Pixel Difference Networks for Efficient Edge Detection
ICCV(2021)
摘要
Recently, deep Convolutional Neural Networks (CNNs) can achieve human-level performance in edge detection with the rich and abstract edge representation capacities. However, the high performance of CNN based edge detection is achieved with a large pretrained CNN backbone, which is memory and energy consuming. In addition, it is surprising that the previous wisdom from the traditional edge detectors, such as Canny, Sobel, and LBP are rarely investigated in the rapid-developing deep learning era. To address these issues, we propose a simple, lightweight yet effective architecture named Pixel Difference Network (PiDiNet) for efficient edge detection. PiDiNet adopts novel pixel difference convolutions that integrate the traditional edge detection operators into the popular convolutional operations in modern CNNs for enhanced performance on the task, which enjoys the best of both worlds. Extensive experiments on BSDS500, NYUD, and Multicue are provided to demonstrate its effectiveness, and its high training and inference efficiency. Surprisingly, when training from scratch with only the BSDS500 and VOC datasets, PiDiNet can surpass the recorded result of human perception (0.807 vs. 0.803 in ODS F-measure) on the BSDS500 dataset with 100 FPS and less than 1M parameters. A faster version of PiDiNet with less than 0.1M parameters can still achieve comparable performance among state of the arts with 200 FPS. Results on the NYUD and Multicue datasets show similar observations. The codes are available at https://github.com/zhuoinoulu/pidinet.
更多查看译文
关键词
Efficient training and inference methods,Representation learning,Vision applications and systems
AI 理解论文
溯源树
样例
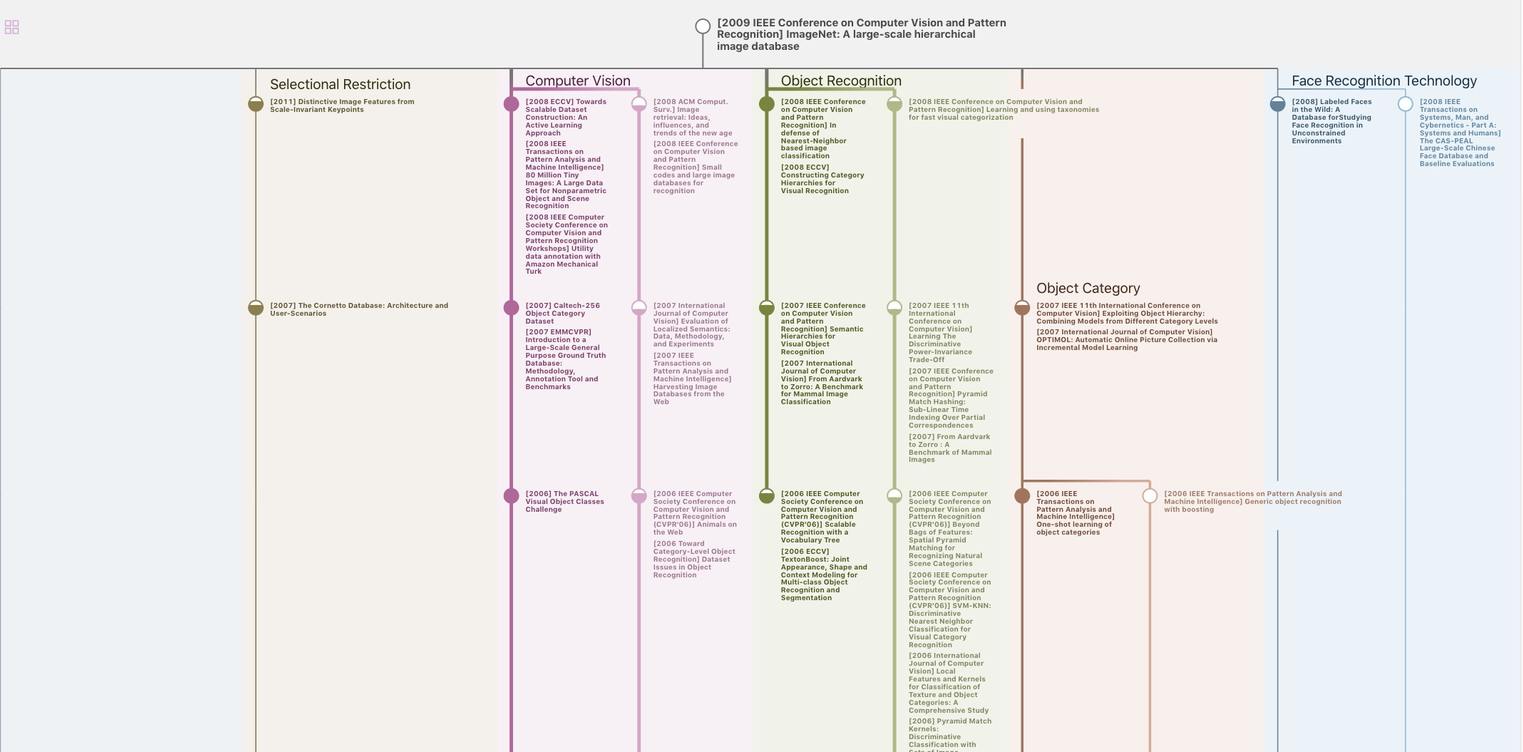
生成溯源树,研究论文发展脉络
Chat Paper
正在生成论文摘要