Learning Melanocytic Proliferation Segmentation in Histopathology Images from Imperfect Annotations
2021 IEEE/CVF CONFERENCE ON COMPUTER VISION AND PATTERN RECOGITION WORKSHOPS (CVPRW 2021)(2021)
摘要
Melanoma is the third most common type of skin cancer and is responsible for the most skin cancer deaths. A diagnosis of melanoma is made by the visual interpretation of tissue sections by a pathologist, a challenging task given the complexity and breadth of melanocytic lesions and the subjective nature of biopsy interpretation. We leverage advances in computer vision to aid melanoma diagnosis by segmenting potential regions of lesions on digital images of whole slide skin biopsies. In this study, we demonstrate a Mask-R-CNN-based segmentation framework for such a purpose. To alleviate the cost of data annotation, we leverage a sparse annotation pipeline. Our model can be trained on sparse and noisy labels and achieves state-of-the-art performance in identifying melanocytic proliferations, producing a segmentation with Dice score 0.719, mIOU 0.740 and overall pixel accuracy 0.927.
更多查看译文
关键词
Mask-R-CNN-based segmentation framework,data annotation,sparse annotation pipeline,noisy labels,achieves state-of-the-art performance,melanocytic proliferations,melanocytic proliferation segmentation,histopathology images,imperfect annotations,skin cancer deaths,visual interpretation,tissue sections,pathologist,melanocytic lesions,subjective nature,biopsy interpretation,leverage advances,computer vision,melanoma diagnosis,digital images,slide skin biopsies
AI 理解论文
溯源树
样例
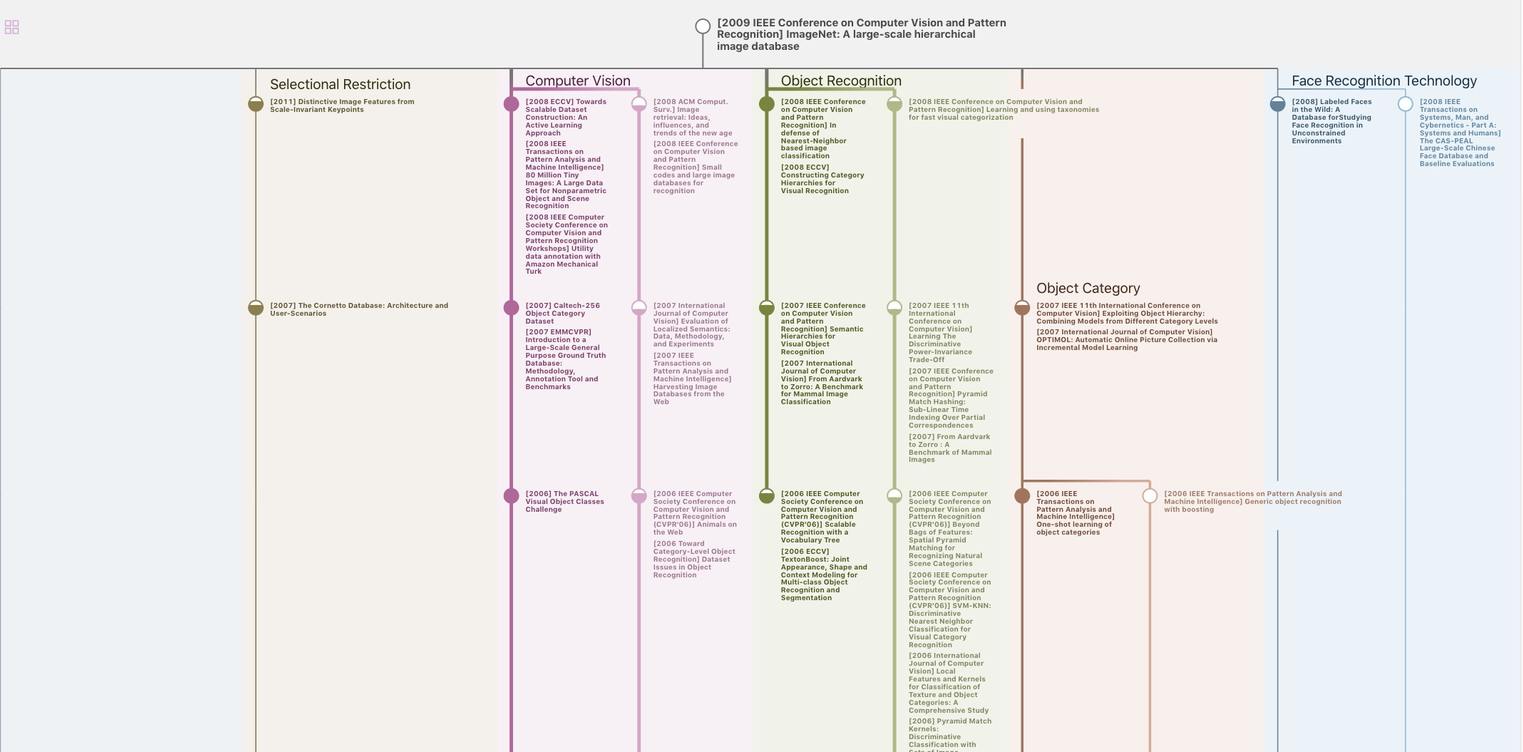
生成溯源树,研究论文发展脉络
Chat Paper
正在生成论文摘要