On Linear Interpolation in the Latent Space of Deep Generative Models
international conference on learning representations(2021)
摘要
The underlying geometrical structure of the latent space in deep generative models is in most cases not Euclidean, which may lead to biases when comparing interpolation capabilities of two models. Smoothness and plausibility of linear interpolations in latent space are associated with the quality of the underlying generative model. In this paper, we show that not all such interpolations are comparable as they can deviate arbitrarily from the shortest interpolation curve given by the geodesic. This deviation is revealed by computing curve lengths with the pull-back metric of the generative model, finding shorter curves than the straight line between endpoints, and measuring a non-zero relative length improvement on this straight line. This leads to a strategy to compare linear interpolations across two generative models. We also show the effect and importance of choosing an appropriate output space for computing shorter curves. For this computation we derive an extension of the pull-back metric.
更多查看译文
关键词
linear interpolation,latent space,models
AI 理解论文
溯源树
样例
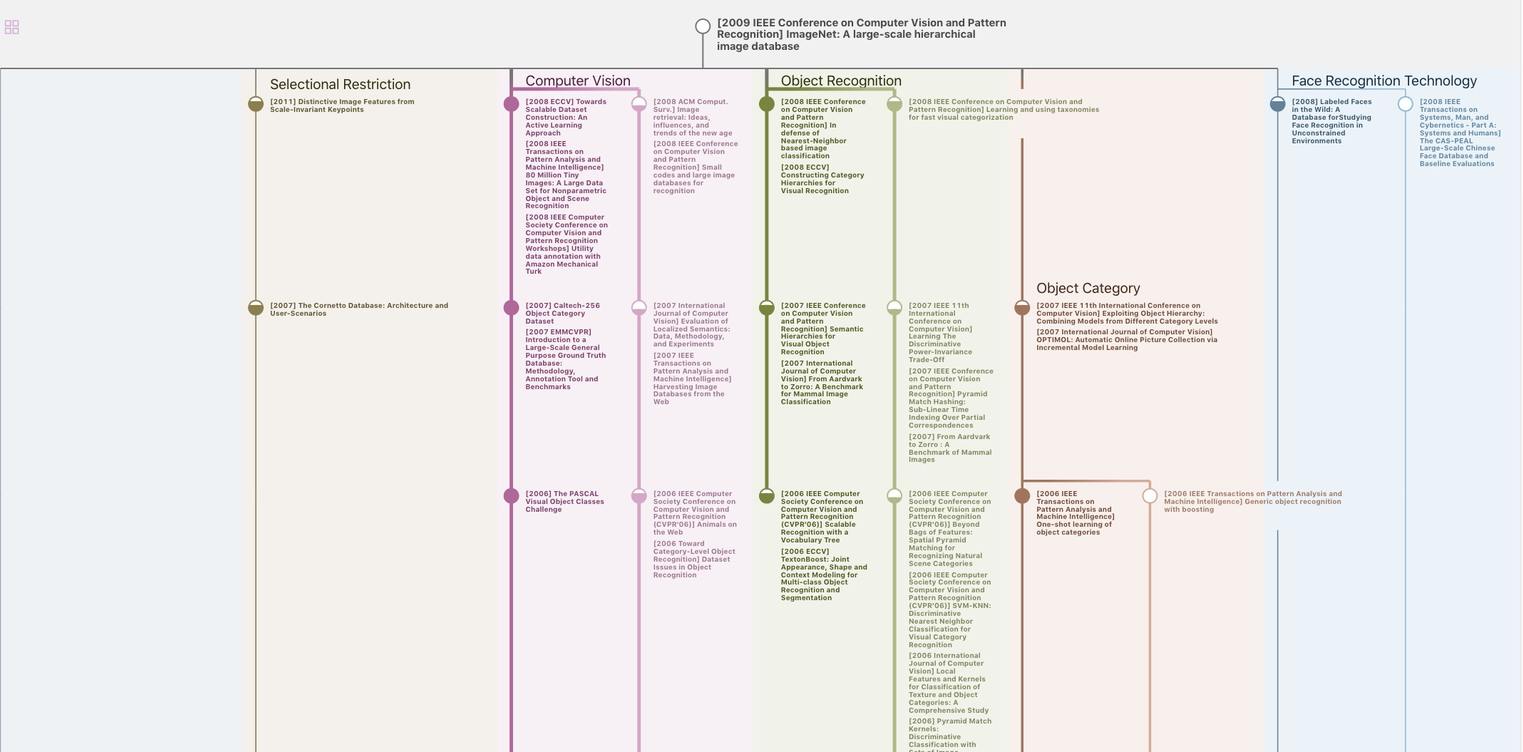
生成溯源树,研究论文发展脉络
Chat Paper
正在生成论文摘要