Image2Point: 3D Point-Cloud Understanding with Pretrained 2D ConvNets
arxiv(2021)
摘要
3D point-clouds and 2D images are different visual representations of the physical world. While human vision can understand both representations, computer vision models designed for 2D image and 3D point-cloud understanding are quite different. Our paper investigates the potential for transferability between these two representations by empirically investigating whether this approach works, what factors affect the transfer performance, and how to make it work even better. We discovered that we can indeed use the same neural net model architectures to understand both images and point-clouds. Moreover, we can transfer pretrained weights from image models to point-cloud models with minimal effort. Specifically, based on a 2D ConvNet pretrained on an image dataset, we can transfer the image model to a point-cloud model by \textit{inflating} 2D convolutional filters to 3D then finetuning its input, output, and optionally normalization layers. The transferred model can achieve competitive performance on 3D point-cloud classification, indoor and driving scene segmentation, even beating a wide range of point-cloud models that adopt task-specific architectures and use a variety of tricks.
更多查看译文
关键词
pretrained 2d convnets,3d,point-cloud
AI 理解论文
溯源树
样例
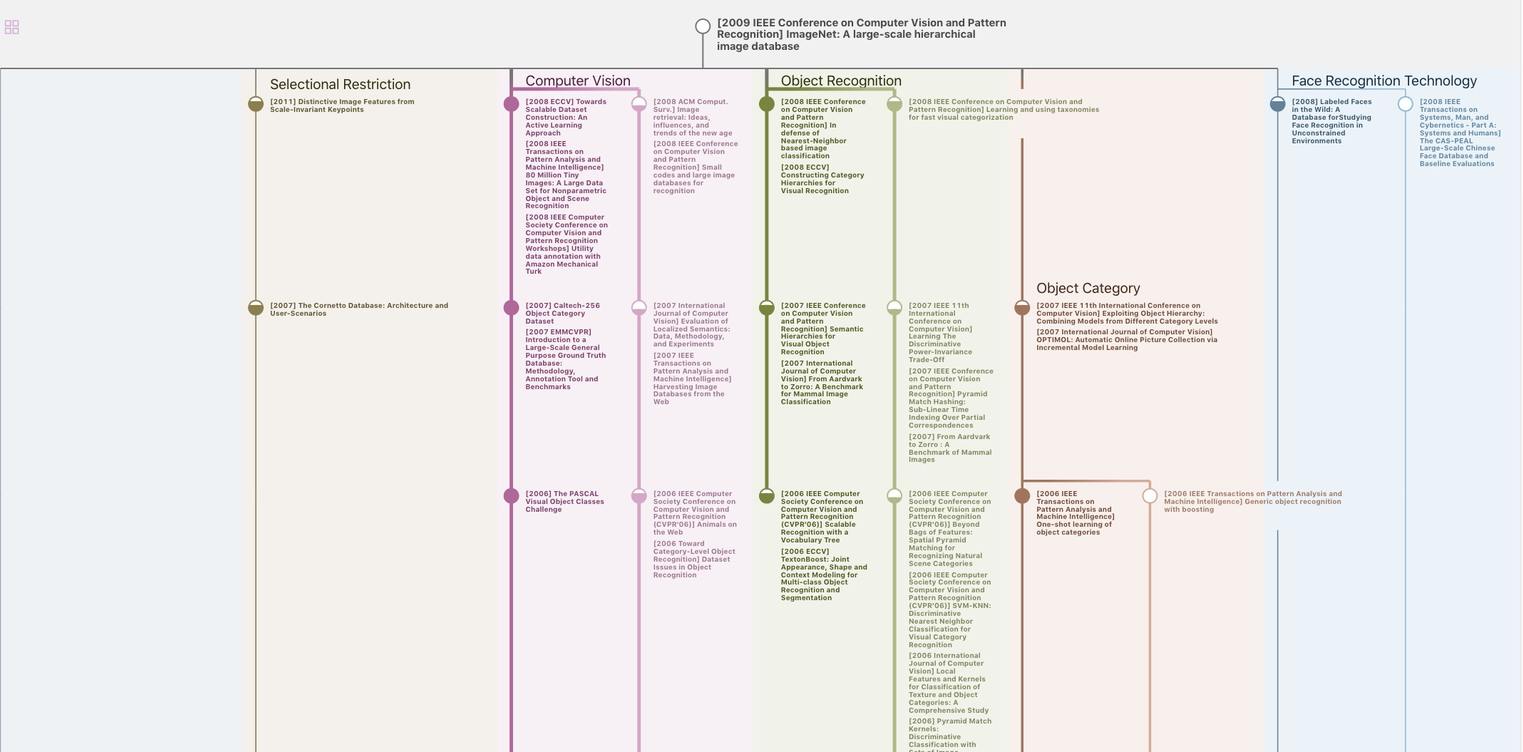
生成溯源树,研究论文发展脉络
Chat Paper
正在生成论文摘要