Aligned Contrastive Predictive Coding.
Interspeech(2021)
摘要
We investigate the possibility of forcing a self-supervised model trained using a contrastive predictive loss to extract slowly varying latent representations. Rather than producing individual predictions for each of the future representations, the model emits a sequence of predictions shorter than that of the upcoming representations to which they will be aligned. In this way, the prediction network solves a simpler task of predicting the next symbols, but not their exact timing, while the encoding network is trained to produce piece-wise constant latent codes. We evaluate the model on a speech coding task and demonstrate that the proposed Aligned Contrastive Predictive Coding (ACPC) leads to higher linear phone prediction accuracy and lower ABX error rates, while being slightly faster to train due to the reduced number of prediction heads.
更多查看译文
关键词
self-supervised learning,contrast predictive coding,dynamic time warping,zerospeech
AI 理解论文
溯源树
样例
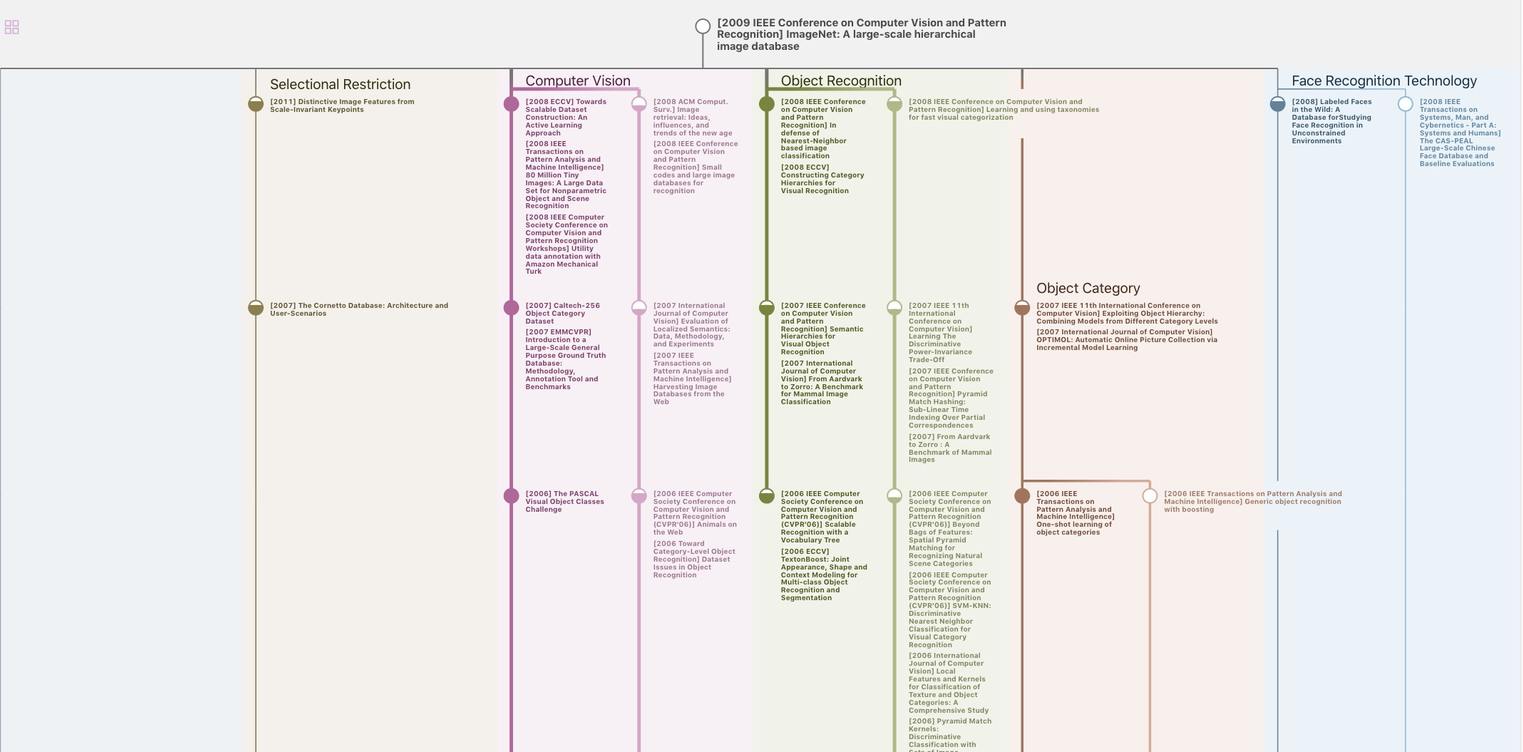
生成溯源树,研究论文发展脉络
Chat Paper
正在生成论文摘要