Hindsight: Posterior-guided training of retrievers for improved open-ended generation
International Conference on Learning Representations (ICLR)(2022)
摘要
Many text generation systems benefit from using a retriever to retrieve passages from a textual knowledge corpus (e.g., Wikipedia) which are then provided as additional context to the generator. For open-ended generation tasks (like generating informative utterances in conversations) many varied passages may be equally relevant and we find that existing methods that jointly train the retriever and generator underperform: the retriever may not find relevant passages even amongst the top-10 and hence the generator may not learn a preference to ground its generated output in them. We propose using an additional guide retriever that is allowed to use the target output and "in hindsight" retrieve relevant passages during training. We model the guide retriever after the posterior distribution Q of passages given the input and the target output and train it jointly with the standard retriever and the generator by maximizing the evidence lower bound (ELBo) in expectation over Q. For informative conversations from the Wizard of Wikipedia dataset, with posterior-guided training, the retriever finds passages with higher relevance in the top-10 (23% relative improvement), the generator's responses are more grounded in the retrieved passage (19% relative improvement) and the end-to-end system produces better overall output (6.4% relative improvement).
更多查看译文
关键词
retrieval,generation,retrieval-augmented generation,open-ended generation,informative conversations,free-form QA,posterior distribution,ELBo
AI 理解论文
溯源树
样例
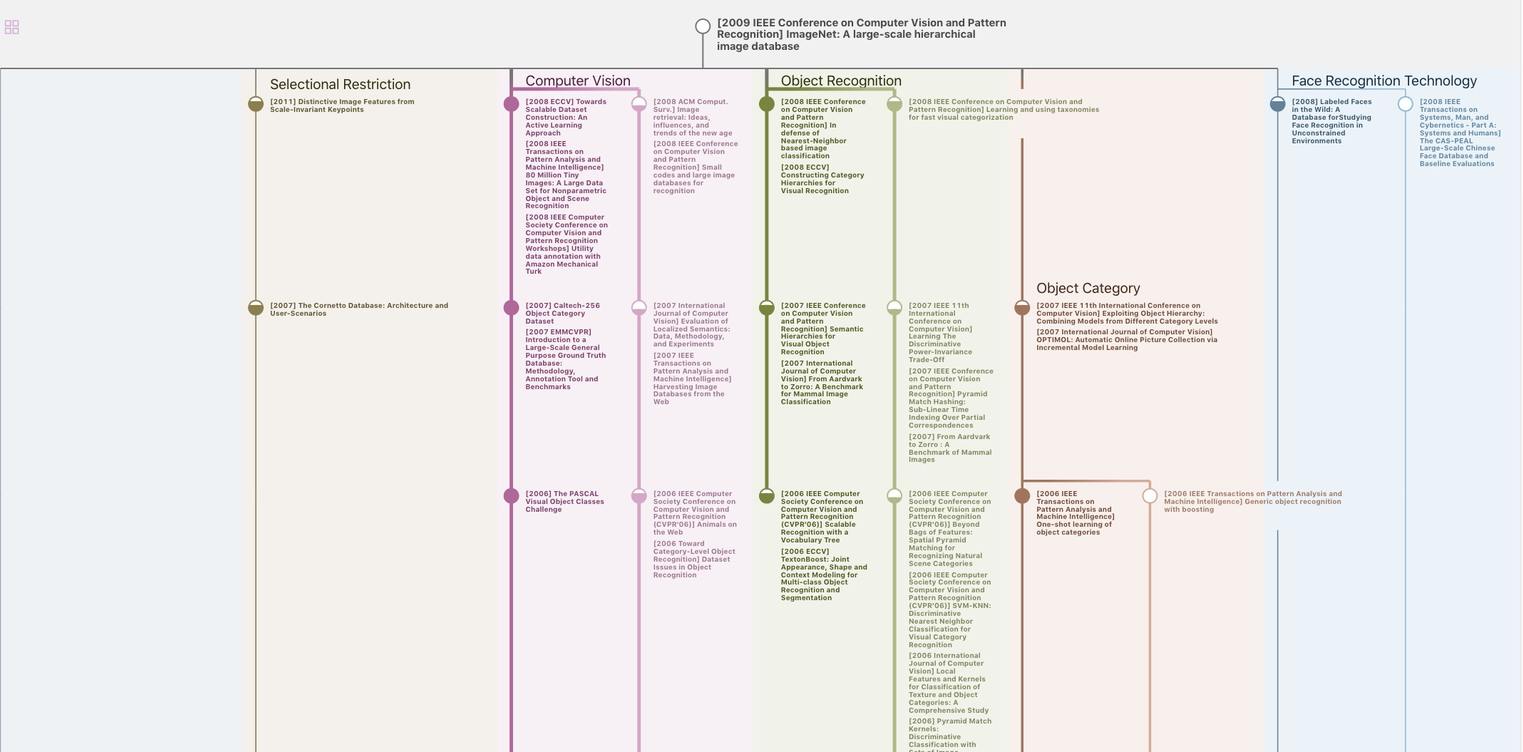
生成溯源树,研究论文发展脉络
Chat Paper
正在生成论文摘要