Learning Time-Invariant Reward Functions through Model-Based Inverse Reinforcement Learning
arxiv(2021)
摘要
Inverse reinforcement learning is a paradigm motivated by the goal of learning general reward functions from demonstrated behaviours. Yet the notion of generality for learnt costs is often evaluated in terms of robustness to various spatial perturbations only, assuming deployment at fixed speeds of execution. However, this is impractical in the context of robotics and building time-invariant solutions is of crucial importance. In this work, we propose a formulation that allows us to 1) vary the length of execution by learning time-invariant costs, and 2) relax the temporal alignment requirements for learning from demonstration. We apply our method to two different types of cost formulations and evaluate their performance in the context of learning reward functions for simulated placement and peg in hole tasks. Our results show that our approach enables learning temporally invariant rewards from misaligned demonstration that can also generalise spatially to out of distribution tasks.
更多查看译文
关键词
reward,learning,inverse,time-invariant,model-based
AI 理解论文
溯源树
样例
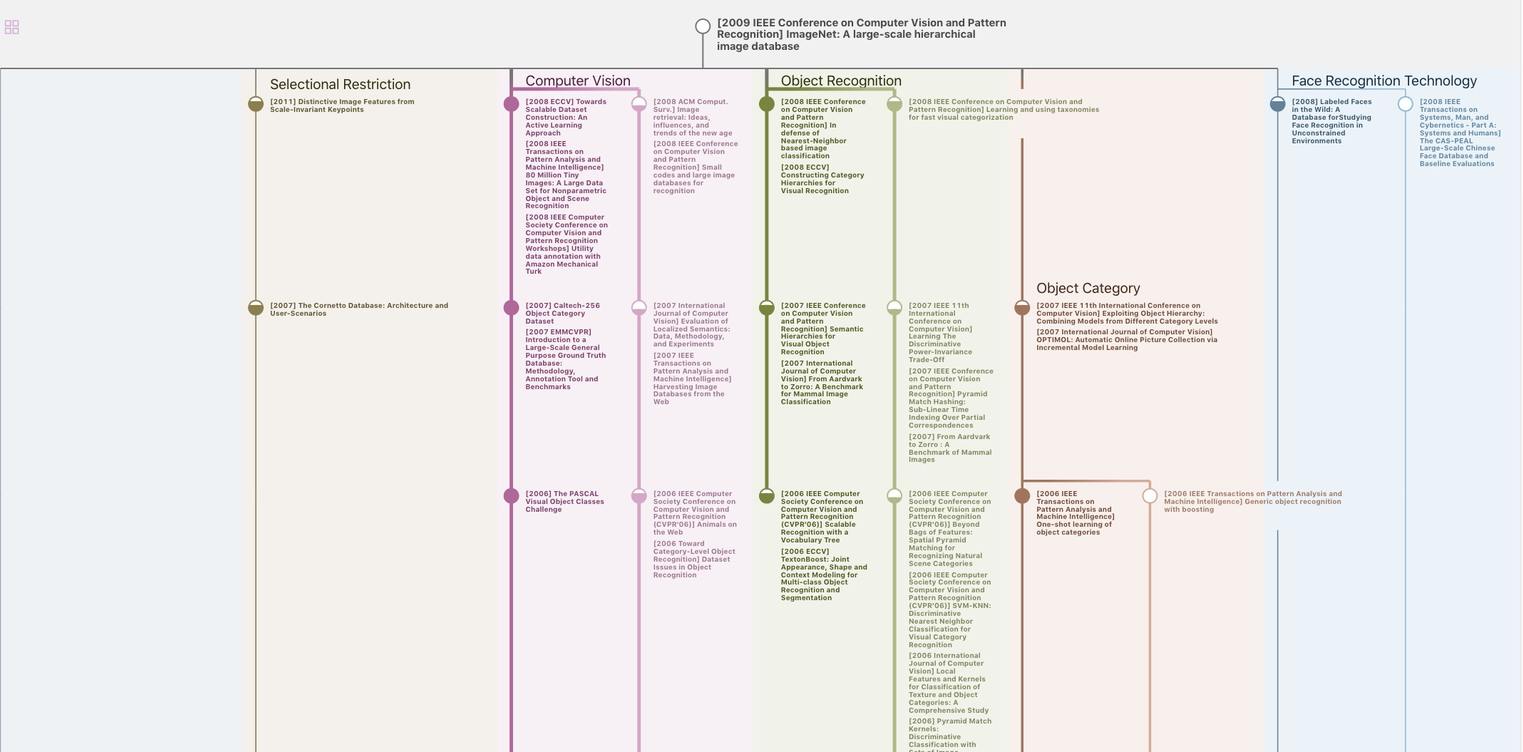
生成溯源树,研究论文发展脉络
Chat Paper
正在生成论文摘要