Cross-Domain Imitation Learning via Optimal Transport
International Conference on Learning Representations (ICLR)(2022)
摘要
Cross-domain imitation learning studies how to leverage expert demonstrations of one agent to train an imitation agent with a different embodiment or morphology. Comparing trajectories and stationary distributions between the expert and imitation agents is challenging because they live on different systems that may not even have the same dimensionality. We propose Gromov-Wasserstein Imitation Learning (GWIL), a method for cross-domain imitation that uses the Gromov-Wasserstein distance to align and compare states between the different spaces of the agents. Our theory formally characterizes the scenarios where GWIL preserves optimality, revealing its possibilities and limitations. We demonstrate the effectiveness of GWIL in non-trivial continuous control domains ranging from simple rigid transformation of the expert domain to arbitrary transformation of the state-action space.
更多查看译文
关键词
optimal transportation,imitation learning,cross-domain imitation learning,gromov-Wasserstein
AI 理解论文
溯源树
样例
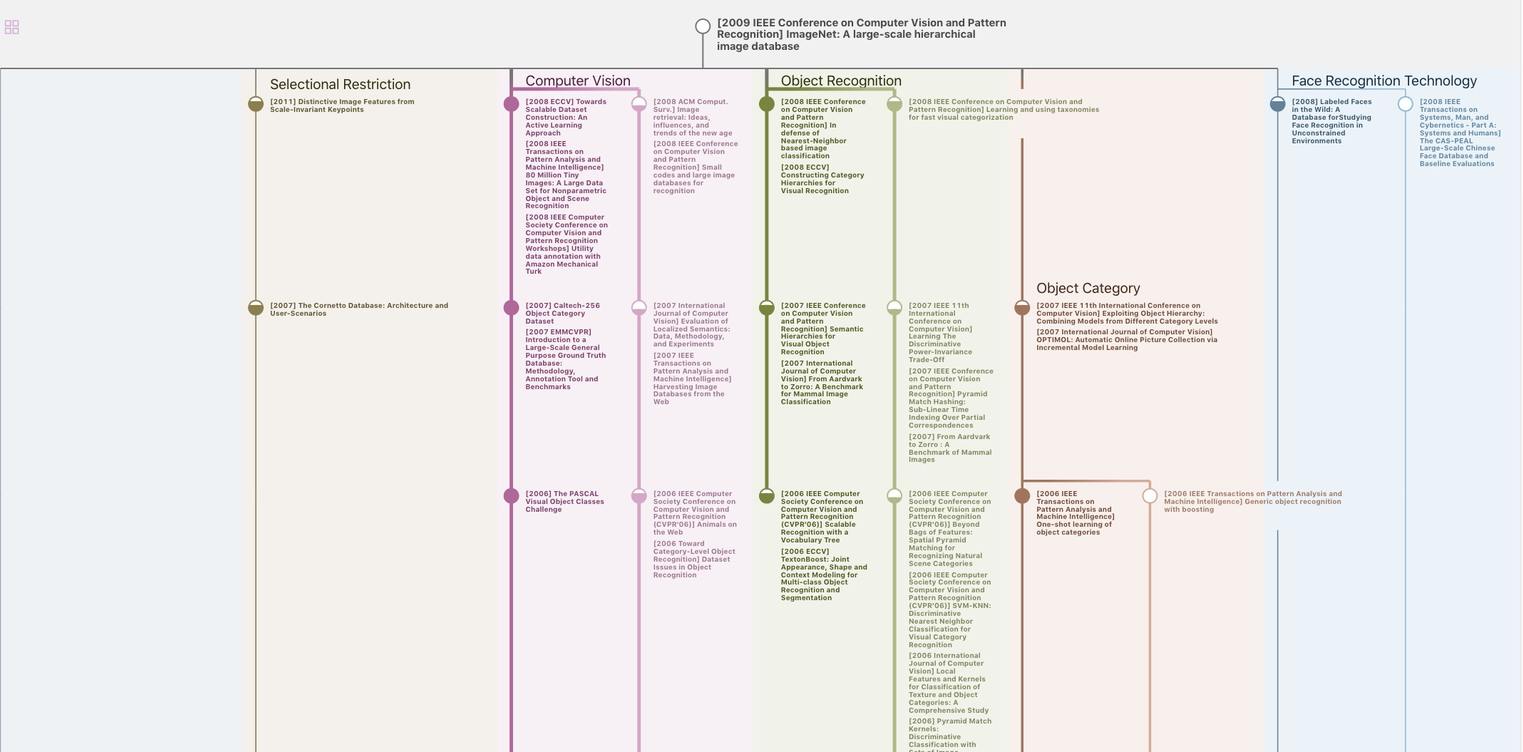
生成溯源树,研究论文发展脉络
Chat Paper
正在生成论文摘要