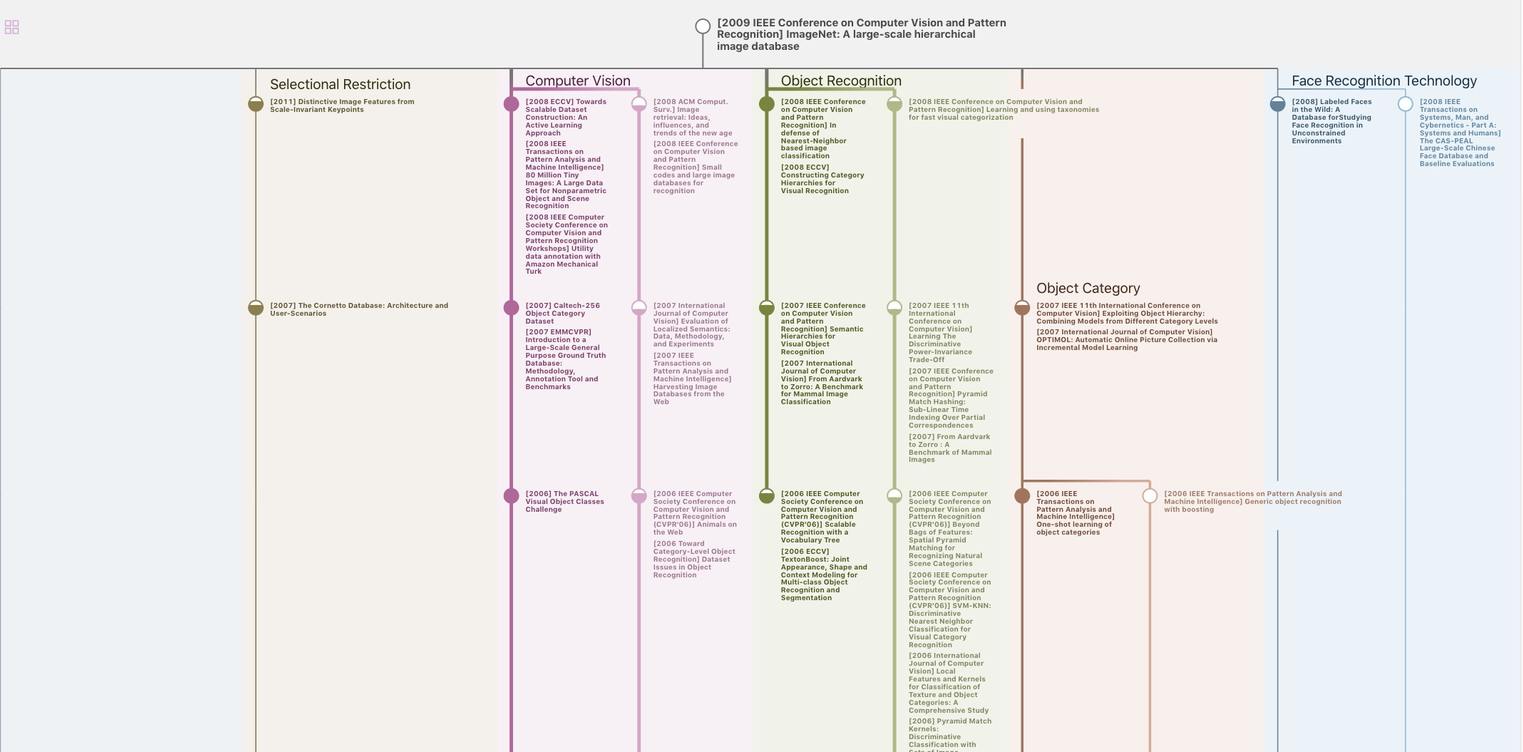
生成溯源树,研究论文发展脉络
Chat Paper
正在生成论文摘要