Randomized Exploration For Reinforcement Learning With General Value Function Approximation
INTERNATIONAL CONFERENCE ON MACHINE LEARNING, VOL 139(2021)
摘要
We propose a model-free reinforcement learning algorithm inspired by the popular randomized least squares value iteration (RLSVI) algorithm as well as the optimism principle. Unlike existing upper-confidence-bound (UCB) based approaches, which are often computationally intractable, our algorithm drives exploration by simply perturbing the training data with judiciously chosen i.i.d. scalar noises. To attain optimistic value function estimation without resorting to a UCB-style bonus, we introduce an optimistic reward sampling procedure. When the value functions can be represented by a function class F, our algorithm achieves a worst-case regret bound of (O) over tilde (poly(d(E)H)root T) where T is the time elapsed, d(E) is the planning horizon and d E is the eluder dimension of In the linear setting, our algorithm reduces to LSVI-PHE, a variant of RLSVI, that enjoys an (O) over tilde root d(3)H(3)T) regret. We complement the theory with an empirical evaluation across known difficult exploration tasks.
更多查看译文
AI 理解论文
溯源树
样例
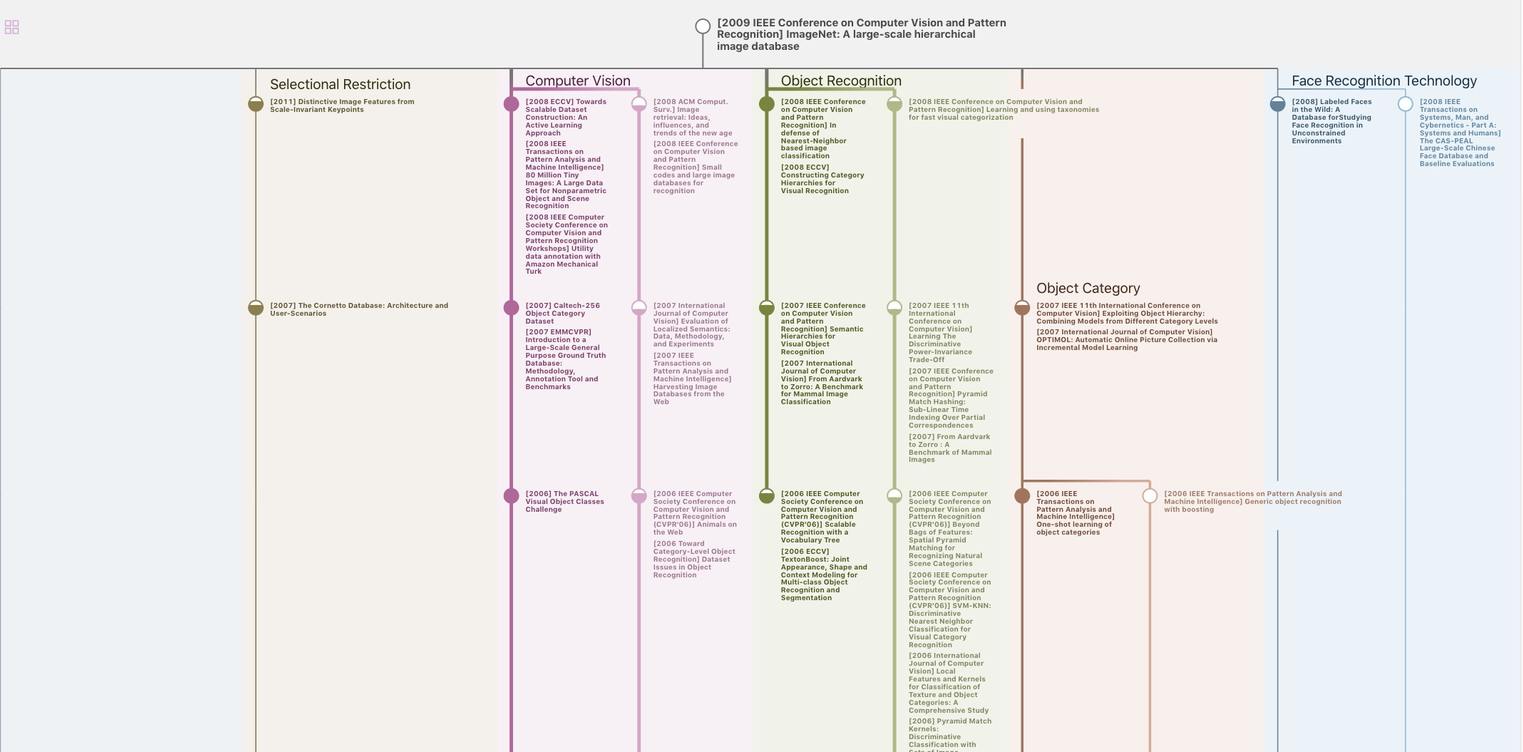
生成溯源树,研究论文发展脉络
Chat Paper
正在生成论文摘要